In an era where digital transformation accelerates at an unprecedented pace, cloud computing has become the backbone of modern enterprises. However, with this rapid evolution comes the challenge of optimizing cloud resources efficiently. Vineela Reddy Nadagouda, a Lead Site Reliability Engineer, delves into the role of Artificial Intelligence (AI) and automation in redefining capacity planning strategies. Her work provides critical insights into how businesses can leverage intelligent solutions to enhance operational efficiency, reduce costs, and maintain high-performance reliability.
The Shift from Traditional to Intelligent Planning
Historically, cloud capacity planning relied on static allocation models and manual oversight, often leading to inefficient resource utilization. Traditional methods struggled to accommodate fluctuating workloads, resulting in either over-provisioning, which drives up costs, or under-provisioning, which degrades performance. AI-driven capacity planning presents a transformative alternative, allowing cloud environments to adapt dynamically to changing demands. By employing machine learning algorithms, organizations can predict resource needs with high accuracy, ensuring optimal allocation at all times.
AI-Powered Demand Forecasting
One of the key advancements in cloud capacity planning is predictive analytics, powered by machine learning models. These algorithms analyze historical workload patterns, identifying trends that inform future resource allocation. Time-series forecasting models, such as ARIMA, have demonstrated up to 91% accuracy in CPU usage predictions. Deep learning techniques further enhance this process by refining data processing across multiple dimensions, reducing forecasting errors by nearly 50%. This level of precision enables enterprises to scale their infrastructure proactively, mitigating performance bottlenecks before they occur.
Automated Scaling and Dynamic Resource Allocation
Beyond predictive analytics, AI-driven automation has introduced sophisticated scaling mechanisms that respond to real-time fluctuations in demand. Unlike traditional threshold-based scaling, modern AI systems employ reinforcement learning to adapt to varying workload intensities. Adaptive auto-scaling can reduce service-level agreement (SLA) violations by 60%, ensuring uninterrupted service delivery. Additionally, intelligent throttling mechanisms optimize cost efficiency by dynamically adjusting resource distribution based on workload priorities, achieving up to 65% cost savings.
The Role of Edge Computing in Capacity Planning
As cloud environments grow more complex, integrating edge computing into capacity planning has emerged as a game-changer. By processing data closer to the source, edge computing minimizes latency, enhancing real-time decision-making capabilities. Studies show that edge-based optimization can reduce data transfer volumes by 80% while maintaining performance efficiency. This innovation is particularly crucial for Internet of Things (IoT) applications, where real-time responsiveness is critical.
Overcoming Implementation Challenges
While AI-powered capacity planning offers immense benefits, its implementation is not without challenges. Data quality remains a significant hurdle, as accurate predictions rely on clean, structured datasets. Additionally, feature selection plays a pivotal role in refining predictive models—optimized feature sets can improve forecasting accuracy by up to 40%. Organizations must also establish robust validation mechanisms to ensure AI models remain reliable over time, requiring frequent retraining and benchmarking.
Business Impact: Efficiency and Cost Optimization
The adoption of AI-driven capacity planning has already demonstrated measurable improvements in operational efficiency. Companies leveraging deep reinforcement learning have reported up to 45% reductions in resource wastage and 70% savings in energy consumption. Enhanced resource utilization not only translates to financial savings but also contributes to environmental sustainability by minimizing excessive energy use in data centers. Furthermore, AI-driven cost management strategies have led to up to 55% reductions in cloud expenditure, making intelligent automation a crucial investment for the future.
AI-Driven Capacity Management: How Tech Giants Optimize Resources Efficiently
Amazon uses AI-driven predictive analytics for AWS capacity planning, optimizing cloud resource allocation to reduce overprovisioning by 23% while maintaining 99.9% SLA reliability. Netflix employs AI for dynamic server scaling, ensuring smooth streaming during peak demand. Google leverages AI in data centers to cut cooling costs by 40%, enhancing server efficiency.
In conclusion,As organizations navigate the complexities of digital transformation, AI-driven capacity planning stands as a cornerstone of resilient cloud infrastructure. With innovations continuously emerging, businesses that embrace intelligent automation will maintain a competitive edge in an increasingly dynamic technological landscape. Vineela Reddy Nadagouda’s research underscores the importance of staying ahead of these advancements, ensuring that enterprises harness the full potential of AI for optimized cloud management.
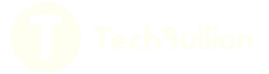