Welcome to the world of data science, where numbers come alive and algorithms drive innovation! As technology continues to advance at an exponential rate, so does our ability to harness the power of data. From uncovering hidden patterns to making accurate predictions, data scientists have become modern-day wizards transforming industries in unimaginable ways. So what lies ahead for this dynamic field? Join us as we take a deep dive into the future of data science, exploring the latest trends and daring predictions that will shape our world in mind-blowing ways. Strap in, because this is going to be one exhilarating journey filled with groundbreaking discoveries and jaw-dropping insights you won’t want to miss!
Introduction to Data Science
Data science, also known as data-driven science, is an interdisciplinary field that combines scientific methods, algorithms, and systems to extract knowledge and insights from structured or unstructured data. It encompasses a wide range of techniques and tools including statistics, mathematics, programming languages like R and Python, machine learning algorithms, and data visualization.
The explosive growth of digital information has led to the rise in demand for skilled professionals who can analyze vast amounts of data and make meaningful conclusions. This is where the role of data scientists comes in. They are trained individuals with a blend of technical skills and business acumen who can effectively collect, clean, organize, analyze and interpret large datasets.
One important aspect to note is that data science not only focuses on extracting insights from existing data but also on generating new datasets through various experiments and simulations. It enables organizations to make informed decisions by identifying patterns and trends within their data.
Applications of Data Science
Data science has numerous applications across various industries such as healthcare, finance, marketing, retail, manufacturing etc. For instance:
1) In healthcare – Data scientists use electronic health records (EHRs) to improve patient outcomes by identifying risk factors for diseases or predicting epidemics.
2) In finance – Data scientists use predictive models to anticipate market trends or fraud detection.
3) In marketing – Big companies use customer’s purchase behavior to personalize advertisements.
4) In retail – Retailers analyze sales figures combined with demographic information to optimize store layouts.
Why Data Science is Important for the Future?
The importance of data science in the future cannot be overstated. With the exponential growth of data and technological advancements, data science has emerged as a crucial field that is driving innovation, decision-making processes, and shaping the future.
1. Driving Innovation:
Data science is at the forefront of driving innovation in various industries such as healthcare, finance, transportation, and marketing. By analyzing vast amounts of data collected from different sources, it enables companies to identify patterns, trends, and insights that can lead to innovative ideas and solutions. Machine learning algorithms used in data science also enhance automation and optimization processes that further drive innovation.
2. Artificial Intelligence (AI) Development:
As AI continues to evolve and encompass various aspects of our lives, data science plays a critical role in developing intelligent systems. Data scientists use machine learning algorithms to train AI models using massive datasets to make predictions and decisions similar to humans. In the future, the demand for AI-driven technology will continue to rise exponentially; thus, making the role of data science even more important.
3. Making Informed Decisions:
In today’s fast-paced business world, making informed decisions based on accurate data is vital for success. Data scientists help organizations by collecting relevant information from multiple sources; they then clean, organize and analyze this information to provide valuable insights that enable better decision-making processes.
4. Predictive Analytics:
One significant aspect of data science is its ability to predict trends or anticipate outcomes based on historical patterns within vast datasets. Predictive analytics allows companies to foresee potential risks, identify opportunities and make strategic decisions accordingly. It also helps in minimizing risks and maximizing profits.
5. Personalization:
Data science has revolutionized the way companies personalize customer experiences. By analyzing customer behavior and preferences, companies can provide personalized recommendations, advertisements, and products tailored to individual needs. This level of personalization enhances customer satisfaction and loyalty, making it an essential aspect of business strategy.
6. Big Data Management:
With the rapid growth of data, traditional methods of data management are becoming obsolete. Data science provides efficient techniques for collecting, storing, and managing large datasets. It enables organizations to process massive amounts of data quickly and accurately; thus, improving overall efficiency.
Current Trends in Data Science
Data science is a rapidly evolving field, and staying updated on the latest trends is crucial for both professionals and individuals interested in this area. Today, we will explore some of the current trends in data science that are shaping the future of this discipline.
1. Increased Focus on Data Ethics
With the increasing use of data in every aspect of our lives, there has been a growing concern about privacy and ethics. As a result, companies and organizations are now placing more emphasis on ethical principles when it comes to collecting, analyzing, and using data. This includes ensuring transparency, informed consent from individuals whose data is being collected, and responsible handling of sensitive information.
2. Artificial Intelligence (AI) Integration
AI has become an integral part of many industries with its ability to analyze large amounts of data quickly and accurately. In the field of data science, AI technologies such as machine learning are being used to develop predictive models that can make accurate forecasts based on historical data patterns. With advancements in Natural Language Processing (NLP) technology, AI is also being used to process unstructured text data such as social media posts or customer reviews.
