Welcome to a world where the battle against fraud is taken to new heights with cutting-edge technology. In an era of ever-evolving deception tactics, it’s crucial for businesses and individuals alike to stay one step ahead. Thankfully, we now have a powerful ally in the form of generative AI—a game-changing innovation that promises not only to identify fraudulent activities but also prevent them from occurring in the first place.
Introduction
Generative artificial intelligence (AI) is a rapidly growing field that holds immense potential in various industries, including fraud detection. In recent years, fraud has become increasingly prevalent and sophisticated, causing significant financial losses for businesses and individuals alike. Traditional rule-based systems and machine learning algorithms have been effective in detecting known types of fraud; however, they often struggle with identifying new or evolving fraudulent techniques.
This is where generative AI comes into play. Unlike traditional approaches that rely on pre-defined rules or training data, generative AI uses deep learning algorithms to understand patterns and generate new data based on those patterns. This enables it to detect anomalies and outliers that may represent fraudulent activities.
Traditional methods of fraud detection and their limitations
Traditional methods of fraud detection have been used for decades to identify and prevent fraudulent activities. These methods include manual review, rule-based systems, anomaly detection, and predictive modeling. While these approaches have been effective to some extent, they also come with limitations that can hinder their overall effectiveness in catching all types of fraud.
1. Manual Review:
Manual review involves human analysts manually reviewing transactions or documents to identify potential fraudulent activity. This method relies heavily on the experience and expertise of the reviewers and is time-consuming. It can also be prone to errors due to human judgment, leading to false positives or false negatives.
2. Rule-Based Systems:
Rule-based systems use a set of predefined rules to flag suspicious transactions or activities based on certain patterns or thresholds. While this method is faster than manual review, it is limited by its static nature. It can only catch known fraud patterns and may miss new types of fraud that do not fit into the existing rules.
3. Anomaly Detection:
Anomaly detection identifies unusual or abnormal behavior that deviates from normal patterns within a dataset. This method uses statistical algorithms to detect outliers and flag them as potentially fraudulent transactions. However, it can also lead to a high number of false alarms since legitimate transactions may also be flagged as anomalies.
4. Predictive Modeling:
Predictive modeling uses historical data and statistical techniques such as machine learning algorithms to predict future outcomes, including potential fraudulent activities. While this approach can be more accurate than other traditional methods, it requires large amounts of data.
How Generative AI Works in Detecting and Preventing Fraud
Generative artificial intelligence (AI) is a cutting-edge technology that has been making waves in the world of fraud detection and prevention. Unlike traditional rule-based systems, which rely on pre-defined rules and patterns to identify fraudulent activity, generative AI works by creating its own rules and patterns based on vast amounts of data.
The first step in using generative AI for fraud detection is training the system with a large dataset of both legitimate and fraudulent transactions. This allows the system to learn the underlying patterns and characteristics of fraudulent behavior. The more data it is trained on, the more accurate its predictions will be.
Once trained, generative AI uses algorithms to continuously analyze new data in real-time and identify anomalies or suspicious patterns that deviate from normal behavior. These anomalies are then flagged for further investigation by fraud analysts.
One key advantage of generative AI over traditional rule-based systems is its ability to adapt and evolve over time. As fraudsters constantly change their tactics, traditional systems struggle to keep up with these new methods. However, generative AI can learn from these changes and update its algorithms accordingly to stay ahead of emerging threats.
Another crucial aspect of how generative AI works in detecting and preventing fraud is anomaly detection. This involves identifying unusual or unexpected behaviors that do not fit into any known pattern or previous data points. For example, if a person suddenly makes large purchases outside of their usual spending habits or if there are multiple login attempts from different locations within a short period, this would be flagged as an anomaly.
Real-life examples of how companies have successfully used generative AI for fraud detection
Generative AI is a branch of artificial intelligence that involves using machine learning algorithms to generate new, unique data based on patterns found within existing datasets. By analyzing vast amounts of data, generative AI can create synthetic data that closely mimics real-life scenarios. This ability makes it highly effective in identifying fraudulent patterns and activities.
Let’s take a look at some real-life examples of how companies have successfully used generative AI for fraud detection:
1. PayPal: As one of the world’s leading digital payment platforms, PayPal is susceptible to various forms of fraudulent activity, such as account takeover and money laundering. To combat this issue, PayPal implemented generative adversarial networks (GANs), a type of generative AI, to analyze customer transaction data and detect anomalies in real-time. This has enabled them to identify suspicious transactions before they are completed, preventing potential losses.
2. American Express: Credit card fraud is a major concern for financial institutions like American Express. To address this issue, the company implemented generative modeling techniques to create fake credit card numbers, which were then inserted into their system alongside legitimate ones. Through continuous monitoring and analysis, any discrepancies or similarities between these fake numbers and actual fraudulent transactions were identified, allowing
Implementing generative AI in your organization to guard against deception
Implementing generative AI in your organization is a crucial step towards safeguarding against deception and fraud. With the rise of sophisticated fraudulent activities, traditional methods of detection are no longer sufficient. This is where generative AI comes into play—utilizing advanced machine learning algorithms to generate new data based on patterns learned from existing data.
The first step in implementing generative AI is to understand the specific needs and challenges of your organization. This includes identifying potential areas of vulnerability, such as customer interactions, financial transactions, or internal processes that may be susceptible to fraud. By understanding your organization’s unique risks, you can tailor the use of generative AI to address these specific concerns.
Once the potential areas for fraud have been identified, it’s essential to gather and analyze large amounts of data. The more data available for training the algorithm, the better it will perform in identifying fraudulent patterns. This can include historical transaction data, customer information, employee records, and any other relevant data sources.
Next, select a reputable vendor or build an in-house team with expertise in developing and deploying generative AI models. It’s crucial to choose a vendor that has experience working with organizations like yours and has a proven track record of success in detecting fraud using generative AI. If building an internal team, ensure they have the necessary skills and resources to continuously develop and maintain effective models.
Conclusion
As technology continues to advance, so do the tactics and schemes used by fraudsters. It is more important than ever for companies to stay ahead of these fraudulent activities in order to protect their customers and maintain trust. By leveraging generative AI, businesses can detect and prevent fraud at a much faster pace, saving time and money while also safeguarding against potential damages. With this powerful tool at our disposal, we can continue to stay one step ahead of deception and build a more secure future for all.
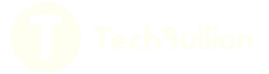