Generative AI: Redefining the Technology Landscape
Generative AI has advanced rapidly, with its capabilities now embedded in tools used for text creation, image generation, code development, and even music composition. Enterprises are increasingly investing in generative ai services to innovate processes, improve customer experience, and unlock new revenue streams. According to McKinsey’s 2024 report, generative AI could add between $2.6 trillion to $4.4 trillion in annual global productivity.
As businesses and developers look ahead, several trends are emerging that signal the future direction of generative AI trends that span ethics, multi-modal models, industry-specific adoption, and real-time capabilities.
1. Rise of Multimodal Generative AI Models
Initially focused on text-based outputs, generative AI has evolved to process and produce multiple content types simultaneously. Multimodal models, such as OpenAI’s GPT-4 and Google’s Gemini, combine text, image, audio, and video processing into one unified framework.
These models enable complex use cases like:
- Automated video generation from written prompts
- Medical diagnostics combining imaging and patient history
- Virtual customer assistants that handle voice, text, and facial cues
This advancement is paving the way for broader adoption across creative industries, healthcare, education, and e-commerce, each of which now explores generative ai services that are more immersive and context-aware.
2. Industry-Specific AI Customization
Another key trend is the fine-tuning of generative AI models for specific industries. Generic, out-of-the-box models often fall short when precision, compliance, or technical language is required. As a result, companies are increasingly building domain-specific solutions to address unique challenges.
Examples include:
- Financial firms deploying AI for regulatory reporting and fraud detection
- Retailers generating hyper-personalized marketing content and product visuals
- Manufacturing companies using generative design for prototyping
A 2024 Deloitte survey found that 59% of enterprises using generative AI plan to invest in domain-specific models over the next 12 months, indicating a shift toward tailored applications rather than broad, generalized tools.
3. Focus on Responsible and Explainable AI
As generative AI systems are deployed at scale, concerns about ethical usage, content accuracy, and bias have intensified. There is a growing emphasis on transparency, auditability, and responsible AI development.
Key developments include:
- Introduction of AI ethics regulations in the EU and the U.S.
- Model interpretability tools that explain AI decisions in human terms
- Built-in safeguards to prevent misinformation or harmful content generation
Organizations are working closely with generative ai services providers to build governance frameworks that address compliance, ethical standards, and risk management. These measures are especially critical in sensitive sectors like legal, healthcare, and public services.
4. Real-Time Generative Capabilities
As hardware and software infrastructure improve, real-time generative AI applications are gaining traction. From generating music live during performances to adapting video game narratives on the fly, latency-sensitive solutions are now viable.
In customer service, for instance, AI agents can provide instant replies with human-like fluency, while also understanding sentiment and context. In manufacturing, real-time generative models are being used for predictive maintenance and adaptive design.
A recent IDC report projected that by 2026, 40% of generative AI deployments in enterprises will be real-time, delivering immediate outputs with dynamic adaptability. This marks a shift from batch processing to interactive, real-world engagement.
5. Integration with IoT and Edge Devices
Generative AI is not limited to cloud environments anymore. With advancements in edge computing and AI chips, models are now running on smaller devices, enabling applications in autonomous vehicles, smart appliances, and AR/VR headsets.
This edge deployment allows:
- Faster decision-making with reduced latency
- Greater data privacy, as sensitive information doesn’t leave the device
- Context-rich personalization, adapting to real-time user inputs
By combining generative ai solutions with IoT infrastructure, businesses can design connected ecosystems that respond intelligently and efficiently to environmental cues.
6. Data Efficiency and Synthetic Data Generation
Training large AI models requires massive datasets, but collecting and annotating high-quality data can be costly and time-consuming. Generative AI itself is now being used to generate synthetic datasets that are statistically representative of real-world data—boosting efficiency while addressing privacy challenges.
In healthcare, for example, synthetic data can be used for training diagnostic algorithms without exposing patient records. In finance, it helps model fraud patterns without using actual client transactions.
Gartner predicts that by 2025, 60% of the data used for AI development will be synthetically generated. This approach is expected to accelerate AI innovation, especially for smaller organizations with limited access to proprietary data.
7. Democratization of AI Development
Low-code and no-code AI platforms are making it easier for non-technical users to build generative AI applications. With drag-and-drop interfaces and pre-trained models, professionals in marketing, HR, and design can leverage generative capabilities without writing complex code.
This democratization is:
- Reducing time-to-market for AI tools
- Enabling creative experimentation across teams
- Expanding AI literacy beyond technical roles
According to a 2024 Salesforce report, 52% of non-developers across enterprises now engage with AI-powered tools in their daily work—indicating a broad cultural shift in how AI is perceived and used.
Conclusion
Generative AI is transitioning from a promising innovation to a foundational part of business strategy. As multimodal capabilities grow, real-time applications emerge, and models become industry-specific, the impact of this technology will be felt across every major sector.
Organizations that understand and adapt to these trends will lead the next wave of AI-driven transformation. Partnering with the right technology vendors, investing in ethical practices, and embracing a data-centric approach will be essential to harness the full potential of generative AI.
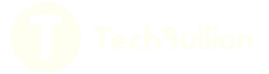