Healthcare is undergoing a technological transformation, with Machine Learning Operations (MLOps) emerging as a crucial framework for integrating artificial intelligence into clinical settings. Shivakrishna Bade explores this evolution, detailing how MLOps enhances predictive analytics, model deployment, and regulatory compliance in medical environments. Bade’s insights shed light on how healthcare institutions are leveraging structured ML workflows to improve patient care outcomes and operational efficiency.
Revolutionizing Model Deployment in Healthcare
The introduction of MLOps into healthcare has significantly streamlined the deployment of machine learning models. Traditional AI implementations often faced prolonged testing phases and inconsistent performance across clinical environments. With MLOps frameworks, healthcare organizations have reported increased efficiency in model validation, leading to faster integration of AI-powered decision support systems. These improvements translate into quicker diagnoses and more effective treatment plans, ensuring that healthcare professionals can rely on AI-driven insights in critical situations.
Strengthening Data Management and Security
Healthcare institutions deal with vast amounts of patient data, requiring robust systems for storage, processing, and privacy management. MLOps frameworks offer sophisticated data pipeline management solutions that enhance accuracy and reduce processing time. Automated validation protocols ensure that medical records, diagnostic imaging, and other patient data are seamlessly integrated into machine learning models without compromising security standards. This has led to significant advancements in maintaining regulatory compliance while fostering innovation in patient data utilization.
Enhancing Predictive Analytics for Better Outcomes
One of the most impactful applications of MLOps in healthcare is predictive analytics. By leveraging machine learning models, healthcare providers can anticipate patient deterioration, predict disease progression, and optimize treatment plans. These capabilities are particularly beneficial in chronic disease management, where early intervention can drastically improve outcomes. Predictive models have demonstrated high accuracy rates in identifying at-risk patients, allowing for timely medical interventions that can reduce hospital readmissions and enhance overall patient well-being.
Improving Diagnostic Accuracy with AI Integration
Machine learning models, when systematically integrated through MLOps, have revolutionized diagnostic accuracy in various medical fields. AI-powered imaging analysis, for instance, has achieved remarkable precision in detecting conditions such as tumors and cardiovascular diseases. Through structured monitoring and iterative model improvements, MLOps ensures that these AI systems maintain high reliability across different patient demographics. This consistency is crucial in making machine-assisted diagnostics a trusted tool in healthcare settings.
Ensuring Regulatory Compliance and Ethical AI Use
One of the significant challenges in deploying AI in healthcare is adhering to stringent regulatory requirements. MLOps frameworks provide a structured approach to compliance, automating documentation, audit processes, and model validation. This not only reduces the time needed for regulatory approvals but also ensures that AI models operate within ethical and legal guidelines. Healthcare institutions with established MLOps frameworks have reported fewer compliance-related setbacks.
The Role of Continuous Monitoring and Model Optimization
MLOps is not just about deploying models; it also emphasizes continuous monitoring and optimization. Healthcare AI models need to be constantly evaluated for performance degradation, biases, and inaccuracies. Automated monitoring tools integrated within MLOps frameworks allow real-time tracking of model efficiency, ensuring that AI-driven solutions remain effective and adaptable to evolving medical needs. This proactive approach minimizes the risk of outdated or erroneous predictions impacting patient care.
Transforming Healthcare Operations Through AI
Beyond clinical applications, MLOps has also played a pivotal role in optimizing healthcare operations. From managing hospital resources to improving patient flow efficiency, AI-driven insights have enabled healthcare providers to make data-driven decisions that enhance operational effectiveness.
The Road Ahead for AI in Healthcare
The continued evolution of MLOps promises to drive further innovation in healthcare. With structured frameworks enabling the reliable deployment of AI, medical institutions can expect improved patient outcomes, enhanced diagnostic precision, and streamlined operations. As healthcare becomes increasingly data-driven, MLOps will remain at the forefront of AI integration, ensuring that technological advancements translate into tangible benefits for patients and healthcare providers alike.
In conclusion, Shivakrishna Bade highlights how MLOps is shaping the future of AI in healthcare, offering a structured and efficient approach to integrating machine learning into medical environments. As institutions embrace these frameworks, they unlock new possibilities for innovation, ultimately leading to better patient care and more efficient healthcare systems.
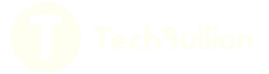