What is Big Data Finance?
Big Data is both structured and unstructured volumetric datasets. Big Data Finance is used in banking services, business, and in general in the financial sector. That is, this kind of data is related to this very field. Why are such large amounts of data good? Their further processing and analysis facilitate the work of various companies that provide their services to a specific target audience. Many businessmen can warn their developments, companies, and products against fraud. Also, such data is often used by investors to analyze the situation in the market.
All in all, according to statistics, Big Data is widely used today in various spheres (mostly – in the financial sector) and by 2025 there will have been 79 zettabytes of them. Big Data and analytics software are mostly provided by globally known companies like Oracle and Microsoft. However, if we speak about the financial sector exactly, InData Labs can be highlighted as one of the most prosperous companies with its Big Data analytics solutions. The brand guarantees to do a lot for your future business and clients.
How can Big Data finance analysis help people?
The first thing that is undoubtedly worth noting is the vastness of the financial sector and the need for a large amount of data in it. Their processing helps both private entrepreneurs and banking institutions and even investors. Thus, Big Data provides the following benefits:
- Stock market analysis. Researching the state of the stock market is very useful for investors. This analysis involves the so-called machine learning – computer algorithms aimed at finding various data patterns. Based on this information, it is possible to make the most reliable stock price predictions for traders.
- Improved predictive modeling. As was mentioned, Big Data finance analysis helps to model the situation in the market or business. Such a model will contribute to the improvement of profits or investments of a person.
- Analysis of clients’ preferences. This is a significant feature of Big Data because due to such research, companies and their owners may create a convenient atmosphere for a client easily. Moreover, it will be much more simple to define a target audience.
- Fraud detection. By analyzing people’s transactions and searching for data patterns, machine learning allows businesses to detect and reduce fraud. It contributes to reducing the risks of an entrepreneur.
Challenges of Big Data in Finance
Besides the fruitful work of Big Data in the financial sector, it still faces different challenges that we are going to get you familiar with.
Security of Clients’ Data
The privacy of customer data should always come first, regardless of the type of company. However, not all corporations can ensure the complete security of customers’ personal information while collecting and processing the entire flow of data at the same time. The same point is dedicated to the further storage of collected data.
The Unwillingness of the Company to Introduce Innovations
Some companies, in particular banking institutions, don’t have sufficiently advanced working systems for machine learning to work properly. For this reason, there may be failures and the use of Big Data will not bring much benefit.
Following Regulatory Requirements
Financial institutions are required to adhere to the strict Basel Committee on Banking Supervision (BCBS) regulations known as the Fundamental Review of the Trading Book (FRTB), which regulate access to crucial data and mandate faster reporting. Unfortunately, not all organizations can do it properly.
Final Verdict
Summing up, Big Data plays a significant role in the financial sphere. It influences it in a positive way, which is quite beneficial both for entrepreneurs and their clients. Nevertheless, there are still some challenges that companies face while implementing Big Data. At the same time, the role of such a system can’t be underestimated, Big Data is growing and improving the quality of more and more businesses. That’s why using such volumetric datasets is helpful and even necessary today.
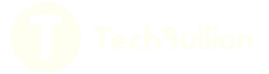