Introduction
Collecting and analyzing data is an essential aspect of any research or decision-making process. However, not all data is the same, and it is crucial to understand the different types of data and how to collect and analyze them effectively. In this section, we will delve into the various types of data and provide tips on how to collect and analyze them.
Tips on How to Collect and Analyze Data
1. Qualitative Data:
Qualitative data refers to non-numerical information that describes qualities or characteristics. This type of data can include interviews, open-ended survey responses, focus group discussions, observations, etc. To collect qualitative data, researchers often use techniques such as purposive sampling or snowball sampling to select participants who can provide rich insights into the research topic.
Analyzing qualitative data requires a coding process where themes and patterns are identified from the collected information. This process involves reading through the data multiple times to gain a deeper understanding of the underlying themes and then organizing them using software like NVivo or manually.
2. Quantitative Data:
Quantitative data consists of numerical values that can be measured objectively. It includes variables such as age, income level, test scores, etc., which are often collected through surveys or experiments involving large sample sizes.
To collect quantitative data accurately, researchers must ensure that their measurement instruments (e.g., surveys) have high reliability and validity. Analyzing quantitative data involves statistical methods such as descriptive statistics (mean, median, mode) and inferential statistics (t-tests, ANOVA) to identify patterns and relationships between variables.
3. Mixed-methods data:
Mixed-methods research combines both qualitative and quantitative approaches to collecting and analyzing data. This type of research provides a more comprehensive understanding by triangulating multiple sources of evidence.
To collect mixed-methods data effectively, it requires careful planning in terms of timing, sequencing of activities, and integration strategies for combining different types of data during the analysis stages. Researchers should also consider potential challenges, such as managing larger datasets while maintaining quality and consistency.
4. Big Data:
Big data refers to large and complex datasets that cannot be managed using traditional methods. It includes information from various sources, such as social media, web analytics, and sensor data collected in real-time.
Common Mistakes in Interpreting Data
Interpreting data is a crucial aspect of any data analysis process. It involves making sense of the information gathered and drawing meaningful conclusions from it. However, it can be a daunting task, especially for those who are not well-versed in data analysis. In this section, we will discuss some common mistakes that people make when interpreting data.
1) Drawing False Conclusions: One of the most common mistakes in interpreting data is drawing false conclusions. This happens when someone jumps to a conclusion without thoroughly analyzing the data or understanding its context. For example, if a study shows that there is a correlation between ice cream sales and shark attacks, one might conclude that eating ice cream causes shark attacks. However, this is an oversimplification of the data and does not take into account other factors such as seasonality and location.
2) Ignoring Outliers: Another mistake is ignoring outliers in the data. Outliers are values that deviate significantly from the rest of the data points. While they may seem like errors at first glance, they can provide valuable insights into trends or patterns that would otherwise go unnoticed. Ignoring outliers can lead to skewed interpretations and inaccurate conclusions.
3) Lack of context: data cannot be interpreted without considering its context. Without understanding where the data came from and how it was collected, one may misinterpret its meaning or draw incorrect conclusions. For instance, if you compare sales figures for two different time periods without taking into account external factors such as economic conditions or marketing campaigns, your interpretation will not accurately reflect reality.
4) Confusing Correlation with Causation: This mistake is similar to drawing false conclusions but specifically relates to confusing correlation with causation—assuming that because two things occur together, one caused the other. Establishing causation requires further investigation and evidence beyond just finding a correlation between two variables.
5) Not Checking for Biases: When interpreting data collected through surveys or other methods, it is essential to check for biases. Biases can occur in various forms, such as sampling bias or response bias, and can significantly affect the results of your analysis. It is crucial to be aware of these biases and consider them while interpreting the data.
Real-life Applications of Different Types of Data
Data is everywhere, and it plays a crucial role in our daily lives. From making decisions to predicting outcomes, data helps us understand the world around us. In this section, we will explore some real-life applications of different types of data and how they are used in various industries.
1. Personal Data:
Personal data is any information that relates to an identified or identifiable individual. It includes information such as name, address, contact details, and demographic information. This type of data is used in many ways, from personalizing advertisements to creating targeted marketing campaigns based on consumer behavior patterns. For instance, social media platforms collect personal data to improve the user experience by showing relevant content and suggesting new connections.
2. Geospatial Data:
Geospatial data refers to information with a geographic component attached to it. It can be collected using satellites, GPS devices, or even drones. This type of data is widely used in mapping applications like Google Maps and Waze for navigation purposes. It also has practical applications in urban planning, disaster management, and environmental research.
3. Financial Data:
Financial data includes all monetary transactions made by individuals or organizations. This type of data can provide valuable insights into market trends and consumer spending habits. Banks and financial institutions use financial data for risk analysis and fraud detection. Retailers use this type of data for inventory management and pricing strategies.
4.Specialized Data:
Specialized or domain-specific data refers to information that is unique to a particular industry or field of study. For example, medical records are specialized healthcare-related data used by doctors for diagnosis and treatment plans, while weather forecasters use specialized meteorological datasets for weather predictions.
5. Social Media Data:
With the rise of social media platforms like Twitter, Facebook, and Instagram, social media has become a goldmine for collecting vast amounts of unstructured text-based user-generated content (UGC) from millions of users worldwide. This valuable source provides insights into consumer sentiment, brand reputation, and market trends. Companies use this data to tailor their marketing campaigns and improve their products or services.
6. Machine-Generated Data:
Machine-generated data is produced by sensors, machines, and other smart devices. This type of data is used in the Internet of Things (IoT), where connected devices collect and share real-time information for analysis and decision-making. For example, smart home devices like thermostats and security cameras use machine-generated data to adjust settings based on user behavior patterns.
Conclusion
The power and importance of data cannot be overstated. It has revolutionized the way we make decisions and conduct business. As technology continues to advance, so will our ability to gather, analyze, and utilize data for various purposes. It is essential for individuals and organizations alike to understand the different types of data available and how to use them effectively for better decision-making processes.
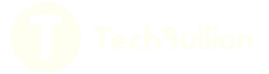