Introduction:
Welcome to the realm of data science, where numbers unlock the secrets of hidden patterns and illuminate the path to predicting future investment trends. In today’s rapidly changing financial landscape, mastering the art of deciphering data can be the key to distinguishing between successful investments and missed opportunities. This article explores the captivating world of data-driven investment predictions, revealing how data science is reshaping wealth creation strategies through algorithms, machine learning, and big data analytics.
The Role of Data Science in Investment Trends:
Data science has become an indispensable tool for individuals, businesses, and governments alike. In an era marked by the constant generation of vast datasets, the ability to collect, analyze, and interpret this information is vital for making informed decisions. In the realm of investments, data science has emerged as a game-changer, offering valuable insights into market trends and empowering investors to make profitable choices.
So, what exactly is data science? It’s a multidisciplinary field that blends statistics, mathematics, computer science, and domain expertise to extract meaningful insights from extensive datasets. Data scientists employ various tools and techniques, including machine learning algorithms and statistical models, to scrutinize data and identify patterns or trends that guide decision-making.
The role of data science in investment trends cannot be overstated. Traditionally, investment decisions relied on gut instincts or expert opinions. However, the rise of big data and technological advancements now grants investors access to a wealth of information capable of influencing their decision-making processes.
Data science techniques, such as predictive modeling and sentiment analysis, enable investors to analyze real-time market trends and make more precise predictions about future performance. This empowers them to optimize their portfolios for maximum returns.
One critical area where data science is revolutionizing investment trends is portfolio management. By analyzing historical financial market data alongside real-time economic indicators, such as interest rates or inflation rates, data scientists develop advanced models to optimize asset allocation strategies for superior risk-adjusted returns.
Understanding Key Data Science Concepts and Methods:
Data science has cemented its role in various industries, including finance and investment. It leverages statistical analysis, programming, and machine learning techniques to extract valuable insights from vast datasets. This section delves into key concepts and methods central to data science’s ability to predict investment trends.
1. Data Collection and Preprocessing:
Initiating any data science project commences with the collection of pertinent data. This may encompass financial market data (e.g., stock prices), economic indicators, company financial statements, and news articles. The quality and quantity of data profoundly influence prediction accuracy.
Once data is gathered, preprocessing is essential before feeding it into analytical models. This entails cleaning the data by eliminating irrelevant or duplicate entries, addressing missing values, and converting it into a usable format.
2. Exploratory Data Analysis (EDA):
Exploratory Data Analysis plays a pivotal role in understanding dataset patterns and relationships. EDA employs descriptive statistics and visualization techniques to uncover data distribution, outliers, variable correlations, and other trends.
EDA aids in identifying underlying patterns or relationships that can inform investment trend predictions. For example, it might unveil a strong correlation between a company’s stock price and its quarterly earnings report.
3. Predictive Modeling:
Predictive modeling involves constructing mathematical or statistical models capable of making predictions based on historical data patterns. These models leverage algorithms to forecast future trends or outcomes, making them an invaluable tool in predicting investment trends.
The Importance of Data Collection and Analysis in Investment Prediction:
Data science has transformed the approach to various industries, including investing. With the immense volume of data readily available, the significance of utilizing data collection and analysis techniques for predicting investment trends cannot be overstated. This section explores the crucial role of data collection and analysis in investment prediction.
Accurate and timely predictions are paramount for successful investments. Data collection plays a pivotal role by providing vital information on market trends, consumer behavior, economic indicators, and other factors influencing investments. Data sources may encompass financial reports, company performance data, news articles, social media platforms, and more.
One of the key advantages of collecting extensive data is the ability to discern patterns and correlations that would otherwise remain elusive. By analyzing historical market trends and patterns through statistical methods and algorithms, valuable insights into how past events or factors influenced investments are gained. This knowledge forms the basis for informed decisions when predicting future investment trends.
Moreover, data analysis efficiency has soared with technological advancements and machine learning algorithms. These algorithms swiftly process vast data volumes while identifying intricate relationships among variables. Consequently, investors can make predictions with higher accuracy levels compared to traditional methods.
Another critical aspect of employing data collection and analysis for investment prediction is risk management. Continuous data collection on relevant market data and tracking evolving trends allows for effective risk assessment and
Successful Applications of Data Science in Investment Trends – Case Studies:
Examining real-world case studies provides concrete examples of data science’s effectiveness in predicting market movements and making profitable investment decisions. This section explores noteworthy case studies showcasing data science’s power in investment.
1. BlackRock’s Aladdin System:
BlackRock, one of the world’s largest investment management firms, oversees assets exceeding $7 trillion. Since 1989, the company has employed its proprietary system, Aladdin (Asset Liability and Debt and Derivative Investment Network), to manage investments.
Aladdin utilizes advanced data analytics techniques, including machine learning and natural language processing, to gather data from sources like market news, social media sentiment, economic indicators, and financial reports. This extensive data undergoes analysis to identify patterns and trends that could impact investment decisions.
The efficacy of Aladdin became evident during the 2008 financial crisis when it successfully predicted the collapse of mortgage-backed securities, helping BlackRock avoid substantial losses. Today, Aladdin remains a valuable tool for making data-driven investment decisions.
