In the exciting world of pharmaceuticals, cutting-edge technologies are revolutionizing the way new drugs are discovered and developed. One such game-changer is machine learning, a powerful tool that is transforming the landscape of drug development. Join us as we delve into the fascinating realm of using machine learning in drug development and uncover how this innovative approach is paving the way for groundbreaking discoveries in medicine.
Introduction to Machine Learning and Drug Development
Machine learning (ML) has revolutionized various industries, including drug development. ML involves the use of algorithms and statistical models to enable machines to learn from data and make predictions or decisions without explicit programming. In recent years, there has been a significant increase in the use of ML techniques in drug development due to their ability to accelerate the process, reduce costs, and improve efficacy.
One of the major challenges in traditional drug development is identifying potential therapeutic targets, which are specific molecules or proteins involved in diseases. This process requires extensive knowledge about disease mechanisms and complex interactions between different biological components. However, with the help of ML, this task can be streamlined by analyzing large amounts of data from various sources such as genomic sequencing, clinical trials, and electronic health records.
ML also plays an important role in drug discovery by helping researchers identify new compounds that could potentially serve as drugs. Traditional methods for this involve screening millions of chemical compounds manually, which is a time-consuming and labor-intensive process. With ML techniques such as deep learning and natural language processing, scientists can quickly analyze vast quantities of chemical data to identify promising candidates for further testing.
In addition to target identification and compound screening, ML is also being used in clinical trials. Clinical trials are essential for evaluating the safety and efficacy of new drugs before they can be approved for use by regulatory agencies. However, these trials are often costly and time-consuming due to strict regulations and guidelines. By utilizing predictive modeling algorithms that incorporate patient data from previous trials, researchers can better design efficient clinical trials with a higher chance of success.
Another area where machine learning is having a significant impact on drug development is personalized medicine. Personalized medicine involves tailoring treatments based on an individual’s genetic makeup or other factors specific to their condition rather than using a one-size-fits-all approach. With the help of ML techniques like predictive analytics and precision medicine platforms, researchers can identify patient subgroups and tailor treatments to their individual needs, resulting in more effective and personalized care.
How AI is Changing the Landscape of Drug Discovery
Over the years, drug discovery and development have been time-consuming, expensive, and unpredictable processes. Traditional methods of identifying new drugs involve trial and error experiments, which can take years to yield results. However, with advancements in technology and the rise of artificial intelligence (AI), the landscape of drug discovery is rapidly changing.
One of the key ways AI is transforming drug discovery is through its ability to analyze large amounts of data. With the help of machine learning algorithms, researchers can now sift through massive datasets containing information about potential drugs, diseases, and genetic variations. This allows them to identify patterns and relationships that may not be easily recognizable by human analysis alone. As a result, AI-powered platforms are able to identify potential candidates for drug development more efficiently than ever before.
Another major impact of AI on drug discovery is in virtual screening or computer-aided design (CAD) techniques. These methods use computer simulations to predict how a particular molecule will interact with its target protein or enzyme in the body. By virtually screening thousands or even millions of molecules, researchers can narrow down potential candidates for experimental testing with much greater accuracy than traditional methods.
Moreover, AI has also played an essential role in accelerating the process of clinical trials. In traditional drug development processes, identifying suitable participants for clinical trials was often a time-consuming and challenging task. However, with AI-powered tools such as predictive analytics and natural language processing (NLP), researchers can quickly sift through vast amounts of patient data to find suitable candidates who meet specific criteria for trials.
In addition to these benefits, AI has also opened up new possibilities for personalized medicine by enabling precision medicine approaches. With access to vast stores of genetic data from patients around the world combined with machine learning algorithms that can interpret this data quickly and accurately; scientists are now able to develop treatments tailored specifically towards individual patients based on their genetic makeup.
Despite all these advantages brought by AI in drug discovery; concerns regarding ethical and regulatory issues have also been raised. For instance, there are concerns about the transparency of AI algorithms and potential bias in datasets. There is also a need for ethical guidelines to be established to ensure the responsible use of AI in drug development.
Advantages of Using Machine Learning in Drug Development
Machine learning (ML) is a rapidly developing field that has shown immense potential in various industries, including healthcare and medicine. Its ability to analyze large amounts of data, find patterns and make predictions has opened up new possibilities in the drug development process. In this section, we will explore some of the advantages of using machine learning in drug development.
1. Improved Efficiency and Speed
One of the most significant advantages of incorporating ML into drug development is its capability to improve efficiency and speed up the process. Traditional methods of drug discovery involve time-consuming experiments and trial-and-error approaches, which can take years to yield results. However, with ML, researchers can quickly analyze vast amounts of data from various sources like genes, proteins, and chemical compounds to identify potential targets for drug development. This reduces the time required to identify potential candidates for further testing significantly.
