Introduction
Artificial Intelligence (AI) has undoubtedly revolutionized various industries, with AI generative models playing a pivotal role in tasks such as content creation, language translation, and even image synthesis. However, as these models continue to advance, there is a growing need to address the ethical considerations surrounding their development and deployment.
Understanding AI Generative Models
AI generative models, particularly those based on deep learning techniques, have the ability to generate new content that mimics human-like patterns. Whether it’s generating text, images, or even music, these models have demonstrated remarkable capabilities. However, the very power that makes them impressive also raises ethical concerns.
Challenges in Ethical AI Development
Bias and Fairness
One of the primary ethical challenges in AI generative models is the potential for bias in the data on which they are trained. If the training data is biased, the model can perpetuate and even amplify existing societal biases. For example, a language model trained on biased text data may produce output that reflects and reinforces stereotypes.
To address this challenge, developers must ensure diverse and representative datasets, encompassing various demographics and perspectives. Implementing fairness metrics during the model evaluation phase can also help identify and rectify biases.
Transparency and Explainability
AI generative models, especially those utilizing complex neural networks, are often criticized for their lack of transparency. Understanding how these models arrive at specific outputs can be challenging, making it difficult to hold them accountable for potential errors or biased results.
Ensuring transparency in the development process and promoting explainability in AI models is crucial. Researchers are actively working on developing methods to interpret and explain the decisions made by these models, providing insights into their inner workings.
Security and Misuse
The powerful capabilities of AI generative models can also be exploited for malicious purposes, such as creating deepfakes or generating misleading information. Ensuring the security of these models and preventing their misuse is a significant ethical concern.
Implementing robust security measures, including authentication and authorization protocols, can help mitigate the risk of misuse. Additionally, promoting awareness about the potential negative consequences of AI-generated content is essential to prevent its unwitting dissemination.
Solutions to Ethical Challenges
Ethical Guidelines and Standards
Establishing clear ethical guidelines and standards for the development and deployment of AI generative models is a crucial step. These guidelines can outline best practices for data collection, model training, and evaluation, emphasizing fairness, transparency, and accountability.
Adhering to ethical standards ensures that AI developers prioritize responsible practices and consider the societal impact of their creations. Industry-wide collaboration is essential to create and enforce such standards effectively.
Diverse and Representative Data
To mitigate bias in AI generative models, it is imperative to use diverse and representative datasets. Developers should actively seek out and include data from underrepresented groups to ensure that the models produce fair and unbiased outputs.
In addition to using diverse data, continuous monitoring and auditing of models for potential bias can help identify and rectify issues as they arise. Regular updates to the training data can also contribute to improving model performance over time.
Explainability and Interpretability
Improving the explainability and interpretability of AI generative models is crucial for fostering trust and accountability. Researchers are exploring techniques such as attention mechanisms and model-agnostic interpretability methods to provide insights into how these models make decisions.
By making the decision-making process more transparent, users and developers can better understand and address potential ethical concerns. Open communication about the limitations and uncertainties of AI models is essential for responsible deployment.
Public Awareness and Education
Promoting public awareness and education about the capabilities and limitations of AI generative models is essential in preventing misuse. Educating users about the existence of AI-generated content and how to discern between authentic and manipulated information can empower them to make informed decisions.
Public awareness campaigns can also serve as a deterrent against the malicious use of AI-generated content, creating a more vigilant and discerning online community.
Conclusion
As AI generative models continue to advance, addressing the ethical considerations surrounding their development and deployment is paramount. By acknowledging and actively working to overcome challenges such as bias, lack of transparency, and security risks, the AI community can ensure that these powerful tools are used responsibly and ethically. Establishing clear guidelines, prioritizing diverse and representative data, improving model explainability, and fostering public awareness are key steps toward creating a future where AI generative models contribute positively to society while minimizing potential harm.
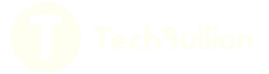