Artificial intelligence isn’t just a buzzword anymore; it’s a tool that’s changing how businesses work. When AI in cloud systems is woven into the core of a cloud strategy, companies can see real, game-changing results.
But too many businesses treat AI integration like an afterthought, tackling it onto existing systems. This limits what AI can do and often creates more headaches than solutions.
This blog dives into what happens when AI in cloud is fully built into a cloud strategy. It covers why superficial approaches fall short, the perks of thoughtful AI integration, real-world examples, and clear steps to make it happen.
The “Add-On” Trap
Slapping AI in cloud onto a cloud strategy without much thought is a recipe for trouble. Many organizations try to plug AI integration into their existing setups without rethinking how data flows or how systems connect.
This creates isolated AI tools that can’t tap into the full power of cloud resources. Picture a company using a machine learning model to analyze customer behavior but not linking it to live data. The result? Insights that are outdated before they’re even used.
This approach also hits the wallet hard. Piecemeal AI integration often needs expensive fixes to work with cloud systems, wasting time and money. Even worse, it can make teams doubt intelligent automation when they deal with glitchy tools or clunky workflows.
A cloud strategy that puts AI in cloud first avoids these traps, keeping data flowing smoothly and systems ready to grow.
Cloud-First AI Integration
A cloud-first mindset makes AI integration a core part of a cloud strategy development, building systems where intelligent automation can shine. It’s about creating infrastructure that handles AI’s big needs, think fast computing, instant data access, and tight security.
Take major cloud platforms like AWS, Azure, or Google Cloud. They offer tools like managed machine learning setups or high-powered GPU instances that make deploying AI in cloud straightforward.
In this kind of cloud strategy, AI in cloud feels like a natural fit. Data storage systems are built to fuel intelligent automation tools, letting things like predictive analytics or language processing happen in real time.
It also brings teams together. Data experts, engineers, and business analysts can all work on AI models in the same cloud setup, creating a unified system where AI integration sparks efficiency and new ideas.
Strategic Advantages and Risks
Bringing AI in cloud into a cloud strategy opens up big wins, but it’s not without challenges that need careful handling.
Advantages
- Scalability– Cloud systems let intelligent automation grow easily, handling bigger data loads or complex models without needing a complete overhaul.
- Cost Savings– With cloud’s pay-as-you-go model, AI integration becomes affordable, even for smaller businesses that couldn’t otherwise invest in high-end tech.
- Speed– A cloud strategy with AI in cloud lets companies test and roll out new ideas quickly, staying ahead of the curve.
Risks
- Data Privacy– Storing sensitive info in the cloud for intelligent automation can raise red flags with laws like GDPR or CCPA, requiring strict compliance measures.
- Vendor Dependence– Leaning too heavily on one cloud provider’s AI tool can box a company in, making it tough to switch later.
- Skill Shortages– Pulling off AI integration needs people who know both AI and cloud systems inside out, which might mean training or new hires.
Managing these risks ensures a cloud strategy gets the most out of AI in cloud without stumbling.
Use Cases Across Sectors
The power of AI integration in a cloud strategy shows up across different fields, proving its value through intelligent automation.
In healthcare, hospitals use AI in cloud to dig into patient data as it comes in, spotting risks like potential readmissions. Cloud-based AI can process scans or health records, catching issues faster while keeping data secure.
Retail businesses rely on intelligent automation to make shopping personal. Cloud-powered recommendation systems study browsing habits, suggesting products that drive more sales.
In manufacturing, AI in cloud keeps supply chains humming. Cloud-based models analyze machine sensor data to predict when equipment might fail, cutting downtime and saving costs.
These cases show how a cloud strategy with strong AI integration delivers real results, from saving money to making customers happier.
Steps to Implementation
Shifting to a cloud strategy that centers AI in cloud takes planning. Here’s a roadmap for successful AI integration–
- Check the Foundation– Look at current cloud systems to spot weak points in data access, computing power, or security that could slow down intelligent automation.
- Set Clear Goals– Decide what AI integration should achieve, like better customer loyalty or automating routine tasks, to steer tech choices.
- Pick the Right Tools– Choose a cloud platform with solid AI features that match the company’s needs and comply with regulations.
- Build Expertise– Train staff or hire specialists in cloud systems and intelligent automation to lead the charge and keep things running.
- Test and Grow– Start with small AI in cloud projects to learn what works, then scale up based on results and insights.
- Keep Improving– Track performance data to fine-tune AI integration, ensuring it stays in sync with business goals.
Following these steps helps organizations root AI in cloud firmly in their cloud strategy, setting the stage for lasting innovation.
Conclusion
When AI integration becomes a cornerstone of a cloud strategy, businesses tap into something truly powerful.
A cloud-first approach makes intelligent automation feel effortless, delivering flexibility, cost savings, and the ability to move fast.
Challenges like data privacy or finding skilled talent need attention, but the rewards are worth it.
From hospitals to online stores, AI in cloud is transforming how industries operate. Companies that commit to this shift today can lead the way in tomorrow’s tech-driven world.
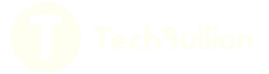