The integration of Artificial Intelligence (AI) and Machine Learning (ML) has rapidly shifted from a futuristic concept to a fundamental driver of innovation within the enterprise software sector.
Its economic significance is underscored by forecasts predicting AI could add over $15 trillion to the global economy annually by 2030 and potentially between $15.5 trillion and $22.9 trillion annually by 2040.
Current adoption rates reflect this momentum, with studies suggesting that 50% to 60% of organizations are already utilizing AI-centric tools.
Lohitaksh Yogi, a Principal Product Manager, has recently transitioned from Adobe to ServiceNow. Both Adobe and ServiceNow have been at the forefront of integrating AI into their operations for over a decade. They utilize AI not just as an additional feature, but as a fundamental element to enhance creative processes and deliver personalized customer experiences at scale, all while maintaining a strong commitment to responsible AI development.
Lohitaksh has been instrumental in driving significant AI-driven innovations at Adobe and continues to do so at ServiceNow. This article highlights his role as a visionary AI product manager, shaping the future of these companies’ offerings.
Lohitaksh’s background includes a Bachelor’s degree in Computer Science and a Master’s in Data Science and Machine Learning, complemented by Stanford’s programs.
Stanford’s programs emphasize the full product lifecycle, strategic thinking, and understanding user needs, with specialized tracks now emerging in AI and Generative AI for product innovation, reflecting the skills Yogi employs. His contributions have earned him the Gold Globee Award, the 2025 Global Recognition Award, and other accolades in the AI field, which are noted for acknowledging exceptional vision, innovation, and impact with credibility.
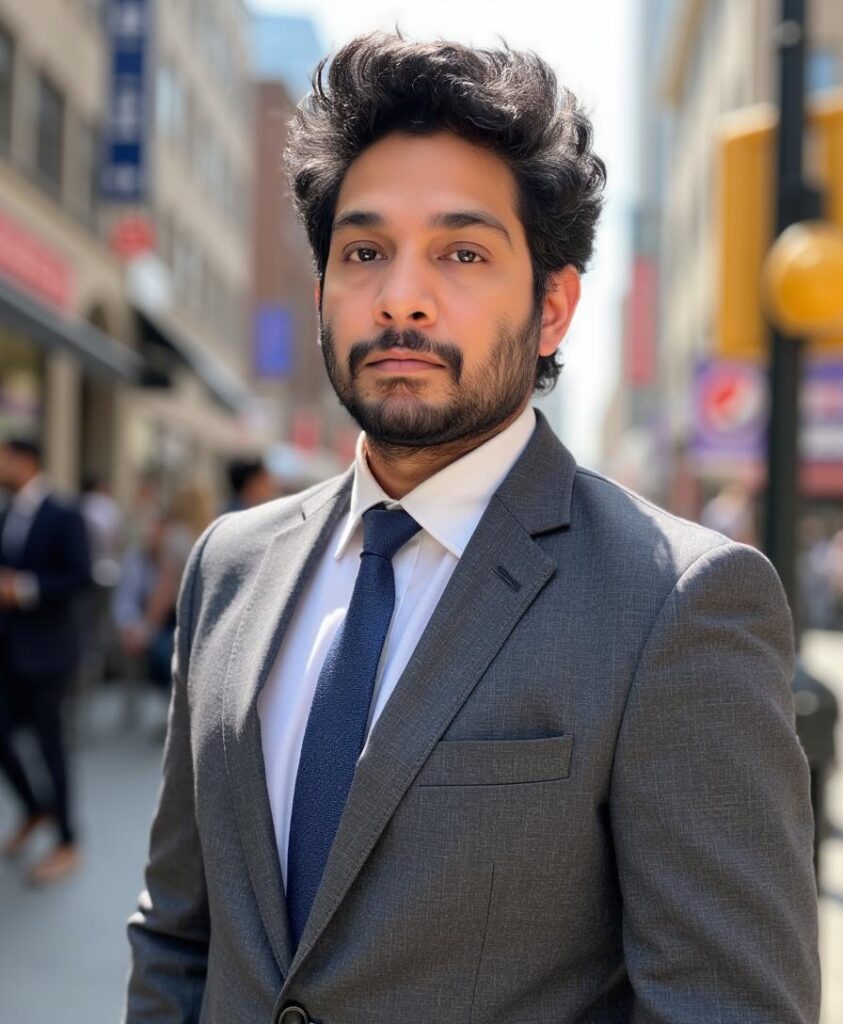
Lohitaksh Yogi
Yogi’s role demands a unique synthesis of deep technical knowledge and strategic product management capabilities. His core product management skills encompass Strategic Vision and Planning, User-Centric Design, Business Acumen, Leadership and Collaboration, Critical Decision Making, and Problem-Solving.
