Security threats keep on growing with each passing moment. To beat the cybercriminals, your organization needs enterprise-class security with advanced measures. Traditional SOCs rely on human analysts to monitor, detect, and respond to threats. In this slow way, it cannot cope with modern complex cyberattacks.
To that end, autonomous SOCs use AI to detect, analyze, and respond to incidents in real-time. They promise swifter and more accurate threat management. However, the adoption of an autonomous SOC comes with challenges. An organization must overcome these to realize the full potential of AI and automation in cybersecurity.
This article covers the main components, benefits, challenges, and strategies for implementing autonomous SOCs.
Understanding Autonomous SOCs and Its Components
An autonomous SOC is an advanced security system using AI and automation for threat detection. This frees up the teams to tackle sophisticated threats by minimizing manual tasks. It will make use of machine learning, security orchestration, and instantaneous response mechanisms that together smoothen and strengthen cybersecurity mechanisms. The main components of an autonomous SOC include:
Machine Learning Algorithms
These models use prior attack patterns and live data to detect abnormal behavior and threats.
Security Orchestration and Automation
This speeds up incident response by reducing time-to-containment and eradicating threats.
Threat Intelligence Integration
AI-driven SOCs use global threat intelligence for early cyber-attack prediction and prevention.
Real-time Monitoring and Analytics
An autonomous SOC monitors, on a continual basis, logs, network traffic, and endpoints for threat detection at its inception stage.
Benefits of an Autonomous SOC
An autonomous SOC enhances security operations in several ways. It detects threats faster, thereby reducing response times to cyberattacks. Automation of repetitive tasks reduces manual work and frees up teams for strategy and complex threat analysis. It scales seamlessly, handling massive data without overloading staff. Accuracy improves too, with fewer false alarms ensuring real risks get swift, focused attention. These upgrades will help build a more adaptive, resilient cybersecurity framework.
Key Challenges in Implementing Autonomous SOCs
-
Data and Integration Challenges
Autonomous SOC demands a huge volume of security data. It is expected to process security logs, network traffic, and endpoint activities in near real-time. Nonetheless, data management and integration become immensely complex. Common challenges organizations face include:
-
Handling Large Data Volumes
Most of the data in security operations is generated on a very large scale and involves advanced capabilities to process it.
-
Integrating with Existing Security Tools
Many organizations have outdated security tools that don’t integrate effectively with AI automation.
-
Ensuring Data Accuracy and Consistency
Poor quality of data negatively impacts AI decision-making in incorrect threat detection.
Limitations of AI and Machine Learning
AI is an excellent tool to use for threat detection, but it has its limitations. Among the biggest challenges is making sure the accuracy of threat identification is correct. Some common issues are:
-
False Positives and Negatives
Incorrect threat detection may lead to resource wastage and security gaps.
-
Training Models Effectively
Intelligent machines need quality training data to enhance their predictive capabilities.
-
Keeping Up with Evolving Threats
Most of the legacy security systems are not flexible enough to keep pace with evolving threats.
-
Workforce and Skill Gaps
Automation reduces manual workloads, but skilled cybersecurity professionals are still required. Most SOC teams lack the necessary skill sets to manage autonomous SCO effectively. Challenges include:
Bridging the AI Expertise Gap
Cybersecurity professionals should have training in AI and machine learning to reap full benefits from an autonomous SOC.
Upskilling Existing Teams
Organizations have to make education and continuous learning part of investments in technology to stay ahead of the pace.
Overcoming Resistance to Automation
The analysts will feel that their jobs will be taken away by AI-driven SOC and, hence will resist adopting one.
-
Regulatory and Compliance Challenges
Compliance is one big factor in the cybersecurity industry. AI-driven SOC needs to maintain industry regulations and data protection laws. Key challenges include:
Working Around Data Privacy Regulations
Regulations such as GDPR and CCPA have placed strict regulations around security data collection and processing.
Compliance with Security Standards
Autonomous SOCs will need to conform to industry-specific cybersecurity frameworks, such as NIST and ISO 27001.
-
Addressing AI Transparency and Accountability
Organizations must be able to explain security decisions taken by AI and ensure that they are auditable.
Strategies for Overcoming Implementation Challenges
-
AI and Machine Learning Capabilities
- To improve AI accuracy, organizations must refine machine learning models by:
-
Using diverse and high-quality training datasets.
- Applying explainable AI to drive transparency in decision-making.
- Implementation of the hybrid approach involves both AI automation and human oversight.
-
Strengthening Data Integration and Interoperability
- Organizations must ensure seamless communication between security tools by:
-
Standardizing data formats and security protocols
- Using Security Information and Event Management (SIEM) solutions to centralize security data.
- Lay down solid API integrations to interface the AI-driven SOCs with already deployed security infrastructure.
-
Workforce Development and Change Management
Successfully adopting these processes requires investment in workforce training as well as in change management, including:
Training provided to SOC analysts on AI and automation
- Collaboration of the security teams with the AI and machine learning professional teams.
- Offer a balanced security model where the human factor plays an important complementary role in such AI-driven automation.
-
Compliance and Ethical Considerations
Organizations should consider:
- Applying AI governance frameworks that outline the use of responsible AI.
- Ensuring transparency in automated security decisions.
- Keeping AI implementations aligned with ever-evolving regulatory requirements to maintain compliance.
Future Trends and Evolving Solutions
Advancements in AI-Driven Cybersecurity
Artificial intelligence technology is continuously improving. Future innovations will involve self-learning models of AI that can adapt to new threats in real time. There will also be better behavioral analytics to spot subtle attack patterns. Plus, AI-driven threat intelligence sharing will boost global cybersecurity collaboration.
Emerging Technologies Supporting Autonomous SOCs
New technologies enhance the capability of the autonomous SOC. These are Extended Detection and Response, which integrate many layers of security. Scalability and flexibility are provided by cloud-based AI SOCs. Blockchain-based security solutions enhance data integrity and authenticity.
Role of Human Analysts in AI-Powered Security
Automation will not replace human analysts but change their roles. The analysts will be focusing on:
- Oversee all AI-driven investigations to affirm threat findings.
- Handling complex, targeted attacks that require human expertise.
- Development of the training models for AI for continuous improvement in machine learning algorithms.
Conclusion
The implementation of an autonomous SOC is complex but rewarding. Organizations must address issues related to data integration, limitations of AI, personnel training, and regulatory compliance for a successful implementation.
Automation can reach its full potential if the organization enhances AI, integrates data, and trains teams. As AI-driven cybersecurity continues to rise, so will the efficiency, scalability, and proactive measures of the SOC. The future of security is one where a mix of AI and human expertise protects digital assets.
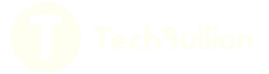