Welcome to our eye-opening blog post, where we delve into the world of Artificial Intelligence (AI) to uncover a disturbing truth that has long remained silent. While AI continues to revolutionize various aspects of our lives, from personal assistants to driverless cars, there is an unsettling reality lurking beneath its seemingly flawless façade – the existence of silent bias. Join us as we embark on an exploration that unveils how biased algorithms can perpetuate discrimination and inequality in our increasingly digital world. Brace yourself for insights that will challenge your perception of AI and inspire us all to demand an equitable future!
Introduction to AI and its Impact on Society
Artificial Intelligence, or AI, has become a buzzword in today’s society. From virtual assistants like Siri and Alexa to self-driving cars and automated customer service chatbots, AI is becoming increasingly integrated into our daily lives. But what exactly is AI, and how does it impact society?
AI refers to the ability of machines to perform tasks that would typically require human intelligence such as problem-solving, decision making, speech recognition, and language translation. This technology has rapidly advanced over the years thanks to developments in machine learning algorithms and big data processing.
The concept of intelligent machines has been around for centuries, but it wasn’t until the 1950s that scientists began actively exploring the possibilities of creating artificial intelligence. Since then, there have been significant advancements in the field with computers now able to perform complex tasks at lightning-fast speeds.
Today, AI impacts almost every aspect of our lives – from healthcare and transportation to education and entertainment. It promises greater efficiency, increased productivity and convenience for individuals and businesses alike. However, as with any new technology, there are also concerns about its potential negative impact on society.
Understanding Bias and Its Importance in AI
Artificial Intelligence (AI) has become an integral part of our daily lives, from virtual assistants to self-driving cars. However, as AI technology continues to advance, it is essential to address the issue of bias within it. Bias in AI refers to the systematic and unjustifiable exclusion or discrimination of certain groups or individuals based on race, gender, age, ethnicity, religion, sexual orientation, disability and other characteristics. This inherent bias poses significant ethical concerns and can have profound consequences on society and our understanding of fairness.
The Need for Understanding Bias in AI
Bias in AI arises due to the input data used by algorithms that influence their decision-making process. These algorithms are designed by humans who may unconsciously embed their own biases into them through the selection of training data or setting biased objectives for the algorithm. Thus, bias in AI is not a technological problem but rather a human one.
It is important to understand and address this bias as AI systems are increasingly being utilized for decision making processes such as hiring practices, loan approvals, medical diagnoses and criminal justice assessments. The decisions made by these systems can significantly impact people’s lives; hence understanding how bias affects these outcomes is crucial.
Types of Biases in AI
When we hear the term “artificial intelligence,” we often think of advanced technology that has been programmed to make decisions without human intervention. However, what many people fail to realize is that AI systems are created and trained by humans, with all of their inherent biases and prejudices. This leads to the emergence of biased algorithms, which can have serious consequences in areas such as hiring decisions, criminal justice, and financial services. In this section, we will explore the different types of biases that exist in AI systems and their potential impact.
1. Confirmation Bias:
Confirmation bias is a type of cognitive bias where individuals tend to seek out information that confirms their existing beliefs while ignoring contradictory evidence. When it comes to AI, confirmation bias can be introduced during the data collection stage where only certain types of data are used to train an algorithm, leading to skewed results. For example, if an AI system is designed for resume screening but is trained on resumes from a specific demographic group rather than a diverse pool of applicants, it may favor candidates from that particular group over others.
2. Selection Bias:
Selection bias occurs when there is a distortion in the sample used for training an algorithm leading to incorrect conclusions being drawn. This type of bias often stems from inadequate or unrepresentative data sets being used as input for machine learning algorithms. For instance, if an image recognition system is trained on images primarily featuring men due to underrepresentation or lack of diversity in the training data set; it may show higher accuracy in recognizing men over women.
