In the ever-evolving world of pharmaceutical research and development, one tool is quickly rising to the top as a game-changer: artificial intelligence. With its ability to analyze vast amounts of data, predict outcomes, and streamline processes, AI has the power to revolutionize drug discovery and development. Join us as we explore how harnessing the power of AI is shaping the future of medicine and paving the way for groundbreaking advancements in healthcare.
Introduction to AI and its relevance in the pharmaceutical industry
Artificial Intelligence (AI) has become a buzzword in various industries, including pharmaceuticals. The use of AI in drug discovery and development has shown immense potential for accelerating the process and making it more efficient. With advancements in technology, the pharmaceutical industry is now able to leverage AI to analyze vast amounts of data and make predictions for drug discovery, clinical trials, and personalized medicine.
AI refers to the simulation of human intelligence processes by machines, specifically computer systems. It involves techniques such as machine learning (ML), natural language processing (NLP), and deep learning (DL) to perform tasks that typically require human intelligence. ML enables computers to learn from data without being explicitly programmed, while NLP allows them to understand and process human language. DL is a subset of ML that uses artificial neural networks with multiple layers to solve complex problems.
The relevance of AI in the pharmaceutical industry lies in its ability to handle large volumes of data quickly and accurately. This is crucial because drug discovery involves analyzing vast amounts of information from different sources such as scientific literature, electronic health records (EHRs), clinical trial results, and genetic data. With traditional methods, it can take years for researchers to gather and analyze this information manually. However, with AI-powered tools, this process can be completed within hours or days.
One key application of AI in pharmaceutical research is virtual screening – a computational approach used for identifying potential drugs based on their chemical structure’s similarity to known active compounds. This technique significantly speeds up the initial stages of drug discovery by reducing the number of compounds that need further investigation.
Moreover, AI algorithms can also aid in predicting a compound’s safety profile by analyzing its chemical properties against known toxic compounds, which could potentially reduce the time-consuming safety testing phase during clinical trials.
Benefits of using AI in drug discovery
The use of Artificial Intelligence (AI) technologies in drug discovery has revolutionized the pharmaceutical industry, offering significant benefits and advancements in research and development. AI-powered tools and techniques have greatly expedited the drug discovery process, making it more efficient, accurate, and cost-effective. Let’s take a closer look at some of the major benefits of using AI in drug discovery.
1. Accelerated Drug Discovery Process:
One of the primary advantages of incorporating AI into drug discovery is its ability to accelerate the entire process. Traditional drug discovery methods involve tedious and time-consuming steps such as target identification, lead generation, optimization, and clinical trials before a potential drug can be brought to market. With AI technologies such as machine learning algorithms and natural language processing (NLP), researchers can now analyze vast amounts of data quickly and accurately. This expedites the identification of potential targets for diseases, identifies effective compounds for synthesis, streamlines lead optimization processes, and enables faster clinical trial designs.
2. Improved Accuracy:
AI technologies offer unparalleled accuracy in analyzing vast amounts of data compared to traditional manual methods that are prone to human error. By leveraging big data analytics tools such as predictive models or simulations powered by AI algorithms, researchers can identify patterns and relationships within complex datasets that are beyond human capability.
3. Cost-Effective:
Drug development is an expensive endeavor with high failure rates due to early-stage decisions being based on insufficient data or incorrect predictions. However, by using AI-based predictive modeling and simulation techniques in preclinical phases, researchers can prioritize which compounds are most likely to succeed while reducing costs associated with failed experiments.
4.Liberalization from Geographical Constraints:
One significant benefit that AI offers in drug discovery is its ability to break down geographical barriers between researchers located around the world. With cloud-based platforms that facilitate collaboration through real-time sharing of data sets via digital devices like laptops or smartphones combined with remote access software solutions now part regular practice for research and development teams, speed and efficiency have dramatically improved. Real-time data sharing allows researchers to work together seamlessly, share ideas and learnings from across different continents.
Case studies of successful use of AI in pharmaceutical research and development
Over the past few decades, the pharmaceutical industry has faced numerous challenges in drug discovery and development. With increasingly complex diseases, rising costs, and long development timelines, there is a growing need for innovative solutions to streamline and enhance the process of bringing life-saving medications to market. Fortunately, advancements in artificial intelligence (AI) have paved the way for new possibilities in pharmaceutical research and development.
