Introduction
Artificial Intelligence (AI) has undergone a revolutionary transformation in recent years, particularly in the realm of generative models. From the groundbreaking Generative Adversarial Networks (GANs) to the highly sophisticated Transformers, the evolution of AI generative models has been nothing short of remarkable. In this article, we will delve into the fascinating journey of these models, exploring their development, applications, and the impact they have had on various industries.
The Genesis: Generative Adversarial Networks (GANs)
The story begins with the inception of Generative Adversarial Networks (GANs), introduced by Ian Goodfellow and his colleagues in 2014. GANs marked a significant breakthrough in the field of AI by presenting a unique approach to generative modeling. The model consists of two neural networks—the generator and the discriminator—locked in a perpetual contest. The generator aims to create realistic data, while the discriminator strives to differentiate between real and generated data. This adversarial training process results in the generator producing increasingly convincing outputs.
Applications of GANs:
GANs have found applications across various domains, including image synthesis, style transfer, and even deepfake generation. In the world of art, GANs have been employed to create unique pieces, blurring the lines between machine-generated and human-created masterpieces. In healthcare, GANs have been instrumental in generating synthetic medical images for training algorithms, enhancing diagnostic accuracy.
The Rise of Variational Autoencoders (VAEs):
While GANs were making waves, another class of generative models emerged—the Variational Autoencoders (VAEs). VAEs operate on a different principle, combining elements of probabilistic modeling and neural networks. These models are capable of learning rich latent representations of data, allowing for the generation of diverse and realistic outputs.
The Emergence of Sequence-to-Sequence Models:
As the need for generating coherent sequences of data arose, sequence-to-sequence models became the focus of AI researchers. These models, often based on Recurrent Neural Networks (RNNs) or Long Short-Term Memory (LSTM) networks, excel in tasks like language translation, summarization, and text generation. The ability to handle sequential data opened up new possibilities for AI applications.
Enter the Transformers:
The true watershed moment in the evolution of AI generative models came with the introduction of Transformers. Originally designed for natural language processing tasks, Transformers demonstrated unparalleled capabilities in handling sequential data. The self-attention mechanism employed by Transformers allows them to capture dependencies between different parts of a sequence, making them highly effective in generating coherent and contextually relevant outputs.
Applications of Transformers:
Transformers quickly became the go-to architecture for a wide range of applications, including language translation, text summarization, and even image generation. GPT-3, one of the largest and most powerful Transformer models, showcased the potential of generative models by generating human-like text across diverse topics. The versatility of Transformers has led to their adoption in industries such as finance, healthcare, and marketing.
The Impact on Creative Industries:
The evolution of generative models has left an indelible mark on creative industries. Artists and designers now leverage AI to assist in the creative process, generating novel ideas and designs. AI-generated music, art, and literature are gaining recognition, challenging traditional notions of creativity and pushing the boundaries of what machines can achieve in collaboration with human creators.
Challenges and Ethical Considerations:
Despite their impressive capabilities, generative models, including GANs and Transformers, are not without challenges. Issues such as bias in generated content, ethical concerns surrounding deepfakes, and the potential misuse of AI technology raise important questions. As these models continue to advance, it becomes crucial to address these challenges and implement responsible AI practices.
Looking Ahead:
The journey from GANs to Transformers represents a trajectory of constant innovation and refinement in the field of AI generative models. As technology continues to evolve, we can anticipate further breakthroughs, with models becoming more sophisticated, efficient, and ethical. The collaboration between AI and human creativity is likely to redefine the boundaries of what is possible, opening up new horizons for innovation and discovery.
Conclusion:
The evolution of generative models stands out as a testament to human ingenuity and technological advancement. From the adversarial training of GANs to the attention mechanisms of Transformers, each phase in this journey has contributed to reshaping how we perceive and interact with AI. As we move forward, the symbiotic relationship between human creativity and AI capabilities promises a future where the unimaginable becomes reality, and the evolution of AI generative models continues to unfold.
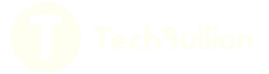