3. Automation Bias:
Automation bias occurs when people have an excessive trust in machines, assuming that they are infallible and always make the right decisions. This type of bias can lead to humans blindly following the decisions made by AI systems without questioning their validity. For example, if a medical diagnosis system recommends a particular treatment, doctors may be inclined to follow it without considering other factors or using their own judgment.
4. Gender Bias:
Gender bias in AI refers to the differential treatment of individuals based on their gender. This can occur in various forms, such as language processing algorithms showing higher accuracy in understanding male voices over female voices or facial recognition systems having difficulty recognizing faces of people with darker skin tones due to a lack of diversity in training data.
5. Algorithmic Bias:
Algorithmic bias refers to the systemic and recurring errors in decision-making by algorithms due to biased data and/or biased coding by human programmers. This type of bias is difficult to identify and often goes unnoticed until it results in discriminatory outcomes, such as recommending higher-paying jobs only to men or denying loans to certain racial groups.
Examples of Bias in AI Systems
As artificial intelligence (AI) continues to advance and become more integrated into our daily lives, it is becoming increasingly important to address issues of bias within these systems. While AI may seem like an unbiased and impartial tool, the reality is that it reflects the biases and prejudices of its creators. In this section, we will explore some notable examples of bias in AI systems, highlighting their impact on society and the need for careful consideration when creating and implementing these technologies.
1. Facial Recognition Technology:
Facial recognition technology has gained widespread use in recent years, with applications ranging from security to social media platforms. However, studies have shown that this technology tends to be biased against certain racial groups, specifically people of color. One study by MIT researchers found that facial recognition software had a higher error rate for identifying darker-skinned individuals compared to lighter-skinned ones. This can lead to serious consequences such as misidentification by law enforcement or denial of access to services based on flawed facial recognition data.
2. Gender Bias in Hiring Processes:
Many companies are now using AI-based algorithms for hiring processes in order to remove human biases from the equation. However, these algorithms have been found to perpetuate gender stereotypes by favoring male candidates over equally qualified female candidates. This is because the algorithm is trained on historical data which contains inherent biases due to the underrepresentation of women in leadership positions.
3.Racial Bias in Criminal Sentencing:
In recent years, there has been growing concern over the use of AI algorithms in predicting criminal recidivism, which is used to inform judges’ decisions on sentencing. These algorithms have been found to be biased against people of color, resulting in longer prison sentences being given to them compared to white defendants.
4. Language Processing Bias:
Language processing AI systems are used in various applications such as chatbots, virtual assistants, and translation services. However, these systems have shown biases towards certain dialects and accents, leading to inaccuracies and misunderstandings for non-standard speakers or those with accents perceived as “foreign”. This can have serious consequences, especially in customer service interactions and legal proceedings.
5. Financial Bias:
AI algorithms used in finance can also reflect societal biases by excluding certain demographics from receiving loans or offering them higher interest rates. This was brought to light when Apple Card came under scrutiny for giving lower credit limits to women compared to men with similar credit scores. Similarly, AI algorithms used in determining insurance premiums may discriminate against people of certain races or socioeconomic backgrounds.
The Negative Effects of Biased AI
The development and use of AI technology has grown significantly in recent years, with its impact being felt in various industries such as healthcare, finance, transportation, and many others. While AI has the potential to streamline processes and improve efficiency, there is a growing concern about the negative effects of biased AI on society.
Biased AI refers to the unintentional discrimination that occurs when artificial intelligence systems are developed with inherent biases or trained on data sets that reflect societal biases. This can lead to discriminatory outcomes for certain groups of people, perpetuate existing inequalities, and even reinforce harmful stereotypes.
One of the most troubling aspects of biased AI is its impact on marginalized communities. As AI algorithms are trained on historical data that may contain built-in biases against certain groups based on race, gender, age or socioeconomic status, these prejudices can be inadvertently embedded into the system. This means that automated decisions made by biased AI can have far-reaching consequences for individuals from underrepresented or vulnerable communities.
