Introduction
Ordinal data is a type of data that is commonly used in research and statistical analysis. It is different from other types of data, such as nominal or continuous data, in the way that it represents the order or rank of a variable rather than exact numerical values. While ordinal data can provide valuable insights and information, there are also certain advantages and disadvantages to using this type of data.
Advantages of Using Ordinal Data
1. Easy to Collect: One of the main advantages of using ordinal data is that it is relatively easy to collect. This is because the responses are usually limited to a set number of options or categories, making it easier for researchers to gather and organize the data.
2. Simplifies Analysis: Ordinal data simplifies analysis by providing clear rankings or categories for variables. This makes it easier for researchers to identify patterns and trends in the data without having to deal with complex calculations.
3. Maintains Data Hierarchy: Unlike nominal or categorical data, ordinal data maintains the hierarchy between categories. This means that higher-ranked categories will always be considered “greater” than lower-ranked ones, providing a more accurate representation of the relationship between variables.
4. Suitable for Statistical Analysis: Ordinal data can be analyzed using both parametric and non-parametric statistical tests, depending on the distribution and sample size. This gives researchers more flexibility in choosing appropriate statistical methods for their analysis.
Disadvantages of Using Ordinal Data
1. Limited Information: The biggest disadvantage of using ordinal data is that it provides limited information compared to other types of quantitative data. For example, if we have an ordinal variable with three levels (low, medium, and high), we only know their relative order but not how much they differ from each other numerically.
2. Loss of Precision: Since ordinal variables do not have exact numerical values assigned to them, there may be a loss of precision when analyzing relationships between variables compared to continuous or interval-level variables.
3. Variations in Interpretation: The interpretation of ordinal data can vary among individuals, leading to potential discrepancies in analysis and conclusions drawn from the data. This is because the categories or rankings may hold different meanings for different people.
4. Limited Statistical Tests: While ordinal data can be analyzed using certain statistical tests, it may not be appropriate for more advanced techniques that require precise numerical values. This limits the types of analyses that can be performed on this type of data.
How to Collect and Analyze Ordinal Data
Collecting and analyzing ordinal data can provide valuable insights into patterns, trends, and preferences among a group of individuals. In this section, we will discuss the steps involved in collecting and analyzing ordinal data.
1. Determine the research question: The first step in any data collection process is to clearly define the research question or objective. This will help guide the selection of variables and ensure that the collected data is relevant to answering the research question.
2. Identify appropriate variables: As ordinal data deals with categories or levels of measurement, it is important to identify which variables are most relevant to the research question being asked. These could be demographic factors such as age, gender, income level, or specific traits or behaviors relevant to the study.
3. Choose an appropriate sampling method: Sampling refers to selecting a subset of individuals from a larger population for analysis. There are various methods of sampling, such as random sampling, stratified sampling, or convenience sampling. The choice of sampling method depends on factors like time constraints and the availability of resources.
4. Decide on survey design: Surveys are commonly used to collect ordinal data as they allow for a large number of responses from a diverse group of individuals. When designing a survey questionnaire, it is important to ensure that questions are clear and unambiguous so that respondents can easily understand and answer them.
5. Administer the survey: Once the survey has been designed, it can be administered either online or through face-to-face interviews, depending on the target audience and available resources.
6. Coding responses: After collecting responses from participants, it is necessary to code them before analysis can take place. Each response should correspond to its respective category or level in order to accurately represent their position on the scale.
7. Analyze using appropriate statistical methods: There are several statistical methods that can be used for analyzing ordinal data, such as chi-square tests or rank correlation coefficients. These methods can help identify relationships and patterns within the data.
8. Draw conclusions: The final step is to draw conclusions based on the analysis of the collected data. It is important to interpret the findings in relation to the research question and any limitations or biases that may have influenced the results.
Conclusion
Ordinal data plays a significant role in research and provides a unique perspective that other types of data do not. By understanding its characteristics and differences from other types of data, we can ensure that our analysis and interpretation are accurate and meaningful. Whether you are conducting your own research or reading someone else’s findings, having a solid understanding of ordinal data will help you better understand and critique the information presented.
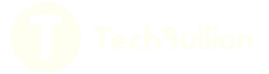