In today’s digital landscape, inundated with an incessant flow of data and information, distinguishing between the two is paramount for navigating the complexities of decision-making and knowledge acquisition. Although often used interchangeably, data and information serve distinct purposes, each contributing uniquely to our understanding of the world. So, what precisely sets them apart?
Introduction
In our digital epoch, data constitutes the raw, unprocessed elements—comprising figures, facts, and statistics—collected through observation, experimentation, or research endeavors. It exists in various forms, from numerical data to textual information, images, and videos. This raw material, akin to the building blocks of a structure, lacks inherent meaning or structure until processed and organized.
Conversely, information emerges as the product of processing and organizing data into a coherent, meaningful narrative. Through analysis and interpretation, data is imbued with context and relevance, offering valuable insights and facilitating decision-making processes. It’s akin to transforming scattered puzzle pieces into a comprehensible picture, where patterns and correlations come to light.
Crucial Differences Between Data and Information
1. Significance
Data: Data consists of raw, unprocessed facts or figures that lack inherent meaning or context. It serves as the foundational element that requires interpretation and organization to derive value.
Information: In contrast, information is the processed and organized form of data that possesses meaning, relevance, and context. It emerges through the analysis and interpretation of data, transforming disparate elements into coherent narratives or insights.
Example: Consider a spreadsheet containing customer purchase records. The raw data might include transaction IDs, item codes, and purchase amounts, which individually convey little meaning. However, when organized and analyzed to identify purchasing patterns or customer preferences, this data evolves into actionable information for targeted marketing strategies or inventory management.
2. Purpose
Data: The primary purpose of data is collection and storage for subsequent analysis. It serves as the raw material upon which information is constructed, providing the necessary inputs for decision-making processes.
Information: Information, on the other hand, serves the purpose of facilitating decision-making, problem-solving, or understanding specific phenomena. It provides actionable insights derived from the analysis and interpretation of the data.
Example: A retail manager may collect data on sales figures, inventory levels, and customer demographics. While the data itself offers little guidance, the information derived from analyzing this data can inform decisions regarding stock replenishment, promotional strategies, or customer segmentation.
3. Structure
Data: Data may exist in various forms, including structured, unstructured, or semi-structured formats. It lacks a predefined structure and may require organization before meaningful analysis can occur.
Information: Information adheres to a defined structure that facilitates comprehension and communication. It often employs visual aids, such as charts, graphs, or reports, to present insights in a digestible format.
Example: Raw survey responses from customer feedback may be unstructured data, comprising free-text comments or ratings. However, once categorized and summarized into thematic insights or visualized through word clouds or sentiment analysis, this information becomes comprehensible and actionable for the organization.
4. Relevance and Context
Data: Data may be relevant or irrelevant depending on its application or context. Its significance is often realized through subsequent analysis and interpretation within a specific framework or problem domain.
Information: Information inherently possesses relevance and context, as it emerges from the processing and analysis of data within a defined scope or objective. It provides actionable insights tailored to address specific questions or challenges.
Example: Consider social media analytics data, comprising metrics such as likes, shares, and comments. While these raw metrics hold inherent value, their significance and actionable insights only emerge when analyzed within the context of a marketing campaign’s objectives or target audience demographics.
Illustrative Examples
1. Sales Data vs. Sales Information: Raw sales figures lack meaningful insights, but once analyzed alongside demographic data or market trends, they transform into actionable sales information, guiding strategic decisions.
2. Weather Data vs. Weather Information: Temperature readings or wind speeds, in isolation, offer limited utility. However, when contextualized with geographic location and seasonal trends, they culminate in informative weather forecasts.
3. Customer Survey Data vs. Customer Insights: Feedback from customer surveys represents raw data until processed and analyzed to unveil underlying consumer preferences, thereby shaping product enhancements and marketing strategies.
Importance of Understanding the Difference
Understanding the disparity between data and information is imperative to harnessing the power of insights in today’s data-driven era. By discerning the nuances between these terms,
1. Accurate decision-making is facilitated as data is transformed into actionable insights, steering clear of misinterpretations or erroneous conclusions.
2. Fallacies such as correlation versus causation are mitigated, ensuring that conclusions drawn are based on robust analysis and contextual understanding.
3. Effective communication is fostered, enabling stakeholders to comprehend and leverage information effectively, thereby enhancing organizational efficacy.
How do you turn data into useful information?
To leverage the full potential of data, it’s essential to follow a systematic approach:
1. Define Objectives: Clearly outline the objectives to guide the analysis and interpretation of the data.
2. Cleanse and Organize: Ensure data integrity by cleansing and organizing it, eliminating redundancies and errors.
3. Analyze Data: Employ suitable analytical techniques to extract meaningful insights from the data.
4. Visualize Findings: Utilize visual aids to present insights comprehensively, facilitating easy interpretation.
5. Explore Perspectives: Delve into various facets of the data to gain holistic insights and uncover hidden trends.
6. Draw Conclusions: Base conclusions on evidence-backed findings, fostering informed decision-making.
7. Communicate Effectively: Present findings accurately and comprehensively to enhance understanding and utilization.
Conclusion
In essence, while data represents the raw material awaiting transformation, information epitomizes the culmination of processing and organizing data into actionable insights. By discerning the distinctions between these concepts and employing systematic methodologies for data analysis, we can unravel the complexities of our data-driven world, empowering informed decision-making and fostering organizational success.
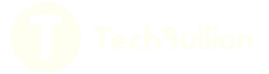