This is coupled with extensive expertise in AI and ML, including Natural Language Processing (NLP), Natural Language Understanding (NLU), Large Language Models (LLMs), multimodal models such as Diffusion and DALL-E, Retrieval-Augmented Generation (RAG), and advanced LLM training techniques like fine-tuning and other advanced techniques.
He leverages this effective combination to spearhead initiatives, including the Now Assist Platform, Adobe Assistant AI, the Generative Models Platform, and notably, the Virtual Agent enterprise platform. These efforts directly influence the business revenue and customer engagement for both Adobe and ServiceNow.
Shaping customer interaction future
Yogi’s path into AI product leadership began with a foundation in data science, where he cultivated an interest in AI models and machine learning. His academic background, including a Master’s degree in machine learning and involvement in clinical NLP research projects, provided a deep understanding of AI’s potential to enhance customer experiences and optimize workflows.
This transition from a technical specialist to a strategic product leader mirrors an essential trend in the technology industry, where individuals possessing both deep technical understanding and product management acumen are increasingly vital for navigating the complexities of AI development.
Forrester highlights that AI product managers need specific competencies in AI/ML technologies, data science, and model performance alongside traditional PM skills, a profile Yogi embodies.
His motivation stems from recognizing the “immense potential these technologies have to revolutionize customer interactions and drive business growth”.
This insight is validated by the significant expansion of the AI market, particularly in conversational AI, which was valued at $10.57 billion in 2023 and is projected to reach $97.64 billion by 2033, growing at a CAGR of 24.9%.
Statistics indicate high adoption rates, with nearly 80% of customer-facing virtual assistants used for customer service and AI adoption potentially boosting business revenue by 6% to 10% on average.
Yogi stated, “As a product manager for a Conversational AI, search, and Machine Learning platform, I have led initiatives that have driven strategic vision, enhanced customer experience, and fueled business growth, resulting in increased revenue, customer acquisition, self-serve rates, and customer satisfaction”.
An exemplary instance of Yogi’s methodology is reflected in his vision for the Virtual Agent enterprise platform, which he led at Adobe and currently advances at ServiceNow. He described this vision clearly: “My vision for the Virtual Agent enterprise platform is to revolutionize customer interactions by leveraging advanced AI technologies to provide seamless, efficient, and personalized support. This platform integrates cutting-edge generative AI models, LLMs, deep learning, machine learning algorithms, and natural language processing (NLP) to deliver superior chatbot and voice bot experiences”.
Yogi’s work on the Virtual Agent platform served as a crucial precursor or validation for this larger strategic direction within Assistant AI field.
His approach emphasizes creating natural, human-like interactions, supporting multimodal inputs (text, voice, image), and ensuring the responsible use of AI through transparency, fairness, and accountability—principles vital for building trust in increasingly sophisticated AI systems.
Harmonizing technology, users, and business objectives
A critical responsibility for any AI product manager lies in navigating the intricate balance between cutting-edge technical feasibility, genuine user needs, and overarching business goals. This challenge is central to the role, requiring a unique blend of technical depth and strategic foresight.
Lohitaksh addresses this through a multi-faceted strategy that ensures AI innovations are not just technically impressive but also align with customer expectations and drive tangible business impact. His approach starts with a deep focus on the user. “Firstly, I prioritize understanding the needs and pain points of our users,” Yogi explained.
