Welcome to our thought-provoking blog post on the fascinating world of Artificial Intelligence (AI) and its ethical implications. As AI continues to shape various aspects of our lives, it is crucial that we delve into the intricate web of biases lurking within this groundbreaking technology. Today, we embark on a captivating journey together, exploring the depths of bias in AI and unraveling its profound consequences for humanity. Join us as we shed light on this imperative topic and empower ourselves with knowledge to address these ethical concerns head-on.
Introduction to Artificial Intelligence
Artificial intelligence (AI) is a rapidly growing field that has the potential to revolutionize the way we live, work, and interact with each other. It involves the development of intelligent machines that can perform tasks that usually require human intelligence, such as visual perception, speech recognition, decision-making, and problem-solving.
The concept of AI dates back to ancient times, but it was not until the mid-20th century that scientists and researchers started making significant progress in this field. Today, we are surrounded by AI-powered technology in our daily lives – from virtual assistants like Siri and Alexa to self-driving cars and advanced medical diagnostics systems.
As AI continues to advance and become more integrated into our society, it is important for us to understand its impact on various aspects of our lives. In this section of the blog article “Addressing Ethical Concerns: Analyzing Biases in Artificial Intelligence,” we will delve deeper into the introduction of artificial intelligence and its potential implications on society.
Understanding Bias in AI: What is it?
Artificial intelligence (AI) has become a powerful tool in various industries, from healthcare to finance, and is expected to continue growing in importance in the coming years. However, with this growth comes the recognition that AI systems can have biases that can lead to discriminatory outcomes. These biases are a result of the data used to train AI algorithms, as well as the programming and decision-making processes within these systems.
But what exactly is bias in AI? Simply put, bias refers to any systematic error or deviation from an accurate representation of reality. In other words, it is when a particular group or characteristic is favored over another in the decision-making process of an AI system. This can happen due to various reasons such as incomplete data, human prejudices and assumptions embedded into algorithms, or lack of diversity among developers creating these systems.
One common misconception about bias in AI is that it only occurs intentionally. However, it can also be unintentional and stem from underlying societal inequalities and stereotypes present in our everyday lives. For example, if an AI system is trained on historical data that reflects past discriminatory practices against certain groups of people, it will likely perpetuate those biases even if they are no longer relevant or valid.
It’s important to note that not all types of bias are inherently negative or harmful. Some may even be beneficial for specific purposes and contexts. For instance, a facial recognition system designed for security purposes may prioritize identifying potential threats accurately over accurately recognizing individuals’ race or gender.
Types of Biases in AI
Artificial Intelligence (AI) has become an integral part of our daily lives, from virtual assistants to self-driving cars. With its growing presence, the need to address ethical concerns in AI has become increasingly important. One such concern is the potential for biases in AI systems.
Biases are defined as preconceived notions or prejudices that can influence a person’s judgment and decision-making process. In the case of AI, these biases can be unintentionally incorporated into algorithms and data sets used to train AI systems, leading to biased outcomes. Let us take a closer look at some of the types of biases that can exist in AI.
1. Data Bias:
Data bias occurs when there is a lack of diversity or representation in the data used to train an AI system. This could be due to various factors such as historical discrimination, limited data sources, or human error during data collection. For example, if an AI system is trained on data that primarily represents one gender or race, it may not accurately reflect the real world and lead to biased decisions.
2. Algorithmic Bias:
Algorithmic bias occurs when the algorithm itself is designed with inherent biases based on certain assumptions made by its creators. These assumptions are often influenced by societal norms and values, which may not always align with ethical principles. For instance, an algorithm designed to screen job applicants may discriminate against candidates with non-English sounding names or those who have gaps in their employment history.
3. Interaction Bias:
Interaction bias refers to how humans interact with AI systems and how these interactions can influence the performance of the system. For instance, if a virtual assistant is programmed to respond to certain phrases or words, it may not accurately understand or respond to individuals who speak with accents or have speech impediments.
4. Measurement Bias:
Measurement bias occurs when the metrics used to evaluate the performance of an AI system are biased. This could happen when the metrics themselves are based on historical data that reflects societal biases. For example, if a hiring algorithm is trained on data from past hiring decisions which were biased towards a certain gender or race, it will continue to perpetuate those biases.
5. Feedback Loop Bias:
Feedback loop bias occurs when an AI system’s decisions reinforce existing biases in society. For instance, if an algorithm is used to recommend job opportunities and it primarily suggests jobs in traditionally male-dominated fields to female candidates, this can further perpetuate gender stereotypes and discrimination.
6. Automation Bias:
Automation bias refers to the tendency for humans to rely too heavily on AI systems without questioning their decisions. This can be problematic if the AI system is biased, as it can lead to discriminatory outcomes without any human intervention.
