Introduction:
Are you intrigued by the buzz around data science but feel like it’s a complex and mysterious world that only experts can unravel? Well, we’re here to demystify it for you! Welcome to our beginner’s guide on data science specifically designed for investors. In this article, we will break down the fundamentals of data science in plain English, help you understand its significance in investment decisions, and explore how it can empower your financial strategies like never before. So fasten your seatbelts as we embark on an exciting journey into the world of data-driven investing!
What is Data Science?
Data science is a rapidly growing field that has gained significant attention in recent years. However, despite its increasing popularity, there is still a lot of confusion surrounding what exactly data science is and what it entails. In this section, we will provide a clear understanding of its definition and purpose.
At its core, data science is an interdisciplinary field that combines statistics, computer science, and domain expertise to extract meaningful insights from large amounts of complex data. It involves using various techniques such as predictive modeling, machine learning algorithms, data mining, and visualization to uncover patterns and trends in data.
One of the key aspects of data science is the use of programming languages like Python or R to manipulate and analyze vast datasets. These languages allow data scientists to clean and preprocess raw data before applying advanced analytical methods to gain valuable insights.
Another essential component of data science is statistical analysis. Data scientists utilize statistical techniques such as regression analysis, hypothesis testing, and clustering to make sense of the massive amount of information available. This allows them to identify relationships between variables and make predictions about future outcomes based on past patterns.
Furthermore, machine learning plays a crucial role in the field of data science. It involves using algorithms that can learn from historical data without being explicitly programmed for specific tasks. Machine learning enables businesses to automate processes such as fraud detection or customer segmentation by training models on large volumes of historical records.
Apart from technical skills like programming and statistics, domain expertise is also an essential aspect of data science.
Why is Data Science Important for Investors?
Data science has become an integral part of the investment world, and for good reason. In today’s fast-paced and data-driven market, it is crucial for investors to understand the importance of data science and how it can help them make more informed decisions.
Here are some key reasons why data science is essential for investors:
1. Data-Driven Decision Making:
Investing involves analyzing vast amounts of data such as financial reports, economic indicators, market trends, and consumer behavior. With the help of data science techniques such as machine learning and predictive analytics, investors can not only process large datasets quickly but also identify patterns and make more accurate predictions. This reduces the risk of making decisions based on gut feelings or incomplete information.
2. Identifying Opportunities:
One of the primary objectives of investing is to identify profitable opportunities in the market. Data science enables investors to analyze historical data and identify emerging trends that could lead to potential investment opportunities. By leveraging advanced algorithms, investors can also spot hidden patterns in complex datasets that may have gone unnoticed otherwise.
3. Risk Management:
Every investment carries a certain amount of risk, and managing that risk is critical for success in the market. Data science plays a crucial role in risk management by providing insights into various factors such as volatility, correlation between assets, and portfolio diversification strategies. This helps investors make more informed decisions about their investments, leading to better risk-adjusted returns.
4. Automating Investment Process:
Data Science has revolutionized investing by automating several processes that were traditionally time-consuming and prone to human error. For example, algorithmic trading relies heavily on data science techniques to execute high-frequency trades and optimize trading strategies automatically.
Common Misconceptions about Data Science
There are many misconceptions surrounding the field of data science, which can often lead to confusion and misinterpretation. In this section, we will address some of the most common misconceptions about data science and provide a clear understanding of what it truly entails.
1. Data science is all about coding and programming: One of the biggest misconceptions about data science is that it only involves coding and programming. While these skills are certainly important in the field, they are not the only ones required. Data scientists also need strong mathematical and statistical skills to analyze and interpret large amounts of data effectively. Additionally, communication skills are crucial for presenting findings to non-technical stakeholders.
2. Data science is only for tech companies: Another misconception is that data science is only relevant in tech companies or industries such as finance or healthcare. The truth is that almost every industry can benefit from utilizing data science techniques to gain valuable insights from their data. From retail to manufacturing, data plays a significant role in decision-making processes across various sectors.