“This involves conducting thorough user research, gathering feedback, and analyzing user behavior to identify key areas where AI can add value. By focusing on user-centric design, I ensure that the solutions we develop are tailored to meet the specific needs of our customers”. This user-centricity is crucial, as research indicates that personalized experiences significantly improve customer engagement and purchasing likelihood.
Complementing this user focus is a rigorous assessment of technical feasibility. Lohitaksh Yogi leverages his own technical background to evaluate various AI models, including LLMs and generative AI, understanding their capabilities and limitations.
This allows for informed decisions on model integration and performance optimization through advanced techniques like pre-training, fine-tuning, and RLHF, which then guides the LLM during reinforcement learning to produce outputs more aligned with human preferences, enhancing helpfulness and safety.
Furthermore, Lohitaksh Yogi emphasizes the practicalities of enterprise deployment, ensuring AI solutions integrate seamlessly with existing systems and third-party channels, facilitating easier adoption and reducing implementation friction, a key factor noted in enterprise AI success.
Crucially, Yogi’s framework explicitly integrates business value and ethical considerations from the outset. “To align AI innovations with business goals, I focus on delivering immediate business value. This includes providing instant ROI with guided setup, prebuilt conversations, integrations, and configuration tools,” he stated.
“Furthermore, I ensure that our AI solutions adhere to ethical AI principles. Establishing robust guidelines for ethical AI use, including monitoring and auditing processes, helps build trust and ensures compliance with industry standards”.
This focus on immediate value connects directly to the measurable benefits reported for tools like the AI Assistant, which demonstrably improve productivity and efficiency.
The proactive integration of ethical AI principles addresses growing concerns about bias, fairness, and transparency, positioning ethics not merely as a compliance requirement but as a fundamental component of building trustworthy and sustainable AI products essential for long-term business success and user acceptance. This entire process is iterative, involving continuous refinement based on performance data and user feedback to ensure ongoing alignment and effectiveness.
Triumphs and hurdles in building enterprise conversational AI
Providing a concrete example of his work, Yogi detailed his leadership in developing the Virtual Agent enterprise platform at Adobe and ServiceNow. This platform aims to fundamentally change customer interactions by delivering seamless, efficient, and personalized support through advanced AI, incorporating generative models, LLMs, deep learning, and NLP.
This initiative acts as a practical implementation of the previously outlined vision, aligning with the company’s ongoing strategic investments in agentic frameworks.
The development journey, however, was not without significant obstacles. A primary challenge involved ensuring the accuracy and contextual relevance of AI-generated responses across different interaction modes, including text and voice.
Lohitaksh Yogi elaborated on the technical approach: “One of the primary challenges was ensuring that the AI-powered response generation was accurate and contextually relevant across various interaction modes (text, voice, phone). To address this, we incorporated state-of-the-art generative models such as GPT, BERT, RoBERTa and Multimodal generative modals such as like Diffusion, VAE, GAN, and DALL-E, and employed advanced training techniques like pre-training, fine-tuning, and reinforcement learning from human feedback (RLHF) to continuously improve model accuracy and relevance”.
This highlights a sophisticated strategy, combining established NLP models known for understanding context (like BERT) with powerful generative models (like GPT) and advanced multimodal capabilities, further refined by advanced techniques to mitigate common LLM issues like hallucination.
This layered technical approach suggests a nuanced understanding that building high-performing conversational AI requires more than just deploying a single large model.
Beyond technical accuracy, ethical considerations and compliance were paramount. “Another significant challenge was ensuring ethical AI and compliance,” Yogi noted.
“Establishing robust guidelines for the ethical use of AI, including monitoring and auditing processes, was crucial to building trust and ensuring compliance with industry standards. This focus on transparency, fairness, and accountability helped maintain the integrity of our AI technologies”. This aligns with the critical need for strong ethical frameworks in enterprise AI.
Additionally, the team tackled the complexity of “providing multilingual and multimodal support to ensure accessibility and convenience for a global audience,” supporting over 10 languages and various interaction modes including text, voice, and potentially image analysis, reflecting the growing importance of multimodal AI capabilities in enterprise applications.