One of the most significant impacts of AI in this field is its ability to analyze vast amounts of data quickly and accurately. This has been particularly useful in drug discovery, where researchers can spend years sifting through vast libraries of compounds to find potential candidates for further study. By utilizing machine learning algorithms, AI can identify patterns and relationships within massive data sets that would be impossible for humans to detect. This significantly speeds up the drug discovery process by identifying promising compounds much earlier on.
A prime example of AI’s use in drug discovery can be seen with Exscientia’s collaboration with Japanese pharmaceutical company Sumitomo Dainippon Pharma. Through their partnership, they were able to design an entirely new molecule from scratch using AI as a key tool. The team employed deep learning algorithms to analyze massive chemical databases along with medical literature data before generating 30 potential molecules for synthesis. One of these molecules was then selected for further evaluation based on its predicted efficacy against the targeted disease – chronic obstructive pulmonary disease (COPD). This molecule completed pre-clinical testing after just seven months – a process that typically takes around four years without AI – showcasing how this technology could dramatically accelerate the early stages of drug discovery.
In addition to streamlining drug discovery, AI is also making strides in clinical research by improving patient selection criteria and optimizing trial design through predictive modeling. In one instance, Pfizer used IBM Watson’s machine learning capabilities during trials for an experimental Alzheimer’s medication called tafamidis. By analyzing millions of data points from diverse sources such as clinical trials, patient data, and scientific literature, IBM Watson was able to identify potential participants for the trial with a high likelihood of developing mild cognitive impairment or Alzheimer’s disease. This technology helped Pfizer refine its patient selection criteria and improved the probability of trial success.
Furthermore, AI is also proving to be a valuable tool in post-market surveillance and pharmacovigilance by analyzing adverse event reports in real-time. This can help pharmaceutical companies and regulatory bodies detect potential safety concerns quickly and efficiently, leading to faster interventions if needed.
Challenges and limitations of AI in the industry
While the potential of artificial intelligence (AI) in pharmaceutical research and development is immense, there are also significant challenges and limitations that need to be addressed. Despite its rapid growth and advancements, AI technology is still relatively new in the industry, which brings along certain obstacles that may impede its full utilization. In this section, we will discuss some of the main challenges and limitations faced by AI in the pharmaceutical industry.
1. Data Quality: One of the primary requirements for successful implementation of AI is access to large amounts of high-quality data. In pharmaceutical research, obtaining reliable data can be a daunting task due to strict regulations and access restrictions. Additionally, much of the available experimental data exists in unstructured formats such as images or text, making it difficult for traditional machine learning algorithms to extract useful insights.
2. Lack of Standardization: The lack of standardization in data acquisition methods can also lead to difficulties in utilizing AI effectively. Different companies may have their own proprietary databases with varying levels of accuracy and consistency, making it challenging to integrate these datasets into one comprehensive database.
3. Biased Data: Another major challenge for AI systems is biased training data. As these systems learn from historical data, any existing biases within that data will be reflected in their decision-making processes. In healthcare specifically, biased data could result in inaccurate diagnoses or treatment recommendations, which could have serious consequences for patient care.
4. Limited Interpretation Abilities: While AI excels at analyzing vast amounts of complex data quickly and accurately, its ability to explain how it arrives at a particular conclusion is limited. This makes it difficult for researchers to fully trust or understand the results produced by an AI system without additional confirmation from human experts.
5. Cost Considerations: Implementing advanced AI technologies can require significant investments- both financially and on an organizational level- which may not always be feasible for every company looking to harness its power. Furthermore, the rapid pace of technological advancements means that pharmaceutical companies need to continuously invest in AI systems and updates to remain competitive in the industry.
The future potential of AI in transforming the drug development process
The use of artificial intelligence (AI) in pharmaceutical research and development has already shown great promise in improving drug discovery and development. As technology continues to advance, the future potential of AI in transforming the entire drug development process is immense.
