Data is the new gold. When businesses can extract and analyze enough of the right data, they make better decisions, increase efficiency, and improve productivity. Web scraping enables companies to harvest large quantities of information from social media platforms and websites and store it in one central location.
Just like raw ore, however, this data must be refined to be most effective. The refining and polishing process that brings value happens best when interdisciplinary teams come together during data preprocessing, data analysis, and predictive modeling. Neil Emeigh, founder and CEO of Rayobyte, explains the process that empowers companies to understand the information they obtain from data scraping and use it to make critical decisions.
Web scraping must be followed by data preprocessing
Data preprocessing is an essential step in the data analytics process and involves cleaning, transforming, and formatting data so that it can be used for analysis. Data preprocessing ensures that businesses are analyzing accurate and reliable data.
Companies clean data by removing noise, outliers, and missing values from their datasets. They then transform that data by aggregating it into usable groups or merging datasets with similar variables, after which they can interpret the data and select the information that is most useful in their decision-making process.
“It’s easiest to think about data preprocessing in terms of a goldmine,” Emeigh remarks. “When you mine gold, you bring rock and ore and a lot of other stuff out of the ground, but that material is worthless until it is converted into pure gold. Data preprocessing accomplishes the same function when you’re mining data — web scraping gathers data, and preprocessing ensures it is useful in driving business decisions.”
Preprocessing leads to data analysis and insights
Data analysis is the process of inspecting data to discover useful information, suggest conclusions, and support decision-making. Data analysts use machine learning algorithms to find patterns in large datasets and make predictions about future events or trends, aiding data-driven decision-making by identifying the right questions to ask and answering them in meaningful ways.
“When an investor chooses a stock or a venture, they never commit their hard-earned money without looking at the previous quarter’s performance or historical reports,” Emeigh asks. “They check trends, industry benchmarks, and other data to be confident in their decision. By the same token, it also makes sense to use data analysis and insights as you invest in marketing, HR, production, and other areas of your business. You extract these insights from the data you gather from your own business and from public data. When it comes to public data, you cannot extract all the insights you need without scraping. Data scraping saves you thousands of dollars and helps you find the insights you need quickly.”
The right data offers predictive modeling
Predictive modeling uses historical data to make predictions about upcoming events. In the business world, it allows companies to use information about today’s customers to make accurate decisions based on how customers will behave in the future.
Predictive models help organizations make better decisions every day by providing insight into their current customer base. By examining past behavior, they can know how likely each customer is to make a purchase. This allows them to understand which segments are most valuable and which are most worth targeting.
However, predictive modeling requires mountains of data to provide accurate models. Web scraping enables businesses to obtain historical sales figures, product prices, and other metrics that offer insights into customers and predict future behavior. It allows businesses to extract data relevant to their products and services from all over the web. This powerful tool gives even companies with limited resources or time constraints the data they need to make informed decisions regarding marketing campaigns or product development.
“In natural language processing, generating sales forecasts, and even preparing for hurricanes, predictive modeling has improved and impacted almost every aspect of every industry,” Emeigh explains. “The key to predictive modeling is collecting billions of data points to create an accurate model. There’s no way a human can collect the amount of data needed. Web scraping plays a vital role in extracting the data we use to build predictive models in every industry.”
The importance of an interdisciplinary approach to data analysis
An interdisciplinary approach to data analysis involves multiple fields working together on one project to reach a better understanding of the issue at hand. It is the most effective means of turning raw data into data-driven decisions.
“It’s like putting together a team of superheroes to save the day,” says Emeigh, “and web scraping is like the sidekick to the interdisciplinary team. It gathers data from various sources and saves the team hours of tedious manual work.”
As an example, a healthcare team collecting patient data for a predictive modeling project might not consider social media — at least not at first. But social media platforms offer massive amounts of data, and a social media marketer knows just where to look.
“When experts from different fields work together, they are better able to solve complex problems and come up with more creative solutions,” observes Emeigh. “By working together, they see data from different angles, develop more comprehensive understandings, and generate ideas they might not have otherwise.”
Web scraping is the integral tool behind these processes. It gathers critical data before preprocessing, analysis, predictive modeling, and interdisciplinary teams turn it into decisions that are worth more than gold to their organization.
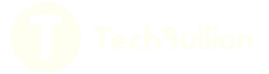