-
How Self-Driving Cars Use Data and Technology
Self-driving cars rely on a combination of sensors, machine learning algorithms, and vast amounts of real-time data to navigate the roads. These vehicles utilize:
- LiDAR and radar sensors to detect obstacles and measure distances.
- Cameras to identify traffic signs, pedestrians, and other vehicles.
- Artificial intelligence (AI) to process data and make split-second driving decisions.
- GPS and mapping technologies to follow routes and recognize road layouts.
-
Common Causes of Self-Driving Car Accidents
Despite technological advancements, self-driving cars still face challenges that can lead to accidents. Some common causes include:
- Sensor Failures: Malfunctions in LiDAR, cameras, or radar can result in misinterpretation of obstacles.
- Software Errors: Inaccurate AI predictions or bugs in the system can cause miscalculations.
- Human Interventions: Many AVs operate in semi-autonomous mode, requiring human drivers to take over in emergencies. Delayed reactions can lead to collisions.
- Environmental Factors: Adverse weather conditions, such as heavy rain or fog, can interfere with sensor readings.
-
The Role of Data in Accident Analysis
When a self-driving car is involved in an accident, data plays a pivotal role in determining the cause and liability. The vehicle’s black box records crucial information, including:
- Speed and braking patterns before impact.
- Sensor readings and AI decisions at the time of the accident.
- Communication logs between the AV system and external infrastructure.
This data is invaluable for improving future AV designs and addressing vulnerabilities in the system. Understanding how these elements function can also help new drivers learn about modern road safety.
-
Legal and Ethical Challenges
As self-driving technology evolves, legal and ethical issues surrounding liability and regulations become more complex. Key concerns include:
- Determining Fault: Is the manufacturer, software developer, or human driver responsible for an accident?
- Privacy Concerns: AVs collect extensive data on driving habits, raising questions about data security.
- Regulatory Adaptation: Laws governing traditional vehicles must evolve to accommodate autonomous technology.
-
Improving Self-Driving Car Safety
To reduce accidents and enhance safety, automakers and researchers focus on:
- Enhancing AI learning models to improve decision-making in real-world scenarios.
- Integrating advanced fail-safe mechanisms to handle sensor failures or unexpected road conditions.
- Collaborating with policymakers to establish clear safety guidelines for AV operation.
-
Data Gaps and Misinterpretation
Autonomous vehicles struggle with “edge cases”—rare scenarios outside their training data. Consider a bicycle carrying an oversized object or a faded stop sign obscured by foliage. Without prior exposure, the AI may fail to react appropriately.
In 2018, Uber’s self-driving test car fatally struck a pedestrian because its software dismissed her as a “false positive.” This tragedy underscores the dangers of over-reliance on imperfect data. Preparing for unexpected scenarios is as crucial for AI as it is for human drivers, which is why DMV permit tests emphasize defensive driving principles.
-
The Human-Machine Interface
While self-driving cars reduce human error, they require drivers to stay alert. Many accidents occur when users overestimate the technology’s capabilities. For instance, Tesla’s Autopilot mandates driver supervision, yet complacency leads to delayed reactions in emergencies.
This duality reinforces why foundational driving skills—tested in permits tests are irreplaceable. Knowing when to override automated systems could prevent collisions.
-
Regulatory and Ethical Hurdles
Data privacy, liability, and standardization remain unresolved. Who’s responsible if a car’s AI chooses between two dangerous outcomes? How often should software be updated? Without unified regulations, inconsistencies in safety protocols persist.
Also See: How Telematics and Video Evidence Are Revolutionizing Liability Assessment in Car Accidents
Conclusion
Data and technology are double-edged swords in autonomous driving. While they offer groundbreaking safety potential, their limitations demand rigorous testing, ethical frameworks, and ongoing human education. As the industry evolves, blending cutting-edge innovation with time-tested driving knowledge will be key to minimizing accidents and building public trust.
By understanding both the capabilities and risks of self-driving tech, we can steer toward a future where human and machine collaboration makes roads safer for everyone.
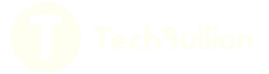