3. The Emergence of Edge Computing
Edge computing refers to processing data closer to where it is generated rather than sending it to a central location for analysis. This trend has gained traction due to the enormous amount of data produced by connected devices and sensors (known as the Internet of Things or IoT). By leveraging edge computing techniques like distributed computing, businesses can reduce latency issues while improving the speed, security, and efficiency of data processing.
4. Democratization of Data Science
Data science used to be a specialized field accessible only to those with advanced degrees in computer science or statistics. However, with the proliferation of user-friendly data science tools and platforms, individuals from various backgrounds can now handle and analyze data without extensive technical knowledge. This trend is known as the democratization of data science, and it is allowing companies to make better use of data by involving more stakeholders in decision-making processes.
5. Augmented Analytics
Augmented analytics combines AI, machine learning, and natural language processing to automate the process of preparing and analyzing large datasets. By automating tasks like data preparation, augmented analytics saves time for data scientists and allows them to focus on more complex analysis. It also enables business users without technical skills to leverage data for decision-making.
6. Graph Analytics
Graph analytics is a technique used for analyzing connected data networks represented by nodes (entities) and edges (relationships). It helps uncover patterns and insights that traditional methods may miss due to its ability to handle complex relationships between data points. Businesses are using this approach to understand customer behavior, detect fraudulent activities, or optimize their supply chain networks.
Emerging Technologies in Data Science
Data science is an ever-evolving field, and with technological advancements being made every day, the future of this discipline looks promising and exciting. With the amount of data generated globally increasing exponentially, it is becoming more crucial to find new and innovative ways to analyze and extract meaningful insights from this data. This has led to the emergence of several new technologies in data science that are shaping the future landscape of the field.
In this section, we will explore some of the most significant emerging technologies in data science that are expected to have a significant impact on its growth and development in the coming years.
1. Artificial Intelligence (AI)
Artificial Intelligence has become one of the hottest buzzwords in recent years, and for good reason. AI enables machines to learn from experience, adapt to new situations and perform tasks that traditionally required human intelligence. Data scientists can use AI-powered tools such as machine learning algorithms for predictive analytics, natural language processing for text analysis, computer vision for image recognition, and many other applications in areas like healthcare, finance, marketing, etc. With advances in deep learning techniques enabling more accurate predictions and decision-making abilities based on complex data sets, AI is undoubtedly one of the most talked-about emerging technologies in data science.
2. Internet-of-Things (IoT)
One buzzword that goes hand-in-hand with AI is IoT (Internet-of-Things). It refers to a network of interconnected devices that collect valuable real-time data from various sources such as sensors embedded in machines or even everyday objects like cars, appliances, etc. IoT data presents a massive potential for analysis and insights for businesses in various sectors including healthcare, manufacturing, retail, and more. Data scientists can use IoT data to improve efficiency, optimize processes, predict maintenance needs, and even prevent failures.
3. Blockchain
Blockchain technology has garnered a lot of attention as the underlying technology powering cryptocurrencies like Bitcoin. But its potential application in data science is also significant. The distributed ledger system of blockchain provides a secure and transparent record of transactions that cannot be altered or deleted. This attribute can solve many challenges faced by data scientists such as maintaining the integrity and trustworthiness of large datasets. It also enables the sharing of data between multiple parties without compromising privacy or security.
4. Edge Computing
With the rise of IoT devices generating vast amounts of data, traditional centralized cloud computing systems face challenges in processing and storing this data effectively. This is where Edge Computing comes into play – it allows for processing and analyzing data closer to its source rather than sending it to a central cloud server for analysis. This reduces network latency, makes real-time decision-making possible while reducing costs associated with cloud computing.
Predictions for the Future of Data Science
As data science continues to rapidly evolve, the future of this field is filled with endless possibilities. In this section, we will explore some of the key predictions for the future of data science and how it is expected to shape various industries and our daily lives.
1) Increased Automation and Integration:
One major prediction for the future of data science is an increase in automation and integration. With advancements in artificial intelligence (AI), machine learning, and deep learning, we can expect to see more automated processes in data science workflows. This will not only enhance efficiency but also enable businesses to make faster and more accurate decisions.
Moreover, we can also expect further integration of data science with other fields such as finance, healthcare, marketing, and retail. As data becomes increasingly important across all industries, the integration of different sources will be crucial for deriving valuable insights.
2) Growth in Predictive Analytics:
Predictive analytics has already made a significant impact in various industries by using historical data to predict future outcomes. However, with advancements in technology and increased access to vast amounts of data from multiple sources, predictive analytics is expected to become even more sophisticated. This will allow businesses to anticipate changing market trends, customer behavior patterns, and make proactive decisions rather than reactive ones.
3) Emergence of Explainable AI:
Explainable AI (XAI) refers to the ability of artificial intelligence algorithms to provide a justification or explanation for their outputs. While AI models are currently being used extensively in decision-making processes without human intervention or understanding of how the decision was made, there is a growing demand for transparency and explainability. In the future, we can expect to see more emphasis on developing AI models that provide insights into their decision-making processes, leading to an increase in trust and ethical use of AI.