Case Studies: Examples of Bias in AI
As artificial intelligence (AI) continues to advance and play a larger role in our daily lives, it is important to examine the potential biases that may exist within these systems. AI algorithms are created by humans, who may unknowingly embed their own biases and prejudices into the technology. This can lead to discriminatory outcomes and reinforce existing societal inequalities. In this section, we will explore several case studies that highlight examples of bias in AI.
1. Facial Recognition Technology:
Facial recognition technology is used in various industries such as security, marketing, and law enforcement. However, numerous studies have shown that these systems often exhibit racial and gender bias. For example, a study by MIT found that facial recognition software had higher error rates for darker-skinned individuals compared to lighter-skinned individuals. This is due to the lack of diversity in the data sets used to train these algorithms, which primarily consist of white faces.
Another concerning aspect of facial recognition technology is its use in law enforcement. The American Civil Liberties Union (ACLU) conducted a study on Amazon’s facial recognition software and found that it falsely matched 28 members of Congress with mugshots from a database of criminal suspects. Furthermore, these false matches disproportionately affected people of color.
2. Employment Algorithms:
Many companies now use AI algorithms during the hiring process to screen resumes and select candidates for interviews. However, these systems have been found to perpetuate gender bias by favoring male candidates over equally qualified female candidates.
In one study by researchers at Carnegie Mellon University, it was found that resumes with female names were less likely to be selected for interviews compared to identical resumes with male names. This bias may be a result of the data used to train these algorithms, which may reflect historical hiring practices that favor men.
3. Predictive Policing:
Predictive policing is a method that uses AI algorithms to forecast potential criminal activity and allocate police resources accordingly. However, these systems have been criticized for perpetuating racial bias in law enforcement.
In one study by researchers at Dartmouth College, it was found that predictive policing software used by the Chicago Police Department was biased against communities of color. The algorithm had higher predictions of crime in predominantly Black and Latino neighborhoods compared to white neighborhoods, leading to increased surveillance and police presence in these areas.
4. Loan Approval Systems:
AI algorithms are also used in loan approval processes to assess the creditworthiness of individuals seeking loans. However, these systems have been found to discriminate against people of color and low-income individuals.
Consequences of Biased AI on Society
The rapid advancement of artificial intelligence (AI) has brought about numerous benefits to society, from streamlining processes in various industries to improving daily lives through virtual assistants and personalized recommendations. However, as with any technological development, there are also ethical concerns that must be addressed. One such concern is the presence of biases in AI systems.
Biases can be defined as the systematic and often unconscious favoritism towards certain groups or individuals over others. In the context of AI, these biases refer to the tendency for algorithms to produce discriminatory results based on race, gender, age, socio-economic status, and other factors. These biases can have significant consequences on society and can perpetuate existing inequalities if left unaddressed.
One of the most alarming consequences of biased AI is its impact on decision-making processes. Many critical decisions in our society today are being made with the assistance of AI systems – from hiring practices to loan approvals and even criminal sentencing. If these systems are built upon biased data or flawed algorithms, they can perpetuate discrimination against already marginalized communities. For example, a biased facial recognition algorithm may falsely identify a person of color as a suspect leading to wrongful arrests.
Another consequence is the reinforcement of societal stereotypes and norms through biased AI systems. These systems learn from historical data that reflect societal biases and prejudices which can then be amplified when used in decision-making processes. This creates a feedback loop where these biased decisions further reinforce existing stereotypes and contribute to their perpetuation.
Addressing Biases in AI: Current Efforts and Challenges
The use of artificial intelligence (AI) has become increasingly prevalent in our daily lives, from personalized advertisements to virtual assistants. While AI technology has the potential to bring about many positive advancements, it also raises ethical concerns such as biases.
Biases in AI refer to systematic errors or unfairness that can occur due to the algorithms and data used in developing the AI system. These biases can lead to discriminatory outcomes, perpetuating societal inequalities and reinforcing existing prejudices.
In recent years, there have been efforts made by researchers, industry experts, and policymakers to address biases in AI. However, these efforts are still faced with numerous challenges.
Current Efforts:
1. Diverse Data Collection:
One of the main causes of bias in AI is biased data used for training the algorithms. To combat this issue, there have been initiatives to collect more diverse datasets that represent a wider range of demographics and perspectives. For example, facial recognition software companies have started collecting more diverse images for their databases to reduce racial and gender biases.
2. Transparency and Accountability:
Transparency is crucial for identifying and addressing biases in AI systems. Some organizations have started implementing measures such as publishing information on their algorithms’ decision-making process or conducting regular audits to ensure fairness and accountability.