Yogi noted the challenge of implementing solutions within a complex enterprise environment. He described the “seamless integration and deployment of the platform” as an important milestone.
Smooth integration with the existing company’s ecosystem, web pages, and third-party channels, along with tools for rapid configuration, was crucial. This highlights the significant difficulty of operationalizing AI.
Although model performance and ethics are important, effectively integrating the solution into existing workflows is what truly adds value and encourages adoption. This success in integration likely provided valuable insights for broader platform strategies.
Strategies for enterprise-wide deployment and reliability
Transitioning AI capabilities from successful pilot projects or specific product features to enterprise-wide adoption presents a distinct set of complexities. Successfully navigating this scaling process requires more than just technical prowess; it demands a strategic framework that ensures reliability, performance, user acceptance, and alignment with business objectives across the organization.
Lohitaksh Yogi outlined several key strategies employed at Adobe and ServiceNow to manage this intricate process effectively, addressing both technical and non-technical dimensions crucial for large-scale AI deployment.
Central to this strategy is the principle of seamless integration and deployment, a recurring theme underscoring its importance in the enterprise context. “Firstly, seamless integration and deployment are crucial,” Yogi emphasized.
“Ensuring that AI solutions can be easily integrated with existing systems and platforms facilitates out-of-the-box development and reduces setup time”.
This focus on minimizing friction during implementation is vital for encouraging broad adoption. Equally important is designing for usability: “User-friendly design and customization are also essential. Offering an intuitive interface allows users to easily create, import, and manage conversations”.
This holistic approach, addressing technical integration alongside user experience, is critical because scaling AI successfully hinges on managing adoption and demonstrating value across diverse user groups within the enterprise.
Maintaining performance and reliability at scale necessitates continuous technological advancement and rigorous governance. “Advanced AI integration and training techniques are employed to maintain reliability and performance,” Yogi explained, referencing the ongoing use of state-of-the-art models and methods like fine-tuning and RLHF. These techniques help ensure that AI features remain accurate and relevant as they are deployed more widely. Simultaneously, ethical considerations become even more critical at scale are non-negotiable to build and maintain trust and ensure compliance across the enterprise. This suggests that responsible AI is viewed not just as a principle but as a prerequisite for sustainable, large-scale deployment, mitigating the amplified risks associated with widespread use.
Driving adoption also requires a clear demonstration of value. Yogi Stated “Providing immediate business value is key for driving adoption, offering instant ROI with guided setup, prebuilt conversations, integrations, and configuration tools enables users to see the benefits of AI features quickly”. This aligns with both companies focus on quantifiable outcomes, as seen in reports detailing significant time savings and efficiency gains from tools like the AI Assistant.
Finally, the strategy incorporates continuous iteration, ensuring that AI features are regularly measured, evaluated against user satisfaction, and optimized over time to maintain relevance and effectiveness. This comprehensive framework, balancing technology, usability, ethics, value, and ongoing improvement, has proven effective in deploying AI features reliably at scale, contributing to business growth and enhanced customer interactions.
Redefining product development and customer interaction
The emergence of powerful generative AI models and Large Language Models (LLMs) marks a significant inflection point, poised to reshape the landscape of product development and customer interaction fundamentally. Industry analysts predict a substantial impact, with Gartner forecasting that by 2025, 30% of outbound messages from major enterprises will be synthetically generated. Lohitaksh sees these technologies playing a transformative role, moving beyond incremental improvements to enable entirely new ways of engaging users and building products.
According to Lohitaksh, “With the rise of generative AI and large language models (LLMs), AI is set to play a transformative role in shaping the future of product development and customer interactions. AI’s ability to generate accurate, contextually relevant responses across various interaction modes (text, voice, phone) revolutionizes customer support, providing instant, tailored assistance”.
This capability directly addresses the goals of advanced conversational AI platforms and aligns with market demands for immediate, effective customer service.
