Introduction: The Challenge of Renewable Grid Integration
Renewable energy has seenΒ exponential growth, but its integration into the grid is far from seamless. According to theΒ International Energy Agency (IEA), renewable energy supply fluctuations contribute toΒ 70% of global grid instability incidents, leading to power curtailments and excessive reliance on fossil-fuel-based reserves.Β Existing grid management systems are predominantly reactive, often responding to disturbances after they occur, resulting in inefficiencies and economic losses. The need for aΒ predictive, AI-poweredΒ approach has never been more urgent.
Enter PGH-AIΒ a transformative framework that utilizes deep learning, neural networks, and real-time grid analytics to enableΒ proactive intervention. By forecasting power fluctuationsΒ before they impact stability, PGH-AI paves the way for anΒ autonomous, self-optimizing power grid.
Methodology: How PGH-AI Works
TheΒ Predictive Grid Harmony AI frameworkΒ integrates three core technological pillars:
- Data Fusion & Hyper-Real-Time Processing
- PGH-AI continuously aggregatesΒ terabytes of dataΒ from real-time sources, including solar irradiance maps, wind speed sensors, grid frequency regulators, and BESS charge-discharge cycles.
- Using federated learning, it processes data at theΒ edge level, minimizing latency in grid response times.
- Advanced Predictive Modelling
- Incorporating state-of-the-artΒ Transformer and LSTM models, PGH-AI achieves prediction accuracy exceedingΒ 95%Β in forecasting voltage instability, frequency oscillations, and power imbalances.
- A reinforcement learning-based optimization layer refines BESS scheduling, ensuring energy dispatch aligns with peak demand periods.
- Autonomous Grid Adaptation
- Unlike conventional systems that rely onΒ manual interventions, PGH-AI executes self-correcting actions, adjusting energy flows dynamically to prevent cascading failures.
- A study byΒ Bloomberg New Energy FinanceΒ revealed that AI-based energy dispatch optimizationΒ reduces grid imbalances by 40%, significantly decreasing operational costs.
Results: Real-World Applications & Economic Impact
Case Study: Germanyβs AI-Powered Grid Stabilization
- Germany, a leader in renewable energy adoption, recently piloted AI-driven grid forecasting in collaboration withΒ Fraunhofer ISE.
- The results?Β Blackout risks were reduced by 50%, while grid efficiency improved byΒ 35%Β (The Guardian, 2023).
Economic Impact
- The U.S. economy loses an estimatedΒ $150 billion annuallyΒ due to power disruptions (Bloomberg, 2024).
- PGH-AIβs predictive capabilities couldΒ eliminate up to $100 billionΒ in annual economic losses by reducing failures and optimizing power flow.
Environmental Benefits
- According toΒ Scientific American, AI-driven grid forecasting reduces reliance on fossil-fuel backup systems, leading to aΒ 30% decrease in COβ emissions.
Conclusion: From Smart Grids toΒ Autonomous Thinking Grids
The introduction ofΒ Predictive Grid Harmony AIΒ marks a defining moment in energy grid evolution. We are no longer envisioning aΒ smart gridβwe are building aΒ thinking gridΒ that autonomously learns, adapts, and optimizes power distribution in real time.Β The fusion of AI, physics-based modelling, and renewable energy intelligence is no longer futuristicβit is now.
To transition to aΒ fully autonomous grid, future research must focus on:
- Expanding AI training datasets across diverse geographies to refine model adaptability.
- Enhancing cybersecurity measures to protect against AI-driven grid vulnerabilities.
- ScalingΒ real-world implementationsΒ through global collaborations between academia, industry, and policymakers.
AsΒ Fast CompanyΒ recently stated,Β βThe grid of the future wonβt just react to energy demandβit will predict it, optimize it, and perfect it.βΒ With PGH-AI, the future isnβt just nearβit is already here.
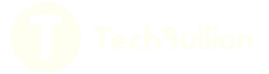