Welcome to a thought-provoking exploration of the fascinating realm where data science intersects with ethics – a topic that has gained significant attention in recent years. As we delve into the increasingly important field of data science, it becomes imperative to examine its ethical dimensions, particularly when it comes to striking the delicate balance between innovation and privacy. Just like any other groundbreaking technology, data science presents us with immense opportunities for progress alongside challenges that demand our careful consideration. Join us on this insightful journey as we navigate through the intricate web of ethical conundrums and discover how best to tread this uncharted territory while upholding our moral obligations.
Introduction to Data Science and its Impact
Data science is an interdisciplinary field that combines statistical analysis, computer science, and information technology to extract insights from data. It involves using advanced algorithms and tools to process large amounts of data and identify patterns and trends that can be used for decision making. The rise of big data has made data science an essential tool for businesses, organizations, and governments around the world.
The Impact of Data Science
Data science has transformed industries in significant ways by providing insights into customer behavior, improving operational efficiency, and identifying potential risks. For example, in the healthcare industry, data science has enabled precise diagnoses through medical imaging analysis and patient monitoring. In finance, it has facilitated fraud detection and risk management.
Furthermore, data science has also played a crucial role in technological advancements such as self-driving cars, virtual personal assistants like Siri or Alexa, recommendation systems on e-commerce sites like Amazon or Netflix. These innovations have undoubtedly enhanced our daily lives but have also raised ethical concerns about privacy.
Data Privacy Concerns
As more personal information is collected through various devices and platforms every day, individuals’ right to privacy becomes more vulnerable. With the rapid growth of big data analytics driven by advances in machine learning techniques such as artificial intelligence (AI), concerns around the misuse of this technology are also growing. For instance, AI systems can unintentionally discriminate against certain groups due to biased training datasets or unethical programming decisions.
The Need for Ethical Considerations in Data Science
With the increasing use of innovative data analytics, the need for ethical considerations in data science has become crucial. This includes establishing transparency in data collection and usage, protecting personal information and privacy, and addressing potential bias in algorithms.
Organizations responsible for handling sensitive data must also ensure that they have proper security measures in place to prevent data breaches. Data scientists need to adhere to ethical standards when building models and analyzing data to avoid any potential harm or discrimination towards individuals or communities.
Moreover, data scientists should continuously evaluate their models and algorithms to identify and correct any biases that may exist. It is essential to involve diverse perspectives in the development process to minimize any potential harm or discrimination.
Balancing Innovation and Privacy in Data Science
In today’s digital age, data has become the currency of innovation. With the rapid advancement of technology, more and more data is being collected and analyzed to gain insights and improve processes in various industries. This has given rise to the field of data science – the practice of extracting knowledge from data through advanced algorithms and techniques.
While data science brings immense potential for innovation, it also raises ethical concerns regarding privacy. Data privacy refers to an individual’s right to control how their personal information is collected, used, shared, or stored by organizations. In recent years, there have been numerous instances where companies have misused or mishandled personal data, resulting in breaches that have compromised people’s privacy.
This has led many experts to question whether businesses are prioritizing innovation at the expense of violating individuals’ right to privacy. However, it is essential to understand that balancing innovation with privacy in data science is not a zero-sum game. It is possible for organizations to harness the power of data while respecting individuals’ rights and maintaining ethical standards.
One way to ensure this balance is by implementing strong regulatory frameworks. Governments around the world are enacting laws such as the General Data Protection Regulation (GDPR) in Europe and California Consumer Privacy Act (CCPA) in the U.S., which give individuals more control over their personal information and hold businesses accountable for protecting their users’ privacy.
Ethical Considerations in Collecting and Using Data
In today’s digital age, data is becoming an increasingly important and valuable commodity. With the rise of technology and the internet, we are able to collect vast amounts of data from various sources such as social media, online transactions, and other digital interactions. This abundance of data has paved the way for a new field known as data science, which involves using statistical methods and algorithms to analyze and extract insights from this data.
However, with great access to data comes great responsibility. Data science has ethical implications that should not be overlooked. The collection and use of personal information raise questions about individual privacy, bias in algorithms, discriminatory practices, and potential misuse by companies or governments.
As a responsible society, it is crucial to consider the ethical considerations involved in collecting and using data. In this section, we will discuss some key aspects that need to be taken into account when dealing with sensitive information.
1. Privacy protection: One of the primary concerns in data science is ensuring the protection of individuals’ privacy rights. While gathering vast amounts of personal information can provide valuable insights for businesses or research purposes, it also raises significant privacy concerns. Personal information collected without consent can lead to breaches in confidentiality and compromise individuals’ rights to control their own personal information.
To ensure privacy protection, it is essential first to determine what type of information is being collected and why it is needed. Companies or researchers must clearly communicate their intentions for using the collected data to individuals before obtaining their consent. Additionally, proper security measures should be implemented to safeguard the data from unauthorized access or use.
2. Informed consent: Informed consent plays a critical role in ensuring ethical data collection. Consent is the process of obtaining permission from an individual to use their personal information for a specific purpose. This means that individuals must be informed about what data is being collected, by whom, and for what purpose. They must also have the option to opt-out at any time without repercussions.