Beyond support, Lohitaksh envisions AI enhancing the overall user experience through more intuitive design and improved accessibility while continuing to build trust via robust ethical frameworks and compliance measures. The focus remains on delivering tangible benefits, driving efficiency through automation and prebuilt solutions that offer immediate business value, echoing the success factors of existing tools like Now Assist platform and Adobe AI Assistant.
A key aspect of this future, highlighted by Lohitaksh, involves leveraging multimodal AI capabilities. He explained the advantage: “A multimodal assistant can help customers in all modalities, such as text, voice, and images, reducing the overhead of following specific instructions to complete a task.
This flexibility ensures that customers receive the support they need in the most efficient way possible, whether they are typing a query, speaking to a virtual assistant, or uploading an image for analysis”. This points towards more natural, less constrained interactions where AI understands context across various input types, a trend gaining traction in enterprise applications.
ServiceNow and Adobe’s initiatives, such as AI agent control tower and Agent Orchestrator which aims to guide consumers using multimodal interactions, reflect this strategic direction. Lohitaksh’s perspective underscores that while AI’s potential is transformative, its successful future integration hinges on the same practical and ethical foundations vital today: user-centricity, seamless integration, demonstrable value, ethical governance, and continuous iteration.
Crafting AI features for value, revenue, and retention
Successfully monetizing AI-driven products presents a unique challenge: features must not only justify their cost but also demonstrably enhance the user experience, contributing positively to both revenue growth and long-term customer retention. Lohitaksh Yogi identifies several critical factors that must be considered when designing AI features intended for commercial success, emphasizing a strategy rooted in delivering sustained, trustworthy value.
The fundamental principle of this approach is to deliver clear and immediate business value. “Providing immediate business value is essential,” Yogi stated. He emphasized that offering guided setup, prebuilt conversations, integrations, and configuration tools yields instant return on investment by enhancing efficiency and productivity. By focusing on tangible benefits, this strategy helps justify the investment and promotes initial adoption, as evidenced by studies demonstrating significant time savings.
Complementing this is the power of personalization: “Personalization is essential. Tailoring responses based on user history and preferences creates a more engaging and relevant experience, increasing customer satisfaction and retention”. Research confirms that personalized experiences strongly influence purchasing decisions and engagement. Seamless integration into existing workflows further enhances value by reducing friction and making the AI features a natural part of the user’s process.
Trust and reliability form another crucial pillar of Yogi’s monetization strategy. “Ethical AI and compliance build trust,” he stated.
“Establishing robust guidelines for ethical AI use maintains the integrity of AI technologies and fosters long-term user trust. Advanced AI integration and training techniques, such as pre-training, fine-tuning, and reinforcement learning from human feedback (RLHF), continuously improve model accuracy and relevance, ensuring AI features remain effective and reliable”.
This highlights a perspective where ethical practices are not just a cost of doing business but a core component of the value proposition, directly impacting user willingness to pay and remain loyal. Furthermore, the commitment to leveraging advanced AI techniques ensures the features continuously improve, maintaining their effectiveness and justifying their ongoing cost or subscription fee, akin to the add-on model for Acrobat AI Assistant.
Finally, continuous iteration based on performance metrics and user feedback ensures the features adapt and remain valuable over time. This holistic approach suggests a value-based philosophy where AI features must consistently deliver and improve upon demonstrable benefits to succeed commercially, fostering retention through earned trust and ongoing utility.
Lessons in cross-functional collaboration and innovation
Developing and deploying sophisticated AI products necessitates exceptionally close collaboration among diverse, cross-functional teams comprising engineers, researchers, designers, marketers, and executives. The inherent complexity of AI technologies requires bridging gaps between deep technical expertise and broader business strategy, a challenge Lohitaksh Yogi has navigated through specific leadership practices aimed at fostering innovation.
Lohitaksh emphasizes the creation of an environment conducive to shared progress. “Firstly, fostering a collaborative environment is essential,” he explained.
“Encouraging open communication and creating a space where team members feel comfortable sharing their ideas and feedback is crucial. This helps in leveraging the diverse expertise of engineers, researchers, designers, and executives to develop innovative AI solutions”.