One major area where AI can have a significant impact is in identifying new drug targets. Traditional methods of target identification rely on years of laborious experimentation and complex data analysis. However, with the help of machine learning algorithms, AI can efficiently analyze vast amounts of data from various sources such as genetic databases, scientific literature, clinical trials, and real-world patient data to identify potential drug targets.
In addition to target identification, AI can also play a crucial role in streamlining the preclinical stage of drug development. Currently, this stage involves extensive testing on animals and takes up a significant portion of time and resources. By using predictive models developed through machine learning techniques on existing data sets from previous studies, AI can help predict how certain drugs will behave in preclinical studies. This increases the chances of selecting successful candidates for further development while reducing costs and animal usage.
AI’s impact extends even further into clinical trials by helping researchers design more efficient and effective studies. One way it does this is through patient selection criteria optimization. AI-powered analytics can identify patterns within large datasets to predict which patients are most likely to respond positively to treatment or experience adverse events based on their unique characteristics such as genetics, lifestyle factors, or medical history. This not only speeds up the recruitment process but also reduces costs associated with failed trials due to inappropriate participant selection.
Moreover, during clinical trials, AI systems can continuously monitor patients’ response to treatment and detect any potential safety concerns early on. In doing so, it allows researchers to make necessary adjustments quickly before entering later phases or seeking regulatory approval.
Interestingly, AI could also revolutionize post-marketing surveillance by analyzing real-world data from electronic health records (EHRs). By leveraging natural language processing (NLP) and data mining techniques, AI can identify post-market safety signals that would have otherwise gone unnoticed. This could help improve patient safety and enable faster response to potential adverse events.
Ethical considerations surrounding the use of AI in pharmaceutical research
Ethical considerations surrounding the use of AI in pharmaceutical research are crucial to address as the field continues to evolve and advance. The potential uses of AI in pharmaceutical research are vast; from drug discovery and development, to clinical trials and post-market surveillance, AI has already shown promising results in improving efficiency, accuracy, and cost effectiveness. However, with great power comes great responsibility.
One of the primary ethical concerns surrounding the use of AI in pharmaceutical research is the potential for bias. As AI systems rely on data inputs to make decisions and predictions, there is a risk that existing biases within the data or programming could be amplified. For example, if a dataset used to train an AI system is skewed towards certain demographics or excludes marginalized populations, the resulting algorithm may not accurately represent all patient populations. This can lead to disparities in healthcare outcomes and perpetuate systemic inequalities.
Another ethical consideration is privacy protection. In order for AI systems to function effectively, they require access to large amounts of personal health data. This raises questions about who has access to this sensitive information and how it will be used or potentially shared with third parties. It also brings up concerns about data security and protecting against cyber attacks that could compromise patient privacy.
Transparency is another important aspect when it comes to using AI in pharmaceutical research. As these systems often involve complex algorithms developed by machine learning techniques, their decision-making processes may not always be easily explainable or understandable by humans. This lack of transparency can raise concerns among patients and regulatory bodies about trusting these systems with making critical decisions related to their health.
Furthermore, there are ethical implications around the potential job displacement caused by increased use of AI in pharmaceutical research. With automation becoming more prevalent throughout many industries including healthcare, there is a possibility that some jobs traditionally held by humans will become obsolete as machines are able to perform tasks faster and more efficiently.
To navigate through these ethical considerations surrounding AI in pharmaceutical research, several guidelines have been developed such as the Ethical Guidelines for Trustworthy AI by the European Commission. These guidelines aim to ensure that AI systems are designed and used in a responsible, fair, and transparent manner.
Conclusion
As we move into a more technologically advanced era, the use of artificial intelligence in pharmaceutical research and development is becoming increasingly important. It has the potential to greatly improve not only the speed and accuracy of drug discovery but also patient outcomes. With continued advancements in AI technology, we can expect to see even greater strides made in this field in the future. By harnessing the power of AI, we have an opportunity to revolutionize medicine and make significant progress towards finding cures for diseases that continue to affect millions around the world. The possibilities are endless, and with proper regulation and ethical considerations, we can truly unlock the full potential of AI in pharmaceutical R&D.
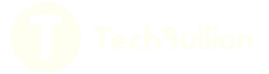