However, obtaining informed consent can be challenging, especially in cases where individuals may not fully understand the implications of providing their personal information. Companies and researchers have a responsibility to clearly explain the potential risks and benefits involved in sharing their information before obtaining consent.
3. Bias and fairness: Data science relies heavily on algorithms and statistical models to extract insights from data. However, these algorithms can be biased based on the data they are trained on, leading to discriminatory practices and outcomes.
For example, if a recruitment algorithm is trained solely on data from male applicants, it may discriminate against female applicants by giving them a lower score due to factors such as gendered language or job history. It is crucial to address bias in algorithms by regularly reviewing and testing them for potential discrimination and ensuring fair practices in decision-making.
4. Data ownership: Another ethical consideration in data collection is determining who owns the data being collected and used. In many cases, individuals are not aware that their personal information is being collected and used for other purposes by companies or organizations.
Organizations have a responsibility to be transparent about their data collection practices and to obtain permission from individuals before using their data for other purposes. Individuals should also have control over their own personal information and the option to request its deletion or correction if needed.
5. Data security: The increase in data breaches and cybercrimes highlights the importance of maintaining proper data security measures. Companies and researchers must take all necessary precautions to safeguard personal information from unauthorized access, use, or manipulation.
This includes implementing encryption techniques, limiting access to sensitive information, regularly updating software, and having contingency plans in case of a security breach. Failure to maintain proper security can result in severe consequences for both the individual whose data was compromised and the organization responsible for safeguarding it.
The Role of Regulatory Bodies in Data Science Ethics
One of the most significant challenges in the field of data science is ensuring that ethical considerations are prioritized alongside technological advancements. As data continues to play an increasingly important role in decision-making processes, there arises a need for regulatory bodies to guide and govern the responsible use of data. In this section, we will discuss the role and importance of regulatory bodies in upholding ethics within data science.
Regulatory bodies are organizations, agencies, or institutions that have been established by governments or industry associations with the purpose of overseeing and regulating specific industries or practices. In terms of data science, these bodies serve as watchdogs to ensure that businesses and individuals are adhering to ethical standards when collecting, storing, analyzing, and using data.
One of the key responsibilities of regulatory bodies in data science ethics is setting and enforcing compliance with laws and regulations related to privacy and security. For instance, in the United States, there are several regulations such as the General Data Protection Regulation (GDPR) and Health Insurance Portability And Accountability Act (HIPAA) that protect consumer privacy. Regulatory bodies work closely with companies to ensure they meet these requirements when handling sensitive personal information.
Moreover, these bodies also play a crucial role in creating guidelines for responsible data collection practices. They establish rules for obtaining consent from individuals before gathering their information and maintaining transparency about how their data will be used. By setting standards for ethical behavior within industries utilizing big data technologies such as machine learning algorithms or artificial intelligence systems, regulatory bodies help safeguard against potential biases or discriminatory practices.
In addition to setting regulations and guidelines, regulatory bodies also conduct audits and investigations to ensure compliance. This involves reviewing an organization’s data management processes, assessing the validity of its data sources and methods, and identifying any potential ethical concerns. These audits help identify areas where companies may be falling short in terms of ethical data practices, allowing for corrective action to be taken.
Another essential function of regulatory bodies is providing resources and education on ethical data practices. They offer training programs, workshops, and other educational materials to individuals working in the field of data science. By promoting awareness and knowledge of ethical considerations within data science, these bodies strive to create a culture that prioritizes responsible handling of data.
Lastly, by enforcing ethical standards within the industry, regulatory bodies contribute to the overall trustworthiness of data science as a field. The public is more likely to have confidence in organizations that demonstrate a commitment to ethical behavior when it comes to handling personal information. This trust is crucial for the widespread acceptance and adoption of new technologies that rely on data.
Examples of Data Science Ethics Gone Wrong
Data science ethics is an increasingly important topic as the field of data science continues to grow and impact our daily lives. While data science has the potential to bring about great advancements, it also comes with its own set of ethical concerns. In this section, we will discuss some examples of data science ethics gone wrong and their implications.
1. Cambridge Analytica Scandal:
One of the most well-known examples of data science ethics gone wrong is the Cambridge Analytica scandal. It involved the political consulting firm Cambridge Analytica collecting personal information from millions of Facebook users without their consent through a third-party app. This data was then used to create targeted ads for political campaigns during the 2016 US presidential election.
The scandal shed light on the lack of transparency and control over user’s personal information on social media platforms, bringing into question the ethical use of this data in influencing public opinion. It also sparked debates on whether or not companies should have access to such extensive amounts of personal information without explicit consent from users.
2. Biased Algorithms:
Another example that highlights the importance of ethical considerations in data science is biased algorithms. Algorithms are often viewed as unbiased decision-making tools, but they can reflect biases and perpetuate discrimination if trained on biased datasets or programmed by individuals with inherent biases.
