In our interconnected world, the digital frontier has become a battleground for individuals and organizations alike. With the ever-evolving tactics employed by hackers, the threat of cyberattacks looms large. However, there is a powerful ally in this fight against unseen adversaries: machine learning. This groundbreaking technology is revolutionizing cybersecurity, empowering us to stay one step ahead of cybercriminals. In this blog post, we will explore the fascinating intersection of artificial intelligence and online security, and discover how machine learning is transforming the way we protect ourselves on the digital battlefield.
Introduction to Machine Learning and Cybersecurity
As the digital world grows increasingly complex and interconnected, protecting against cyber threats becomes more challenging. Machine learning offers a powerful solution to help organizations keep pace with this ever-changing landscape. Machine learning algorithms excel at analyzing data, making them highly effective in identifying potentially malicious activity. Additionally, they can automatically generate security rules and enhance the accuracy of intrusion detection systems.
By leveraging machine learning in their security operations, organizations can improve their ability to detect and respond to threats promptly. Machine learning also helps reduce the false positive rate of security alerts, enabling security teams to focus on critical issues. As machine learning technology continues to evolve, it will play an increasingly vital role in securing the digital frontier.
How ML is Used in Cybersecurity
Over the past decade, cyberattacks have increased dramatically, becoming more sophisticated and harder to detect. To keep up with this evolving threat landscape, cybersecurity experts are turning to machine learning (ML). ML is being utilized in various ways to enhance cybersecurity. It aids in developing better intrusion detection systems, identifying malware, automatically blocking malicious traffic, and even detecting anomalies in network activity.
Although the use of ML in cybersecurity is still in its early stages, it has already shown great promise. As the technology continues to advance, we can expect even more innovative applications of ML in cybersecurity.
Benefits of Using ML for Cybersecurity
Organizations are adopting machine learning (ML) for cybersecurity due to several key benefits it offers:
- ML helps identify patterns in data that may indicate a security breach.
- ML can automatically block known malicious IP addresses or domains.
- ML monitors user behavior and flags suspicious activity.
- ML aids organizations in understanding their overall security posture and vulnerabilities.
- ML assists in developing more effective cyber defenses by providing insights into the latest threats and attack methods.
Common ML Algorithms Used in Cybersecurity
Several machine learning algorithms are commonly employed in cybersecurity applications, including:
– Anomaly detection: Identifies unusual behavior that may indicate a security breach by comparing it to what is considered “normal.”
– Classification: Distinguishes between benign and malicious data by training models on labeled data.
– Clustering: Groups together similar data points, useful for grouping malware samples with shared characteristics.
– Regression: Predicts values based on past data, such as the likelihood of a user clicking on a malicious link in an email.
– Neural networks: Recognizes patterns in data by training models on labeled data.
Challenges Faced with Implementing ML for Cybersecurity
Implementing machine learning (ML) for cybersecurity comes with unique challenges.
One obstacle is the need for large amounts of data to train effective models, which can be challenging to acquire as organizations are often hesitant to share information about security breaches. Additionally, the lack of labeled datasets makes it difficult to train supervised learning models accurately, given the rapid evolution of cybersecurity threats.
Another challenge is the “black box” problem associated with many ML models, making it challenging to understand their decision-making process. Lack of interpretability could pose risks if ML-based systems were used for critical security decisions without human oversight. There is also the potential for cybercriminals to exploit ML vulnerabilities, making it a target for attackers.
Despite these challenges, ML holds great promise for transforming cybersecurity. Organizations that overcome these obstacles will be well-positioned to take advantage of this transformative technology.
Best Practices for Securing the Digital Frontier
In the digital age, cybersecurity is more crucial than ever. Machine learning plays an increasingly vital role in securing the digital frontier. Here are some best practices for using ML to improve cybersecurity:
- Use machine learning for anomaly detection to identify potentially malicious behavior.
- Train ML models on known threats to recognize patterns and detect future attacks.
- Utilize unsupervised machine learning to detect previously unknown threats and sophisticated attacks.
- Combine machine learning with other security measures for a comprehensive security strategy.
- Keep machine learning models up to date to effectively detect and protect against new threats.
Conclusion
In conclusion, as the digital frontier evolves, organizations must adapt to the ever-growing cyber threats. Machine learning integrated into cybersecurity solutions helps detect potential threats and respond quickly to mitigate damage. Machine learning has become an invaluable tool for businesses to protect their digital assets and secure their data against potential breaches. With its transformative capabilities, machine learning is reshaping the landscape of cybersecurity, making the digital world a safer place for all.
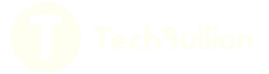