3. Bias Detection Tools:
There has been an emergence of tools designed specifically to detect biases in AI systems. These tools use various methods such as statistical analysis and algorithmic testing to identify potential discriminatory patterns in the data and algorithms.
4. Ethical Guidelines:
Several organizations, including The Institute of Electrical and Electronics Engineers (IEEE) and The European Commission, have published ethical guidelines for the development and use of AI. These guidelines aim to promote responsible and fair use of AI technology while addressing issues such as bias.
5. Collaboration:
Addressing biases in AI requires collaboration between various stakeholders, including researchers, policymakers, industry experts, and affected communities. Many organizations have started collaborating to develop best practices and strategies for mitigating biases in AI.
Challenges:
1. Lack of Diversity in the Tech Industry:
The lack of diversity within the tech industry is a major challenge in addressing biases in AI. In 2020, only 26% of computing jobs were held by women, and even fewer were held by people from underrepresented racial or ethnic groups. This lack of diversity hinders the development of more inclusive and unbiased AI systems.
2. Limited Access to Data:
Data collection is crucial for addressing biases in AI, but there are often limitations on access to diverse datasets due to privacy concerns or proprietary data ownership. This makes it challenging for researchers to build unbiased models.
3. Unintentional Biases:
Even with efforts made towards diverse data collection and algorithmic transparency, unintentional biases may still exist in AI systems. This is due to the complex nature of data and the difficulty in predicting all potential outcomes.
4. Cost and Time Constraints:
Addressing biases in AI can be costly and time-consuming. It requires significant resources for data collection, algorithm development, and testing. This can be a barrier for smaller organizations or startups with limited resources.
5. Lack of Regulation:
There is currently no comprehensive regulation on AI technology, making it challenging to hold organizations accountable for biased decision-making by their algorithms. Without proper regulation, it can be difficult to enforce ethical guidelines and ensure fairness in AI systems.
Ethical Considerations in Developing Unbiased AI
When it comes to the development of artificial intelligence (AI), one of the most pressing concerns is the potential for bias. As AI systems become more prevalent in our daily lives, it is crucial to address any biases that may exist and work towards creating unbiased AI. In this section, we will delve into the ethical considerations surrounding the development of unbiased AI.
1. Understanding Bias in AI:
Before diving into ethical considerations, it is important to have a clear understanding of what bias in AI means. Bias can be defined as a tendency or preference towards certain groups or individuals based on their characteristics such as race, gender, age, etc. In relation to AI, bias refers to unfair or discriminatory treatment towards certain groups due to how data is collected and used by algorithms.
2. The Impact of Biased AI:
The consequences of biased AI can be far-reaching and detrimental. For instance, biased hiring algorithms can lead to discrimination against certain groups in job opportunities. Similarly, facial recognition software with built-in racial bias has been shown to disproportionately misidentify individuals from marginalized communities.
3.Bias in Data Collection:
One major source of bias in AI is its reliance on data for decision making. If the data used to train an algorithm contains inherent biases or reflects societal prejudices, then these biases are likely to be perpetuated by the algorithm. For example, if historical data used for predicting loan approvals has consistently favored individuals from a particular demographic group due to past discriminatory practices by banks, then an algorithm trained on this data will continue to perpetuate this bias.
4. Ensuring Diversity in Data:
To mitigate the risk of biased AI, it is essential to ensure diversity in the data used for training. This means collecting data from a broad range of sources and avoiding datasets that are skewed towards a particular group. It is also important to regularly review and update data sets to account for any changes in societal norms or biases.
5. Transparency and Explainability:
The lack of transparency and explainability in AI algorithms can make it difficult to identify and address bias. Developers should strive to create algorithms that are explainable and can be audited for potential biases. This not only helps in identifying any problematic biases but also builds trust with end-users.
6. Diversity in Development Teams:
Having a diverse team involved in the development of AI can help identify and address biases that may go unnoticed otherwise. A team with diverse perspectives can offer different insights into potential biases and ensure that multiple viewpoints are considered during the development process.
7. Ongoing Monitoring and Evaluation:
Developers should continuously monitor their AI systems for potential biases, even after deployment. Regular evaluation of the algorithm’s performance can help identify any unintended consequences or discriminatory outcomes, which can then be addressed promptly.
Conclusion
It is clear that addressing ethical concerns and analyzing biases in artificial intelligence is crucial for creating a fair and just society. As technology continues to advance, it is our responsibility to ensure that these systems are designed and developed with ethical considerations in mind. By being aware of potential biases and actively working towards mitigating them, we can create AI systems that truly benefit all individuals, regardless of race, gender, or other factors. Let us continue to have open discussions and take action towards building a more equitable future through responsible development of artificial intelligence.
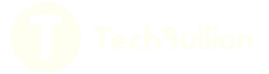