For instance, Amazon had to shut down an AI recruiting tool after discovering it was biased against women due to being trained on male-dominated resumes from past hires. This example shows how even unintended biases can seep into algorithms and result
Best Practices for Responsible Data Science
As data science continues to advance and shape our society, it is crucial to consider the ethical implications of how we collect, store, analyze, and use data. Data privacy and security have become major concerns in recent years, with various high-profile data breaches and controversies surrounding companies’ use of personal data. This has led to a growing need for responsible practices in the field of data science.
Here are some best practices that can help ensure responsible data science:
1. Transparency
Transparency is key when it comes to responsible data science. Organizations collecting and using data should be transparent about their processes and policies regarding data collection, storage, usage, and sharing. This includes being upfront with users about what kind of data is being collected, for what purposes it will be used, who will have access to it, and how long it will be stored.
2. Informed Consent
Obtaining informed consent from individuals whose data is being collected should also be a top priority. This means ensuring that individuals are fully aware of how their personal information will be used before giving their consent. Organizations should clearly explain the terms and conditions associated with the use of their services or collection of their information.
3. Anonymization and De-identification
Data anonymization refers to the process of removing identifiable information from datasets to protect individuals’ privacy while still allowing for analysis on the remaining anonymous data. De-identification involves altering or removing specific identifying characteristics from a dataset that could potentially identify an individual. These practices should be used whenever possible to protect individuals’ privacy and prevent misuse of their data.
4. Data Minimization
Data minimization is the practice of collecting only the minimum amount of data needed for a specific purpose. Instead of collecting all available data, organizations should carefully consider what information is necessary for their analysis and limit their collection to that specific data. This reduces the risk of unnecessary personal information being collected and helps protect individuals’ privacy.
5. Data Security
Organizations must take appropriate measures to ensure the security and protection of the data they collect. This includes implementing secure systems for data storage, limiting access to sensitive information, regularly backing up data, and monitoring for any potential threats or breaches.
6. Ethical Standards
Data scientists should adhere to ethical standards when conducting research and analyzing data. This includes respecting individuals’ rights to privacy and confidentiality, avoiding bias in data analysis, ensuring transparency in reporting results, and obtaining proper consent when using human subjects in research.
7. Ongoing Review and Oversight
Responsible data science requires ongoing review and oversight to ensure that processes are aligned with ethical principles. Organizations should have procedures in place for regularly reviewing their policies and practices regarding data collection, usage, storage, sharing, and security to ensure they are in line with ethical standards.
8. Education and Awareness
Education and awareness play a vital role in promoting responsible data science practices. Data scientists, as well as individuals whose data is being collected, should be educated on the importance of ethical data handling and best practices for ensuring privacy and security.
Future Implications and Challenges for Ethical Data Science
The rapid advancement of technology and the increasing availability of data have led to the emergence of a new field – Data Science. This powerful tool has revolutionized industries such as healthcare, finance, and marketing by leveraging large amounts of data to provide valuable insights and improve decision-making processes.
However, with great power comes great responsibility. As data science continues to grow and evolve, it brings forth several ethical implications and challenges that must be addressed in order to maintain a balance between innovation and privacy.
Privacy Concerns
One of the major concerns surrounding data science is the invasion of privacy. In order for data scientists to extract meaningful insights from data, they often need access to personal information such as browsing history, location data, and social media activity. With this level of access comes the risk of misuse or mishandling of sensitive information.
The recent Cambridge Analytica scandal is a prime example where personal Facebook data was harvested without consent for political purposes. This raised serious questions about the ethical implications of using big data without proper regulations and guidelines in place.
Bias in Algorithms
Another challenge for ethical data science is ensuring impartiality in algorithms used for decision making. Data scientists face the difficult task of handling biased historical datasets while trying to develop unbiased algorithms. These biased algorithms can have serious consequences on individuals or groups, especially when used in fields like finance or criminal justice.
Conclusion: Striking the Right Balance between Innovation and Privacy in Data Science
Data science has undoubtedly revolutionized the way we collect, analyze, and utilize information. It has immense potential to drive innovation and progress in various industries such as healthcare, finance, transportation, and many more. However, with great power comes great responsibility.
As discussed in this article, data science also raises several ethical concerns regarding privacy infringement. The increasing use of big data and advanced technologies have made it easier for companies to collect vast amounts of personal information without individuals’ consent or knowledge. This not only violates basic human rights but also poses threats to individuals’ autonomy and freedom.
Therefore, striking the right balance between innovation and privacy in data science is crucial. It requires a holistic approach that considers both the benefits of utilizing data science and the potential risks it brings along.
One way to achieve this balance is by implementing strong regulations on data collection and usage practices. Governments around the world are already taking steps towards protecting individuals’ privacy rights through laws like GDPR (General Data Protection Regulation) in Europe, CCPA (California Consumer Privacy Act) in California, USA; PIPEDA (Personal Information Protection & Electronic Documents Act) in Canada; among others.
These regulations aim to enforce transparency about how personal data is collected, used, stored, shared or sold by organizations. They also give individuals greater control over their personal information by allowing them to access their data or opt-out of any marketing campaigns they do not wish to be.
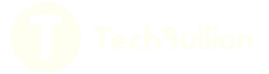