Are you ready to dive into the world of data and unlock its mysteries? In this blog post, we’ll be exploring the fascinating realm of ordinal data—what it is, how it differs from other types of data, and why it’s so important in the field of research and statistics.
Introduction to Ordinal Data
Ordinal data is a type of categorical data that is often used in statistical analysis. Unlike other types of data, such as continuous or nominal data, ordinal data has a specific order or sequence to it. In this section, we will dive deeper into what exactly ordinal data is and how it differs from other types of data.
What is ordinal data?
Ordinal data is a type of data that falls under the category of categorical or qualitative data. It is used to represent information that can be organized into distinct categories or ranks but does not have numerical values. This makes it different from other types of data, such as continuous or discrete data.
To understand ordinal data better, let’s first look at its characteristics. Ordinal data has an inherent order or hierarchy among its categories, meaning one category is considered “higher” than another. For example, in a survey asking participants to rate their level of satisfaction with a product on a scale of 1–5, the responses would be considered ordinal because they have an ordering, with 5 indicating the highest level of satisfaction and 1 representing the lowest.
In addition to having an inherent order, ordinal data also has unequal intervals between its values. Unlike continuous or discrete data, where the difference between any two consecutive numbers is equal (e.g., 5-6=1), ordinal data may have varying differences between its values depending on the context. Using our previous example, while there may be only one unit difference between each value (e.g., from “satisfied” to “very satisfied”), there could potentially be a larger gap between each value above that (e.g., from “very satisfied” to “completely satisfied”).
One important note about ordinal data is that while it has some characteristics similar to numerical data, it cannot be treated as such. This means that mathematical operations like addition and multiplication cannot be performed on it, as these operations would not make sense for this type of information. Going back to our earlier example, it would not make sense to add someone’s level of satisfaction with a product (e.g., rated as ‘4’) to someone else’s rating (e.g., rated as ‘2’).
Characteristics of Ordinal Data
Ordinal data is a type of categorical data that represents the ranking or order of a set of categories. It differs from other types of data, such as nominal and interval, in its unique characteristics. In this section, we will delve deeper into the specific features that make ordinal data distinct.
1. Ranking or Order
The most prominent characteristic of ordinal data is that it has an inherent order or ranking among its categories. This means that each category can be assigned a numerical value based on its position in the series. For example, if we have a dataset representing different levels of education (high school, college, and graduate school), high school would be ranked as 1, college as 2, and graduate school as 3. This order is essential for analyzing and interpreting ordinal data accurately.
2. Unequal Intervals
Unlike interval data, where the intervals between values are equal, ordinal data does not have uniform intervals between categories. The difference between two consecutive ranks may not necessarily be the same throughout the entire dataset. For instance, going from rank 1 to rank 2 may represent a larger leap than going from rank 4 to rank 5.
3. Limited Mathematical Operations
Due to unequal intervals and a lack of proportionality among categories, mathematical operations like addition and multiplication cannot be performed on ordinal data. It limits our ability to calculate measures like the mean or standard deviation for this type of data accurately.
4. Limited Number of Categories
Ordinal data typically consists of a limited number of categories or ranks compared to nominal or interval data sets, which can have an infinite number of options for categorization or measurement.
5.Boundary Issues
Ordinal scales often face boundary issues, which occur when there is ambiguity about whether certain values belong to one category or another due to overlapping boundaries between them.
6.Negative Ranks
Another key feature distinguishing ordinal scales from other types is their potential inclusion of negative ranks, indicating either zero value (no particular rank) or less than the first category.
7. Non-Numeric Labels
While interval and ratio data have numerical labels, ordinal data may not necessarily have numeric values as their labels. For example, categories like “large,” “medium,” and “small” cannot be assigned numerical values but can still be ranked in an ordered series.
Understanding these characteristics of ordinal data is crucial for accurately analyzing and interpreting this type of data. The unique properties of ordinal scales require different statistical techniques compared to other types of data, making it essential to correctly identify them in a dataset.
How is it different from other types of data?
Ordinal data is a type of data that is often used in statistical analysis and research studies. It differs from other types of data, such as nominal or interval data, in several ways. In this section, we will explore the key features of ordinal data and how it sets itself apart from other types of data.
To begin with, let’s define what ordinal data is. Ordinal data is a categorical type of data where the values represent a ranked order or position. This means that the categories have a natural ordering to them, but the numerical difference between each value cannot be determined. For example, if we were to gather information on students’ academic performance by asking them to rank their grades from best to worst (1st place, 2nd place, 3rd place), we would be collecting ordinal data.
One way in which ordinal data differs from nominal and interval data is in its level of measurement. Nominal and ordinal are both categorical types of measurement, while interval and ratio are numerical types. However, unlike nominal variables, where each category holds equal weight and there is no inherent order among them (e.g., hair color or gender), ordinal variables have an ordered relationship among their categories (e.g., level of education or socioeconomic status). This means that for ordinal variables, the categories can be arranged in a logical sequence based on their respective magnitudes.
Another important distinction between ordinal and other types of data lies in the way they are represented numerically. While interval and ratio variables have equal intervals between their numbers (e.g., temperature measured in Celsius), this does not apply to ordinal variables as there is no consistent numerical difference between categories (e.g., Likert scale responses ranging from strongly disagree to strongly agree). As such, performing mathematical operations like addition or subtraction on these values would not yield meaningful results.
Unlike nominal variables, where mode is the only measure of central tendency that can be used to describe the data, ordinal variables allow for the use of median and mode as measures of central tendency. This is because ordinal data can be ranked and ordered, allowing for the calculation of the median, which represents the middle value when all categories are arranged in order.
Examples of Ordinal Data in Real Life
Ordinal data is a type of data that is used to categorize and rank different levels or categories. It differs from other types of data, such as nominal and interval, because the categories have a specific order or hierarchy. In this section, we will explore some real-life examples of ordinal data to gain a better understanding of how it is used in various fields.
1. Education: One common example of ordinal data in education is academic grades. Grades are typically assigned to students based on their performance in exams or assignments. These grades follow a specific order, with A being the highest and F being the lowest. This ranking system allows for easier comparison between students’ performances and helps identify high-achieving students.
2. Customer Satisfaction Surveys: Many companies use customer satisfaction surveys to gather feedback about their products or services. These surveys often include questions where customers are asked to rate their experience on a scale from “very satisfied” to “very dissatisfied.” This rating system follows an ordinal scale as there is an implied hierarchy, with “very satisfied” being the most positive and “very dissatisfied” being the most negative.
3. Likert Scales: Likert scales are commonly used in research studies to measure attitudes or opinions towards a particular subject. They consist of statements that respondents can agree or disagree with on a five-point scale ranging from “strongly agree” to “strongly disagree.” The responses obtained from Likert scales can be treated as ordinal data as they have an inherent order.
4. Medical Conditions: In medicine, many diagnoses are categorized using an ordinal scale called the New York Heart Association Functional Classification (NYHA). This classification ranks patients’ heart disease symptoms on a scale from I (no symptoms) to IV (severe limitations). This information helps doctors determine appropriate treatment plans for their patients based on the severity of their condition.
Conclusion
While there may be some similarities between ordinal data and other types of data, it is clear that ordinal data possesses unique characteristics that set it apart from nominal or interval data. Its hierarchical structure and limited numerical properties make it a valuable tool in statistical analysis and research studies. Understanding these differences is crucial to accurately interpreting and utilizing ordinal data in various fields of study.
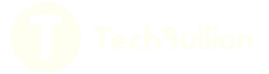