The world of robotics is undergoing a remarkable transformation, thanks to the power of synthetic data. In his latest work, Tanay Choudhary explores how artificially generated datasets are revolutionizing the way robotic systems are trained, tested, and deployed. His research delves into the innovative tools, methodologies, and applications that are accelerating development in this space.
The Rise of Synthetic Data in Robotics
Traditional data collection for robotics is an expensive and time-consuming process. Gathering real-world data often involves elaborate physical setups, human intervention, and extensive annotation efforts. Synthetic data, however, offers a cost-effective and scalable solution by simulating realistic environments, objects, and sensor interactions through advanced algorithms and simulation platforms. The adaptability of synthetic data is crucial for ensuring that robotic systems perform effectively across diverse and unpredictable environments, reinforcing its importance in modern robotics.
Closing the Reality Gap with Simulation
One of the biggest hurdles in using synthetic data is the “reality gap”—the differences between simulated environments and real-world conditions. To bridge this divide, researchers employ techniques such as domain randomization and sim-to-real transfer. Domain randomization introduces controlled variations in simulated environments, making robotic models more adaptable to real-world unpredictability. Sim-to-real transfer refines machine learning models by progressively integrating real-world data, ensuring that robots trained in simulations perform effectively outside the lab.
Cutting-Edge Tools for Synthetic Data Generation
The development of synthetic data has been powered by sophisticated simulation platforms. CARLA, an open-source simulator, is widely used for autonomous driving research, allowing developers to test self-driving algorithms in diverse traffic scenarios and weather conditions. Gazebo, a flexible simulation tool, provides a robust environment for industrial and service robots, integrating seamlessly with robotic operating systems. The variety of these tools showcases the diverse approaches taken in synthetic data generation to meet different robotics industry needs.
Advancing Safety and Efficiency
One of the key advantages of synthetic data in robotics is its role in enhancing safety and risk management. Testing robotic systems in real-world conditions can be hazardous and costly, particularly in applications like autonomous vehicles and surgical robotics. With synthetic data, developers can simulate critical failure scenarios, ensuring that robots are trained to handle extreme conditions before deployment. This not only improves safety but also reduces hardware damage and testing costs.
Expanding Applications Across Industries
Synthetic data is making waves in various robotics applications. In autonomous driving, synthetic datasets enable self-driving cars to learn how to navigate complex urban environments and respond to rare but crucial scenarios, such as emergency braking or pedestrian crossings. In warehouse automation, robotic arms and mobile units can be trained to optimize logistics, improving efficiency in large-scale operations. The healthcare sector is also benefiting from synthetic data, with robotic-assisted surgeries leveraging realistic simulations to refine precision and reduce patient risk. Beyond these industries, synthetic data is also finding applications in consumer robotics and space exploration, further broadening its impact.
The Future: AI-Powered Synthetic Data and Collaborative Simulations
Looking ahead, the integration of artificial intelligence with synthetic data generation is set to drive further innovation. AI-powered tools are enabling the creation of more realistic training datasets, reducing the reality gap and enhancing model performance. Automated scenario generation is also emerging as a game-changer, allowing developers to create edge-case scenarios that would be impossible to capture in the real world. Additionally, collaborative simulation environments, where multiple robotic agents interact within synthetic settings, are opening new avenues for multi-robot systems and complex autonomous behaviors. As computational power and AI capabilities continue to improve, synthetic data will become even more refined, making robotic systems more adaptable to real-world challenges.
In conclusion, synthetic data is reshaping the future of robotics by providing an efficient, scalable, and risk-free approach to training intelligent systems. Its ability to create diverse datasets tailored to specific applications makes it a powerful tool for driving advancements in artificial intelligence and automation. As the technology evolves, its role in bridging the gap between simulation and reality will become even more critical. With researchers like Tanay Choudhary leading the way, synthetic data is poised to drive innovation across industries, making robotics more adaptable, efficient, and capable than ever before.
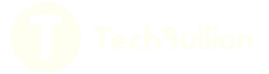