2. Enhanced Predictive Capabilities
Machine learning algorithms have an incredible ability to learn from past data and make accurate predictions about future outcomes. In the context of drug development, this means that ML can help identify potentially effective drugs much earlier in the process than traditional methods. By analyzing existing drugs’ chemical structures and their effects on diseases at a molecular level, machine learning models can predict which compounds are likely to be successful without extensive experimentation.
3. Cost Savings
In addition to improving efficiency and speed in drug development, ML also has the potential to save costs significantly. With traditional methods, researchers must screen thousands or even millions of compounds before finding a suitable candidate for further testing – a costly endeavor involving high labor costs and expensive laboratory equipment. On the other hand, machine learning algorithms can sort through enormous volumes of data relatively inexpensively since they do not require physical resources or excessive manpower.
4. Identification of Potential Side Effects
Another advantage that ML brings to drug development is its ability to predict harmful side effects more accurately. Before any medication makes it onto pharmacy shelves worldwide use; it must undergo rigorous testing for safety and efficacy. However, even with stringent testing processes in place, some drugs have caused unforeseen side effects when introduced to the general population. With machine learning, researchers can analyze data from clinical trials to identify patterns that may indicate potential side effects and make necessary adjustments before moving on to human trials.
Case Studies: Successful Implementation of ML in Drug Development
Machine learning (ML) is a rapidly evolving technology that has shown great potential in various industries, including drug development. Its ability to analyze large amounts of data and make predictions based on patterns and trends has made it an invaluable tool for pharmaceutical companies in streamlining their drug discovery and development processes.
Over the years, several case studies have demonstrated the successful implementation of ML in different stages of drug development. This section will explore three noteworthy examples that highlight the impact of ML in this field.
1) Drug Repurposing: In 2018, a team led by researchers at Pfizer developed a novel ML algorithm called Deep Purpose (DeepPU) with the aim of identifying new therapeutic uses for existing drugs. The algorithm was trained on over 6 million data points from biological databases and was able to predict novel protein interactions with high accuracy. As a result, DeepPU successfully identified two potential new indications for an existing breast cancer drug, palbociclib. This approach not only saves time and resources spent on developing new drugs but also provides faster access to potentially life-saving treatments for patients.
2) Optimizing Clinical Trials: Clinical trials are an essential part of drug development but can be lengthy and expensive. In 2019, Roche announced their collaboration with Britain’s Oxford University to develop ML algorithms that could help identify potential participants for clinical trials based on electronic health records. The algorithm incorporates factors such as age, sex, medical history, lab results, etc., to match suitable patients with specific trial criteria accurately. This approach has proven to accelerate patient recruitment while reducing costs and increasing efficiency in clinical trials.
3) Predicting Drug Safety: One major challenge in drug development is ensuring the safety profile of a compound before it reaches clinical trials. In 2020, AstraZeneca partnered with Google’s DeepMind Technologies to develop an AI-based system named AlphaFoldTM that can accurately predict protein structures from amino acid sequences. This technology has the potential to predict off-target interactions, which could lead to adverse side effects, thereby optimizing drug design and potentially reducing the risk of harm to patients.
Challenges and Limitations of Machine Learning in Drug Discovery
The use of machine learning (ML) in drug discovery has shown great promise and potential to revolutionize the traditional process. However, like any other technology, it also comes with its fair share of challenges and limitations that need to be addressed for it to reach its full potential.
One major challenge of utilizing ML in drug discovery is the availability and quality of data. ML algorithms rely heavily on high-quality data to make accurate predictions. In the case of drug development, this means having access to comprehensive and well-curated databases that contain information about various compounds, targets, and disease targets. Unfortunately, these types of databases are often limited or inaccessible due to several reasons such as privacy concerns or proprietary restrictions. This poses a significant hurdle for researchers trying to develop ML models for drug discovery.
Another critical limitation is the black box nature of some ML algorithms. Unlike traditional methods where scientists have a clear understanding of the underlying principles, some ML approaches can produce results without providing an explanation or reasoning behind them. This can be a major drawback in drug discovery since understanding how a particular compound interacts with a target is crucial in determining its effectiveness and safety profile. The lack of interpretability can make it challenging for researchers to trust and validate the predictions made by ML models.
Furthermore, there is also the issue of bias in data used for training ML models, which can lead to biased results. Since most datasets used in drug discovery are created from previous research studies, they may already contain implicit biases that can affect the accuracy and generalizability of ML models. For example,
an algorithm trained on historical data that predominantly represents one demographic group may not perform well when used on another group with different genetic backgrounds.