3. You need a degree in computer science or mathematics to become a data scientist: While having a degree in computer science or mathematics may give you an advantage when pursuing a career in data science, it is not always necessary. Many successful data scientists come from diverse backgrounds such as economics, psychology, or business management. What matters more is having a strong aptitude for problem-solving and critical thinking.
Key Components of Data Science
Data science is a rapidly growing field that has become increasingly important for businesses and investors alike. It involves the use of various techniques, tools, and algorithms to extract valuable insights from large amounts of data. In this section, we will explore some of the key components of data science that are essential for understanding this complex field.
1. Statistics:
At its core, data science is about analyzing and interpreting data to make informed decisions. This requires a strong foundation in statistics, which enables data scientists to identify patterns and trends in the data. Understanding concepts such as probability, hypothesis testing, regression analysis, and sampling methods is crucial for accurate data analysis.
2. Programming:
Data scientists need to have a solid understanding of programming languages such as Python or R to work with large datasets efficiently. These languages allow them to clean, manipulate, and transform raw data into meaningful insights. Additionally, knowledge of SQL (Structured Query Language) is also beneficial as it helps in retrieving specific information from databases.
3. Machine Learning:
Machine learning (ML) is a subset of artificial intelligence (AI) that focuses on developing algorithms capable of learning from data without being explicitly programmed. ML techniques such as supervised learning (classification and regression), unsupervised learning (clustering), and reinforcement learning are widely used in predictive modeling and decision-making processes.
4. Data Visualization:
Communicating insights effectively is an essential aspect of any successful business strategy. Data visualization tools like Tableau or Power BI enable data scientists to create interactive visualizations that help convey complex information in a clear and understandable manner. Visualizations are valuable for presenting findings to stakeholders and making data-driven recommendations.
How to Analyze and Interpret Data as an Investor
As an investor, making informed decisions is crucial for success in the stock market. However, with the vast amount of data available, it can be overwhelming to know where to start. This is where data analysis and interpretation come into play. By understanding how to analyze and interpret data as an investor, you can gain valuable insights that can guide your investment decisions.
Here are some key steps on how to effectively analyze and interpret data as an investor:
1. Define Your Objectives:
The first step in analyzing and interpreting data as an investor is to define your objectives. What do you want to achieve through your investments? Are you looking for long-term growth or short-term gains? Understanding your goals will help determine what type of data you need to focus on and what metrics are most important for your investment strategy.
2. Identify Relevant Data Sources:
Once you have defined your objectives, the next step is to identify relevant data sources. It’s essential to gather a diverse range of data from reputable sources to get a well-rounded view of the market.
3. Clean and Organize Data:
Before delving into analysis, it’s crucial to clean and organize the data properly. This involves removing any irrelevant or duplicated information and organizing it in a way that makes sense for your objectives.
4. Utilize Data Visualization Techniques:
Data visualization is a powerful tool for investors. It allows you to create visual representations of your data, such as charts and graphs, which can help you identify trends and patterns more easily. Tools like Excel, Tableau, or Google Data Studio can assist you in creating meaningful visualizations.
5. Develop a Data-Driven Strategy:
Based on your analysis and interpretation of data, develop a data-driven investment strategy. This strategy should align with your objectives and risk tolerance. It may involve diversifying your portfolio, adjusting your asset allocation, or making specific investment decisions based on your findings.
6. Seek Expert Advice:
If you’re unsure about data analysis or interpretation, consider seeking advice from financial experts or hiring a financial advisor. They can provide valuable insights and guidance to help you make informed investment decisions.
Conclusion:
In today’s data-driven world, investors have access to a wealth of information that can be harnessed to make more informed decisions. Analyzing and interpreting data is a valuable skill for investors, enabling them to identify opportunities, manage risks, and optimize their investment strategies. By following the steps outlined above and staying informed about market developments, investors can leverage the power of data to achieve their financial goals.
Read More
The Future of Data Science: Trends and Predictions
Exploring Algorithms, Models, and Visualization Techniques Of Data Science
Data Science vs. Artificial Intelligence: Understanding the Distinctions
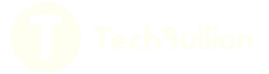