4) Greater Focus on Data Privacy and Security:
As businesses continue to collect huge amounts of data, there will be a greater focus on data privacy and security. With new data protection laws being introduced worldwide, like the General Data Protection Regulation (GDPR) in Europe and the California Consumer Privacy Act (CCPA) in the U.S., organizations will need to comply with strict regulations for handling personal data. This will lead to increased investments in data governance, privacy-enhancing technologies, and cybersecurity measures.
5) Democratization of Data Science:
Data science has traditionally been a highly specialized field accessible only to those with advanced technical skills. However, with the rise of user-friendly tools and platforms, we can expect to see a democratization of data science. This means that more non-technical professionals will be able to leverage data-driven insights for decision-making purposes without having extensive coding or statistical knowledge.
Impact of Data Science on Industries and Society
The rapid advancements in data science have brought about significant changes and disruptions across various industries and society as a whole. With its ability to analyze large and complex data sets, extract valuable insights, and make informed predictions, data science has become a crucial tool for businesses and organizations to stay competitive in today’s digital age.
Industries such as healthcare, finance, retail, transportation, marketing, and many others have been profoundly impacted by the implementation of data science techniques. Let’s explore how data science is changing these industries and shaping our society.
1. Healthcare:
Data science has revolutionized the healthcare industry by enabling accurate diagnosis, personalized treatments, drug discoveries, monitoring patient health records in real-time and predicting future health trends. With the help of machine learning algorithms and predictive analytics models, doctors can now make more accurate diagnoses at an early stage, thus improving patient outcomes. Additionally, wearable devices equipped with sensors are collecting patient data in real-time to provide continuous health monitoring and risk assessment.
2. Finance:
Data science has significantly transformed the finance industry by automating manual processes through advanced algorithms while providing critical insights to decision-makers. Investment firms are leveraging big data analytics to predict market trends accurately and make informed investment decisions. Banks also use fraud detection algorithms to prevent fraudulent activities in credit card transactions or loan applications.
3. Retail:
Retail companies are using customer shopping patterns analysis through data science techniques like collaborative filtering recommendations systems to personalize their marketing strategies based on individual preferences ultimately leading to increased sales revenue.
Challenges and Ethical Considerations in Data Science
As data science continues to rapidly evolve, it brings with it a host of challenges and ethical considerations that must be carefully navigated. In this section, we will discuss some of the most pressing issues facing the data science industry today.
1. Privacy and Data Security
One of the biggest challenges in data science is ensuring privacy and data security. With the increasing amount of personal information being collected and analyzed, there is a growing concern about how this data is being used and protected. From credit card numbers to sensitive health records, any type of personal information can be vulnerable if not handled properly. Data breaches are becoming more common, highlighting the need for stricter regulations and protocols for safeguarding sensitive data.
Data scientists must also consider ethical implications when accessing and using personal data for their research or business purposes. It is important to adhere to appropriate guidelines such as obtaining informed consent from individuals whose data is being used and ensuring anonymity wherever possible.
2. Bias in Data
Another challenge in data science is combating bias within datasets that may result in biased algorithms or models. This can occur due to several factors such as incomplete or skewed datasets, systemic biases embedded in historical data, or human error during the coding process.
If left unchecked, biased algorithms can perpetuate discrimination against certain groups of people based on race, gender, age, or other characteristics. This has serious ethical implications both for individuals affected by these biases as well as for society as a whole.
How to Prepare for a Career in Data Science?
Preparing for a career in data science requires a combination of technical skills, soft skills, and industry knowledge. It is a rapidly growing field with diverse opportunities, but it also has high competition. To stand out as a successful data scientist, you need to have the right qualifications and be up-to-date with the latest trends and technologies. In this section, we will discuss some practical steps on how to prepare for a career in data science.
1. Develop Your Technical Skills:
One of the essential requirements for becoming a successful data scientist is having strong technical skills. This includes proficiency in programming languages such as Python, R, SAS or SQL, familiarity with statistical analysis methods like regression and classification techniques, and experience with big data tools like Hadoop or Spark.
To acquire these skills, there are many online courses available from reputable institutions that you can take at your own pace. Additionally, participating in online coding challenges and attending workshops or boot camps can help improve your technical abilities.
2. Get Familiar With Data Science Tools:
Apart from programming languages and statistical analysis methods, there are many tools used by data scientists to manage and analyze large datasets efficiently. Some popular ones include Tableau for data visualization, TensorFlow for deep learning applications, and Apache Kafka for real-time stream processing.
Conclusion: Embracing the Future of Data Science
It is evident that data science has a bright future ahead. The advancements in technology, the growing demand for data-driven decision making, and the increasing availability of big data are all contributing to the evolving landscape of this field. The possibilities and potential of data science seem endless and companies across various industries are beginning to recognize its value.
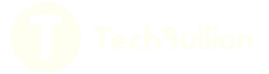