Information is a powerful thing, but too much data can be overwhelming if you don’t have the right tools to manage and interpret it.
In real estate, being deluged with data points is a common problem, but with the principles and practices of data science it’s possible to turn this situation to your advantage.
Here are just some examples of data science and real estate combining to great effect.
Providing accurate property valuations
Being precise when valuing real estate is tricky, and requires years of experience as well as lots of local knowledge to get right. Even then, mistakes can be made, which is bad for clients and worse for your agency’s reputation.
Thankfully there are large data sets relating to property transactions in each region, and this provides property price indices upon which future valuations can be based.
This goes much deeper than merely analyzing historical sale prices and finding an average, of course. And there are comprehensive platforms like northspyre.com which are able to quash data disorganization and improve visibility of key insights, in turn allowing you to make informed decisions rather than taking unfounded risks.
In turn, automation comes into play and means that valuations can be generated entirely by software. This is what underpins many modern property platforms.
Predicting market fluctuations
Real estate isn’t just about what a property is worth today, but how the market will change going forward. Being able to predict upticks in values or potential setbacks allows realtors, as well as owners, to plot out their moves and prepare for whatever they might face in the months and years to come.
Here, more data sets are added to the mix, including broader info relating to the current economic state of play. Everything from interest rates on mortgages to levels of unemployment and even exchange rates on foreign currencies can feed into projections for the real estate market.
Identifying patterns to single out points of divergence
Data science is all about pattern recognition, and once a pattern has been found, it’s up to the experts to determine what this might mean and how this should influence decision-making according to the context.
For real estate, this requires the ability to highlight divergences to get a better understanding of how certain assets are to be interpreted.
To put this in a simpler way, even within a single neighborhood there will be fluctuations in property values and salability, according to the category of property that’s being considered. High end apartments might sit separate from affordable housing that’s just across the street when it comes to pricing, even if the location is virtually identical.
Commercial properties are another distinct subsection of the market which data science can set apart and assess.
So-called cluster analysis means that models for each category of property are developed, enabling realtors to better adapt their tactics for courting sellers and buyers alike, without wasting time.
Scrutinizing geographic data
Location matters more than almost anything else in the world of realty, and once again there’s a torrent of information to sift through; so much, in fact, that it’s impossible to handle this manually.
Data science tools and strategies mean that it’s possible to look into things like public amenities, crime rates, transport links, average household incomes, and so on. Both residential and commercial real estate can then be dealt with using a holistic and informed overview.
Final thoughts
Data science is so ingrained in modern real estate that most people won’t even realize that it’s being used, because of how seamlessly it assists them.
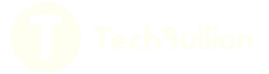