For example, a study found that facial recognition software had higher error rates when identifying darker-skinned individuals compared to lighter-skinned ones. This highlights how biased algorithms can disproportionately affect people of color and potentially lead to false arrests or wrongful convictions.
Addressing and Preventing Bias in AI
Addressing and preventing bias in AI is a crucial step towards creating a fair and just society. Bias in artificial intelligence refers to the systematic errors or discrepancies in data and algorithms that can lead to discriminatory outcomes, reinforcing existing stereotypes and inequalities.
The use of AI has been rapidly expanding in various industries, including healthcare, finance, education, criminal justice, and employment. While it promises efficiency and accuracy, it also perpetuates discrimination against marginalized groups by reflecting the biases of its creators.
Fortunately, there are steps that can be taken to address and prevent bias in AI. In this section, we will discuss various strategies that individuals and organizations can adopt to mitigate the impact of bias in artificial intelligence systems.
1. Diversifying Data Sources
Data is the foundation of AI systems. It is crucial to ensure that the data used in training these systems accurately represents diverse populations. The lack of diversity in data sources can result in biased outcomes as it fails to capture the experiences and perspectives of marginalized groups.
To prevent this issue, companies should actively seek out diverse datasets from different sources while building their AI models. Moreover, they must regularly review their datasets for any inherent bias.
2. Explicitly Addressing Biases during Model Development
AI developers must take proactive measures to identify potential biases during every stage of model development. This includes understanding different demographic groups’ needs and ensuring that no group is unjustly excluded or discriminated against by an AI system’s design or decision-making processes.
This approach requires a diverse team with varied backgrounds and perspectives to identify biases that may go unnoticed by a homogenous team.
3. Regularly Auditing AI Systems
Just like any other technology, AI systems must undergo regular audits to identify potential sources of bias. This process involves analyzing data inputs and outputs to detect patterns of discrimination and making necessary adjustments to the system’s algorithms.
These audits not only help in identifying and mitigating existing biases but also ensure ongoing monitoring of AI systems for future bias prevention.
4. Promoting Transparency in AI Decision-Making
To address concerns around biased decision-making, AI models must be transparent about how they reach their decisions. This means explaining how the algorithm works, which factors it considers, and what data it uses during decision-making processes.
Transparency is key when addressing potential biases as it allows for greater scrutiny and understanding of an AI model’s outcomes. It also enables individuals or organizations who have been negatively impacted by biased decisions to hold the system accountable.
Moving Towards Ethical and Fair AI
As artificial intelligence (AI) becomes increasingly integrated into our daily lives, it has become imperative to address the issue of bias in AI systems. While these systems are meant to be objective and impartial, studies have shown that they can inherit biases from their human creators and datasets. This has led to concerns about the fairness and ethical implications of AI technology.
In recent years, there has been a growing recognition of the importance of addressing bias in AI. In 2018, Google’s CEO Sundar Pichai stated that “we must be clear-eyed about how we [use] technology.” He emphasized the need for transparent and accountable development of AI systems that prioritize fairness and responsible decision-making. This sentiment has been echoed by other tech giants such as Microsoft and IBM.
One major concern with biased AI is its potential impact on various aspects of our society, including employment, finance, healthcare, criminal justice, and more. For instance, biased hiring algorithms could perpetuate discrimination against certain demographic groups by automatically filtering out qualified candidates from marginalized communities. Biased loan approval algorithms could deny loans to individuals based on irrelevant factors like race or gender instead of their creditworthiness. These are just a few examples of how biased AI can reinforce existing societal inequalities.
Conclusion
As we continue to rely on and integrate AI into our daily lives, it is imperative that we confront the silent bias that exists within this technology. By educating ourselves and actively working towards more diverse representation in AI development, we can combat discriminatory algorithms and ensure a fairer future for all. Let us strive for diversity and inclusivity in every aspect of our society, including in the crucial technologies that shape our world. Together, we can create a more equitable future for all.
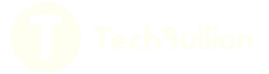