2. Renaissance Technologies’ Medallion Fund:
Renaissance Technologies LLC, a renowned hedge fund, employs sophisticated quantitative models in its trading strategies. Its flagship fund, the Medallion Fund, has consistently delivered remarkable returns to investors since its inception in 198
Challenges and Limitations of Data Science in Investment Trends:
While data science offers powerful tools for investment prediction, like any technology, it comes with challenges and limitations. This section explores potential obstacles investors may encounter when employing data science in investment trends.
1. Data Quality:
Data accuracy and reliability are paramount in data-driven analyses and predictions. In investment trends, the quality of data used significantly affects prediction accuracy. Accurate and relevant data availability can be challenging due to the dynamic and complex nature of financial markets. Furthermore, discrepancies in historical market data may influence prediction models.
2. Limited Historical Data:
Financial markets have a relatively limited historical context compared to other industries like healthcare or retail. This shortage of historical market data can hinder the accurate training of complex algorithms. Consequently, prediction models may lack reliability.
3. Biased Data:
Utilizing large datasets for predicting investment trends can lead to biased results if the dataset itself is biased. For instance, if historical market data predominantly represents specific types of investments or industries, predictions may favor those areas and overlook others.
4. Overfitting:
Future Outlook: Data Science’s Impact on the Investment Industry:
The investment industry has perpetually relied on data and analytics. Nevertheless, data science’s rise, fueled by technological advancements and the abundance of big data, has elevated its role in shaping investment decisions. Data science melds statistics, computer science, and domain expertise to extract insights and value from extensive datasets, ushering in a new era in the investment sector. In recent times, data science has emerged as a game-changer, providing novel tools and techniques for analyzing market trends, predicting future performance, and facilitating informed investment choices. How, then, is data science reshaping the investment industry? Here are key areas where its impact is palpable:
1. Predictive Analytics:
Data science’s most substantial contribution to the investment industry is predictive analytics. Through machine learning algorithms and extensive historical financial data, investors can accurately forecast stock prices, market trends, and shifts in consumer behavior. This equips them to detect opportunities and preemptively manage risks.
2. Risk Management:
In an environment marked by unpredictable economic conditions and dynamic markets, risk management is pivotal to successful investing. Data science empowers investors to pinpoint patterns in historical market behavior, facilitating precise risk assessment for various investments. This leads to informed decisions rooted in robust statistical models rather than intuition or guesswork.
3. Automated Trading:
Data science has paved the way for automated trading systems that rely on artificial intelligence (AI) and machine learning algorithms. These systems can analyze vast datasets and market conditions in real time, executing buy and sell orders with remarkable precision and speed. By removing human emotions from the equation, automated trading minimizes the risk of impulsive decisions and capitalizes on opportunities that might elude human traders. Additionally, automated trading systems can continuously learn and adapt to evolving market dynamics, ensuring they remain effective even in changing conditions.
Tips for Integrating Data Science into Your Investment Strategy:
Data science’s transformative potential has made it an integral component of modern investment strategies. However, seamlessly integrating data science into your investment approach can be a complex endeavor. To help harness data science’s power in your investments, consider the following tips:
1. Define Your Investment Objectives:
Before infusing data science into your investment strategy, clarify your investment goals and objectives. While data science provides invaluable insights, it should complement, not replace, human judgment. Having a clear investment vision ensures you can effectively leverage data science to achieve your goals.
2. Identify Relevant Datasets:
The quality of data profoundly influences the reliability of your analysis. As an investor, it’s vital to identify and gather datasets that align with your investment objectives. This may encompass financial market data, economic indicators, company financials, social media sentiment analysis, or other pertinent information.
3. Employ Diverse Analytical Techniques:
Data science offers a myriad of analytical techniques, including regression analysis, machine learning algorithms, natural language processing (NLP), and network analysis. The choice of technique depends on the type and volume of available data. Employing diverse analytical methods provides a comprehensive view and deeper insights into investment trends.
Conclusion: Leveraging Data Science for Investment Success:
In today’s dynamic investment landscape, predicting and capitalizing on market trends is a formidable challenge. Traditional analysis and forecasting methods often fall short in the face of intricate economic factors. However, data science has emerged as a formidable ally, granting investors access to powerful tools capable of unraveling market trends and potentially leading to successful investments.
Data science, an interdisciplinary field encompassing statistics, machine learning, and computer science, excels in extracting insights from vast datasets. By leveraging this approach, investors gain a deeper understanding of market dynamics, identify potential opportunities, and make informed investment choices.
One of data science’s primary advantages in investing is its ability to rapidly process extensive data, including financial data, news reports, social media activity, and economic indicators. Through advanced algorithms and predictive models, data scientists analyze this diverse information to detect patterns or anomalies influencing future market movements.
Furthermore, data science enables objective decision-making, mitigating the impact of emotions and biases. Algorithms minimize human error and subjectivity, fostering systematic evaluation of investment opportunities based on reliable data rather than intuition.
Read More
The Future of Data Science: Trends and Predictions
Exploring Algorithms, Models, and Visualization Techniques Of Data Science
Data Science vs. Artificial Intelligence: Understanding the Distinctions
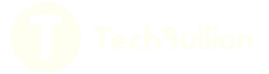