In the fast-paced and ever-changing world of finance, staying ahead of potential pitfalls is crucial for success. Fortunately, a powerful solution is emerging: machine learning. This cutting-edge technology is revolutionizing risk analysis in finance, paving the way for smarter decision-making and mitigating uncertainties. In this article, we will explore how machine learning is transforming risk analysis, its applications in the financial industry, advantages, challenges, and the future it holds.
Introduction to Machine Learning and Its Role in Risk Analysis
Machine learning, a subset of artificial intelligence, focuses on constructing and studying algorithms that learn from data and make predictions. In risk analysis, machine learning plays a vital role by identifying risks and predicting financial outcomes.
Machine learning algorithms can be applied to various tasks in risk analysis, including pattern recognition, anomaly detection, and future event prediction. The technology’s strengths lie in its ability to handle large data sets, identify complex patterns, and make predictions based on limited data.
Different types of machine learning algorithms are used in risk analysis. Supervised learning algorithms are trained on labeled data, such as historical data, to predict specific outcomes. Unsupervised learning algorithms find patterns in data without predefined labels, while reinforcement learning algorithms learn through trial and error, receiving rewards for correct actions and punishments for incorrect actions.
How Machine Learning is Used in the Financial Industry
The financial industry is increasingly leveraging machine learning to mitigate risks. Financial institutions use machine learning models to identify risk factors, predict financial losses, and develop early warning systems.
Machine learning’s strength in handling large data sets and uncovering patterns that human analysts might miss makes it ideal for risk analysis in finance. It enables models to recognize fraudulent activity, assess creditworthiness, and predict market trends. By employing machine learning, financial institutions gain a deeper understanding of risks and can take proactive steps to mitigate them.
Advantages of Using Machine Learning for Risk Analysis
Machine learning offers several advantages for risk analysis:
- Quick risk identification: Machine learning algorithms can identify risks more rapidly.
- Accurate risk identification: Machine learning algorithms enhance risk identification accuracy.
- Time-saving: Machine learning reduces the time spent on risk analysis.
- Improved risk management: Machine learning helps enhance overall risk management strategies.
- Early risk detection: Machine learning aids in identifying potential risks before they escalate.
- Data-driven decision-making: Machine learning enables data-driven decisions in risk management.
- Automation: Machine learning automates the risk analysis process, delivering faster and more accurate results.
Challenges Faced When Implementing ML in Finance
Implementing machine learning in finance comes with challenges that must be addressed to maximize its transformative impact. Some key challenges include:
Biased data sets: Training machine learning models with biased data can lead to inaccurate predictions. High-quality, representative data sets are crucial to mitigate this risk.
Explainability: Explaining the reasoning behind a machine learning model’s predictions can be difficult. Transparency and accountability are particularly important in finance, necessitating efforts to address this challenge.
Continuous model updating: Models must be regularly updated with new data to maintain accuracy. While this process can be costly and time-consuming, it is vital to avoid using outdated models.
Despite these challenges, machine learning holds significant promise for risk analysis and management in finance. Overcoming these obstacles can enable financial institutions to improve efficiency, effectiveness, and decision-making outcomes.
Applications of ML in Risk Management and Avoidance Strategies
Machine learning finds applications in various industries for risk management purposes. In finance, it has been extensively utilized for fraud detection, risk modeling, and stress testing. ML is also making inroads in healthcare for predicting disease risks, improving patient safety, and optimizing pricing and fraud prevention in retail and manufacturing.
As data becomes more abundant and complex, machine learning will play an increasingly vital role in risk management and avoidance strategies. Organizations that embrace machine learning can proactively mitigate risks and avoid costly mistakes in the future.
The Future of Machine Learning in Risk Analysis
As technology continues to advance, machine learning’s potential for risk analysis grows. Machine learning offers several advantages for risk analysis:
Improved risk identification: Machine learning algorithms can quickly identify risks that may be challenging for traditional methods.
Enhanced understanding of data relationships: Machine learning can help build more accurate models by considering a broader range of data points and their relationships.
Faster and more accurate decision-making: Machine learning can process data rapidly, leading to faster and more accurate decision-making.
Automation: Machine learning enables the automation of risk analysis processes, reducing time and effort.
Conclusion
machine learning is set to revolutionize risk analysis in finance, enabling more accurate and cost-effective risk mitigation strategies. Embracing this technology empowers financial institutions to make better decisions, reduce potential losses, and navigate the complex financial landscape with confidence.
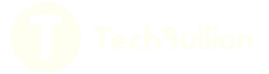