Additionally, implementing an effective regulatory framework for using ML in drug development remains a challenge as regulatory bodies struggle to keep up with advancements in technology. The lack of standardized guidelines makes it difficult to assess whether an ML model is performing correctly or ethically.
Ethical Considerations Surrounding the Use of AI in Healthcare
The use of Artificial Intelligence (AI) in healthcare is becoming increasingly common, with many researchers and pharmaceutical companies turning to machine learning to aid in drug development. While AI has the potential to revolutionize the field of medicine and improve patient outcomes, there are also ethical considerations that must be addressed when implementing these technologies.
One of the main concerns surrounding AI in healthcare is data privacy and protection. Machine learning algorithms rely heavily on large datasets to train and improve their performance. In drug development, this means using sensitive patient information from electronic health records, genetic testing, and clinical trials. This raises questions about who owns this data and how it can be used ethically. With the growing number of cybersecurity threats, ensuring that patient data is protected becomes a top priority for both healthcare providers and pharmaceutical companies.
Another ethical consideration is the potential for bias within AI systems. Machine learning algorithms are only as unbiased as the data they are trained on. If historical or biased data is used, it can perpetuate existing inequalities, leading to unequal access to treatments or misdiagnoses for certain populations. It is crucial for developers to actively address these biases by reviewing and diversifying their training data sets.
Transparency and explainability are also crucial elements when considering AI in healthcare. As machine learning models become more complex, it becomes difficult for humans to understand their decision-making process. In healthcare, where people’s lives are at stake, it is essential to have explanations for why a particular treatment plan or diagnosis was chosen by an algorithm.
Additionally, there needs to be accountability when developing and deploying AI systems in healthcare. There should be clear guidelines on who should be responsible if something goes wrong due to an AI’s recommendation or decision-making process. Developers must also ensure that there are proper safeguards in place if an algorithm makes a mistake or learns incorrect patterns from its training data.
Issues related to trust need to be considered when implementing AI in healthcare settings. Patients and healthcare professionals must have confidence in the technology being used and understand its limitations. This requires effective communication and transparency from developers to build trust in AI systems.
Future Possibilities for AI in the Field of Drug Development
The role of artificial intelligence (AI) in drug development is rapidly evolving, and the possibilities for its application are endless. By utilizing machine learning algorithms, researchers and pharmaceutical companies can accelerate the drug discovery process, reduce costs, and ultimately bring more effective treatments to market.
One of the most significant potential uses for AI in drug development is optimizing target identification and validation. With traditional methods, identifying a viable drug target can take years of research and experimentation. However, with the use of machine learning techniques such as natural language processing and deep learning, vast amounts of data from various sources can be quickly analyzed to identify potential targets for specific diseases. This not only speeds up the process but also increases the chances of finding successful targets that may have been missed using traditional methods.
Another area where AI could revolutionize drug development is in lead optimization. This stage involves refining a compound’s chemical structure to make it more effective against a particular disease while minimizing unwanted side effects. Traditionally, this has been a time-consuming process that requires numerous rounds of trial and error. With AI-powered tools like predictive modeling and virtual screening, scientists can analyze massive datasets to predict how changes in a molecule’s structure will impact its effectiveness against a particular disease. This allows for faster identification of promising compounds that can then be further developed for clinical trials.
Furthermore, machine learning could play an essential role in predicting possible adverse reactions before drugs enter clinical trials. Currently, adverse reactions are often discovered during these trials or even after drugs have been approved by regulatory bodies – leading to costly setbacks or recalls. By analyzing data from past clinical trials and real-world patient information through advanced statistical models, AI algorithms could identify patterns that indicate potential harm before it occurs.
In addition to streamlining different stages of drug development processes, there is also exciting potential for AI utilization in personalized medicine – tailoring treatments based on an individual’s genetic makeup or medical history. With advances in genomic sequencing technology producing vast amounts of data, AI algorithms can analyze and identify connections between genetic mutations and diseases. This could lead to the development of more precise, targeted treatments for patients based on their unique genetic profile.
Conclusion
As the demand for new and effective drugs continues to rise, the use of machine learning in drug development has become increasingly prevalent. With its ability to analyze vast amounts of data and identify patterns, machine learning is revolutionizing the way drugs are discovered, developed, and tested. The potential benefits are immense – from reducing time and costs to producing more targeted and personalized medications. As we continue to explore this exciting field, it is clear that machine learning will play a crucial role in shaping the future of drug discovery and ultimately improving human health.
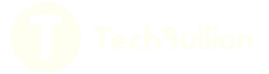