The integration of Natural Language Querying (NLQ) into Security Information and Event Management (SIEM) systems represents a significant leap forward in cybersecurity operations. This technology aims to simplify interactions between security analysts and complex datasets, thereby facilitating more efficient threat detection and response. Yeshwanth Vasa, with over five years of experience in Artificial Intelligence (AI) and Machine Learning (ML) within the cybersecurity domain, has conducted extensive research and made substantial contributions in exploring how NLQ is transforming SIEM systems and bridging the gap between security analysts and intricate data environments.
The Role of Natural Language Querying in SIEM Systems
“NLQ enables security analysts to interact with SIEM systems using everyday language, bypassing the need for complex query languages”. This capability is particularly valuable in environments where rapid data interpretation is essential. Yeshwanth Vasa’s research highlights how leveraging NLQ allows analysts to swiftly extract relevant information from extensive datasets, thereby enhancing their effectiveness in responding to security incidents.
Enhancements through AI and ML
“The integration of AI and ML into SIEM systems has revolutionized the processing and analysis of security data. AI-driven platforms, such as those employing Large Language Models (LLMs) like OpenAI’s GPT, can automate complex processes, provide deeper insights, and improve overall efficiency in security operations”. Yeshwanth Vasa’s work has shown how these models can automate log analysis, reduce false positives, and generate actionable threat intelligence. His research demonstrates that this automation not only saves time but also enables analysts to focus on critical tasks, such as incident response and threat mitigation.
Practical Applications of NLQ in SIEM
- Automated Log Analysis: According to Yeshwanth Vasa’s findings, “LLMs can analyze large volumes of logs to identify patterns and anomalies that traditional methods might overlook. This reduces false positives and emphasizes genuine security incidents, thereby improving threat detection accuracy”.
- Threat Intelligence Generation: Vasa’s research indicates that by analyzing historical and current security data,”LLMs can provide insights into potential vulnerabilities and attack patterns. This helps organizations proactively address security weaknesses”.
- Incident Response Automation: Yeshwanth Vasa has demonstrated how LLMs can automate parts of the incident response process, such as generating scripts or workflows based on detected threats. This capability streamlines the response to security incidents and enhances the overall security posture of an organization.
Challenges and Considerations
While NLQ offers numerous benefits, it also presents challenges. The accuracy of NLQ systems relies heavily on the underlying AI models and their ability to process and understand natural language accurately. Yeshwanth Vasa’s work emphasizes the importance of fine-tuning these models to handle cybersecurity-specific data for optimal performance. Additionally, organizations must ensure that their SIEM systems are capable of integrating with NLQ technologies and that security analysts are trained to utilize these tools effectively.
Future Directions
The future of NLQ in SIEM systems is tied to the ongoing advancement of AI technologies and their integration into cybersecurity operations. As AI models evolve, they will offer even greater capabilities for automating threat detection and response. Yeshwanth Vasa’s insights into the development of more intuitive NLQ interfaces will make it easier for security analysts to interact with complex data environments, ultimately enhancing organizational security.
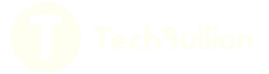