AI is the driving force behind many technological advances in modern industry. As the push for innovation continues, more and more data is required to help train and develop these complex algorithms.
Real data, data from actual events or objects that has been collated and analyzed by data scientists, has long been the only way to provide robust information for research and development.
While that remains a valid source of data production, it’s not necessarily the best solution for providing data to train and develop advanced AI algorithms.
The use of synthetic data is now at the forefront of AI development and is helping many industries make considerable advances in the building and testing of leading-edge algorithms.
What is Synthetic Data
Synthetic data is information artificially generated by an AI computer algorithm to mimic the structure of ‘real-world’ data.
It is created by feeding real-world data into an algorithm that can analyze and extract the patterns and statistical properties of the raw data. These patterns and properties are then used to produce new anonymized information that replicates the statistical distribution and behavior of the original data.
Advantages of using synthetic data
Protecting Sensitive Information – One of the main advantages of synthetic data is that it can be used to protect sensitive information. Since synthetic data is not real data, it can be shared without violating privacy laws or risking security breaches.
Reduces on Development Costs and Time – Synthetic data is a valuable resource for developing technology such as AI models because it offers affordable and quick access to data that can be used for training and testing purposes. Instead of spending time, resources, and money on collecting real-world data, AI development engineers can use synthetic data to train and validate newly developed algorithms. This enables engineers to test the concept of a model and fix errors or bugs before the product becomes customer-facing.
Limitations of using Synthetic Data
Real world accuracy – One limitation that can come with using synthetic data is that it might not always reflect real-world scenarios accurately. While synthetic data is designed to mimic real data, it may only capture some of the nuances and complexities of real-world situations. This can lead to biases or inaccuracies in AI models trained on synthetic data.
Proposed use – It’s also important to consider the proposed use of synthetic data as it may not be suitable for specific applications requiring actual real-world statistics.
For example, in the medical industry, there are some situations where authentic real world information with extraneous or anomalous points is essential for determining the safety or efficacy of a product. If this information had been used to create synthetic data on mass, these significant anomalies may have been ‘diluted’ in the distribution model.
Use Cases for Synthetic Data
Machine Learning – Synthetic data is ideal for using to develop and test machine learning models. Synthetic data can be used to increase the size and diversity of training information, making it easier to test and validate models.
Sharing of sensitive data – Synthetic data can also be used for data anonymization, allowing organizations to share data without compromising privacy or security. This is an ideal solution for financial institutions looking to limit fraudulent transactions and losses.
Simulations – Synthetic data is a fantastic tool to help simulate complex scenarios or environments, such as in the design of autonomous vehicles or virtual reality simulations.
AI is often used to train for ‘rare’ events, a good example of this is its use in early warning systems (EWS).
Natural disasters such as floods, earthquakes or volcanic eruptions are rare but can have devastating effects in a very short period of time. Due to their rarity and the short timeframe in which they occur and escalate, the amount of real world data we have on them is lacking.
By using AI to analyze the real world data that is available and then produce synthetic data to mimic its features at scale, we are able to train EWS on a much larger pool of potential scenarios and simulations to build a more robust and sensitive system.
What is Real Data
Real data is data that is collected from real-world events or objects. The data is generated by collecting information from various sources such as observations, experiments, sensors, or surveys. This information is then processed and analyzed to extract meaningful patterns and insights.
Advantages of using real data
Accuracy – One of the main advantages of using real data is its accuracy. Real data is collected from actual events or objects, making it more reliable and trustworthy. This accuracy can be critically important in fields such as medicine, finance, and engineering where even minor errors can have significant consequences.
Insight – Real data also reflects real-world scenarios and can provide valuable insights into how things work. This can help organizations make better decisions and improve their operations.
Algorithm Validation – Real data can be used to validate models and predictions, making it an important tool for decision-making. While synthetic data can be useful in certain situations, real data remains an essential resource for many organizations.
Limitations of using Real Data
Accessibility – One of the main limitations is that real data may not always be available or accessible. Collecting real data can be time-consuming, expensive, and sometimes impossible due to privacy or security concerns.
Incomplete or Biased – Real data may be incomplete or biased, especially if it is collected from a limited sample size or a specific population. This can lead to inaccuracies or errors in data analysis and decision-making.
Life Cycle – Real-world data has a definitive ‘life cycle’ and can quickly become out of date, especially in rapidly changing fields such as technology or finance.
Use Cases for Real Data
Medical research – One of the primary use cases for real data is in medical research, where actual patient data can be used to develop new treatments and therapies.
Finance – Real data is also essential in fields such as finance, where accurate and up-to-date data is critical for making informed investment decisions.
Supply and manufacturing – Real data can also be used to improve supply chain management by providing insights into inventory levels, demand patterns, and shipping times. It can be used to optimize manufacturing processes, by identifying inefficiencies and areas for improvement.
In conclusion, both synthetic and real data have their own unique applications and benefits in various fields. Synthetic data is particularly beneficial in situations where real data is difficult to obtain or where privacy concerns exist. On the other hand, real data provides a more accurate representation of real-world events and objects and is essential in fields such as medicine, finance, and logistics. However, it can be challenging to obtain and may be biased or incomplete.
Ultimately, the choice between synthetic and real data depends on the specific needs of the organization and the application at hand.
By understanding the strengths and limitations of each data type, organizations can make informed decisions on choosing the appropriate option to achieve the best results.
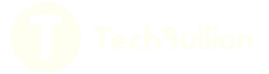