In recent years, the field of generative artificial intelligence (Gen AI) has seen significant progress and has transformed sectors like healthcare, manufacturing, automobiles & finance. The advent of Gen AI models like GPT-4, Claude, and Gemini, which have demonstrated remarkable capabilities in language understanding, content creation, and reasoning. However, these significant strides brought forth it’s fair share of challenges like maintaining performance, efficiency and adaptability as they scale. Finetuning and deploying sophisticated GenAI models require significant computational power, which can be costly and infrastructure-intensive. This meant that large organizations with deep pockets would largely be only able to leverage Gen AI.
Above listed challenges prompted the exploration of agentic systems, which offer a more modular and adaptive approach to AI. This article explores the emergence of ambient agentic systems (AAS) as a next evolution beyond standard agentic systems. By integrating context awareness, environmental perception, and distributed intelligence, ambient agentic systems represent a paradigm shift in how artificial intelligence interacts with and serves human needs. This paper examines the current limitations of standard agentic systems, and the transformative potential of ambient agentic systems to address complex challenges.
Standard Agentic System
Agentic AI is the broader concept of solving issues with limited supervision, whereas an AI agent is a specific component within that system that is designed to handle tasks and processes with a degree of autonomy. Agentic systems introduce a decentralized approach to AI. These systems consist of multiple autonomous agents that collaborate to achieve common goals. Key benefits include:
1) Autonomy and Decision-Making: Agents an operate independently, making decisions and taking actions without constant human input. They can analyze situations, develop strategies, and execute tasks in a way that Gen AI, which typically relies on explicit prompts, cannot.
2) Modular Approach: Agents can be developed, deployed, and updated independently, allowing for greater flexibility and scalability.
3) Compute Resource Optimization: Agents can distribute computational tasks more efficiently, reducing the overall resource demand.
4) Learning & Adaptability: Agents can learn and adapt to new tasks or environments with minimal retraining, enhancing the system’s resilience. This learning process allows them to scale easily and handle complex tasks that would require constant human intervention.
5) Simplified Integration: Agentic systems can be integrated into existing frameworks more seamlessly, reducing compatibility issues.
Standard agentic systems, although powerful, often struggle with the following issues:
1) Context Awareness: Standard agents may lack the ability to understand and adapt to the dynamic environment in which they operate.
2) User Interaction: These systems often require explicit user input, which can be cumbersome and less intuitive. This input drives the reasoning and decisioning making process. Wrongful interpretation can lead to unintended consequences and causing serious harm.
3) Scalability: As the complexity of tasks increases, standard agentic systems may face challenges in scaling efficiently in terms of having the right compute and reliable networks. The agents can only have one conversation at a time with an employee and is seen as a serious limitation
4) Integration: Implementing agentic systems into existing infrastructures can be difficult and may require significant modifications. This is significant when we are taking about legacy systems which may require additional planning and implementation.
5) Management and Oversight: As AI agents become more autonomous, managing their behavior, ensuring they operate within boundaries, and preventing unintended consequences which can cause significant harm becomes critical.
Solution: Ambient Agentic Systems
Ambient agentic systems represent a novel approach to addressing the limitations of traditional agentic systems. These systems are designed to be more context-aware, intuitive, and scalable. Several studies have explored the potential of ambient intelligence and agentic systems. For instance, Hung Du et al (2024) highlight the importance of Context awareness emerges as a pivotal element in fortifying multi-agent systems when dealing with dynamic situations. Here’s how they achieve this:
1) Enhanced Context Awareness:
Ambient agentic systems utilize real time user behavior and environmental awareness (by using advanced sensors and machine learning algorithms) to perceive and understand the context better. This allows them to make more informed decisions and adapt to changes in real-time. This is significant in terms of bridging the gap between real human intent and AI understanding of the need. For example, a real time ambient ai assistant would schedule meeting based on your time zone, resolve meeting conflicts and would ideally prioritize meetings based on your interaction and behavior.
2) Intuitive User Interaction:
Standard Agents require explicit commands but ambient agentic systems can interpret implicit cues and natural language. This makes interactions more seamless and user-friendly. For instance, a user might simply say, “I’m feeling cold,” and the say integrated ai assistant listening would adjust the thermostat without needing further instructions.
3) Scalability:
Ambient agentic systems are built with modularity in mind, allowing them to scale efficiently as the number of tasks and users increases. This is particularly beneficial in enterprise settings where the system needs to accommodate a growing number of employees and processes. AAS can perform multiple task taking in multiple cues and thus scale efficiently.
4) Seamless Integration with Internet of Things (IoT) & Other Smart Devices:
These systems are designed to integrate effortlessly with existing infrastructure and can efficiently communicate with other smart devices, Internet of Things (IoT) devices and systems. This also means predicting the needs even before they arrive. This interoperability ensures that ambient agentic systems can be a force multiplier rather than be seen as disruptor.
5) Improves Security:
These systems can significantly improve the security posture by continuously monitoring the environment for anomalies and notifying and possibly remediating them before it before a real threat.
6) Personalized Experiences:
By understanding user preferences and behaviors, ambient agentic systems can deliver highly personalized experiences. This is particularly valuable in customer service, where personalized interactions can lead to higher satisfaction and loyalty.
7) Usage across for Domains – Timing is Priceless:
The flexibility of ambient agentic systems allows them to be used across most domains, including healthcare, education, and transportation. For example, in healthcare, these systems can monitor patient vitals in real time and detect anomalies, run possible scenarios and provide timely analysis to medical professionals to take informed decisions.
8) AI Democratization
AAS intuitive and context aware capabilities simplifies the adoption of AI technologies, by seamlessly integrating with everyday environment bringing in AI inclusivity even for non-technical users.
Conclusion
The advent of AAS marks a new era in the field of artificial intelligence. Combining the power of Gen AI and standard agentic systems, AAS takes this evolution to a new level. This is making the agentic ai event more intelligent and significant. AAS ability to understand context, scale & intuitive user interaction leads to ushering in new era that will changes the way we interact with technology in our daily life while it does the job for us in background.
References
- Weiser, M. (1991). The Computer for the 21st Century. Scientific American, 265(3), 94-100.
- Russell, S., & Norvig, P. (2016). Artificial Intelligence: A Modern Approach (3rd ed.). Pearson.
- Introducing Ambient Agents
- Context-Aware Multi-Agent Systems: Techniques, Challenges and Future Directions
- Ambient intelligence: Technologies, applications, and opportunities
- How Ambient AI Enhances Agentic AI: A New Era of Intelligence
Manish has over 16+ years of Technology experience with expertise in Cloud and Automation. He provides technical leadership and best practices guidance to build best in class architecture, robust and scalable solutions in the cloud helping them achieve their desired business outcome. He is passionate about Generative AI, helping drive adoption and running security workshops to improve the security posture for his cloud customers.
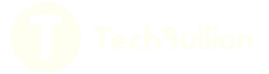