This collaborative spirit must be paired with clarity: “Clear communication is another important lesson. Ensuring that everyone is on the same page regarding project goals, timelines, and expectations helps in aligning efforts and avoiding misunderstandings. Regular meetings and updates are vital to keep everyone informed and engaged”. Aligning these efforts with the overarching strategic vision is also critical for motivation and ensuring collective focus on common goals.
Effective AI leadership, in Lohitaksh Yogi’s experience, also involves actively bridging knowledge domains. “Leveraging technical expertise is key to driving AI innovation,” he noted.
“As a product manager with a background in AI and machine learning, I can bridge the gap between technical and business aspects, ensuring that the product roadmap aligns with both user needs and business goals”. This ability to translate between technical possibilities and business requirements is a hallmark of successful AI product management.
Simultaneously, embedding ethical considerations into the team’s culture is presented as a distinct leadership responsibility: “Ethical AI and compliance are important considerations. Establishing robust guidelines for ethical AI use and ensuring that all team members understand and adhere to these principles helps build trust and maintain the integrity of AI technologies”.
This approach moves beyond policy setting to actively cultivate ethical awareness and responsibility within the development process itself, a practice also reflected in his mentorship efforts. Finally, a commitment to continuous iteration and feedback loops ensures that the team remains adaptive and focused on optimizing the AI features based on real-world performance and user satisfaction.
Strategies for sustained innovation
The field of AI is characterized by its relentless pace of evolution, demanding constant vigilance and adaptation from product leaders aiming to keep their organizations at the forefront of innovation.
The rapid emergence of new models, techniques, and applications requires proactive strategies to not only track trends but also integrate meaningful advancements effectively. Lohitaksh employs a multi-pronged approach to ensure that companies remain on the cutting edge, blending external awareness with internal capability building.
Continuous learning forms the foundation. “Continuous Learning: I stay informed about the latest advancements by participating in industry conferences, webinars, and workshops,” Yogi shared.
“Engaging with thought leaders and experts helps me stay updated on trends and best practices. By staying updated on AI trends, reading research papers, and exploring model enhancements, we can evolve and stay ahead of the AI trend”.
This external focus is complemented by efforts to cultivate innovation internally: “Fostering Innovation: Encouraging team members to explore new ideas and experiment with emerging technologies creates an environment where innovation thrives. Regular brainstorming sessions and collaborative projects help identify opportunities for integrating cutting-edge AI solutions”.
This emphasis on building an adaptive internal culture suggests that sustainable innovation relies as much on empowering teams to experiment as it does on absorbing external knowledge.
Staying ahead also means actively leveraging technological advancements and maintaining foundational principles. This includes staying updated on advanced AI integration techniques, understanding the nuances of new methods like Retrieval-Augmented Generation (RAG) or advancements in advanced AI techniques, and incorporating them where appropriate.
Critically, ethical considerations remain central even when exploring new frontiers. “Ethical AI Practices: Establishing robust guidelines for ethical AI use, including monitoring and auditing processes, ensures transparency, fairness, and accountability,” Yogi stated. “This focus on responsible AI practices is essential for maintaining the integrity of our technologies”.
This consistent focus reinforces that, under leaders like Yogi, future innovation and ethical responsibility are intertwined. Continued cross-functional collaboration and a commitment to continuous iteration based on performance and user feedback round out the strategy, ensuring that innovations are effectively developed, deployed, and refined to meet user needs and business goals.
Lohitaksh Yogi’s work exemplifies the modern AI product leader navigating the complex intersection of deep technology, user-centric design, and strategic business imperatives. His journey from data science roots to leading significant AI initiatives underscores the value of combining profound technical expertise—spanning LLMs, generative AI, RLHF, RAG, and multimodal systems—with core product management disciplines like strategic planning, customer focus, and decisive leadership.
Through his contributions to platforms like the Virtual Agent enterprise system, Lohitaksh has demonstrated a structured approach that consistently balances technical feasibility with user needs and business impact, notably integrating ethical considerations as a fundamental pillar alongside integration, value delivery, and continuous improvement.
His leadership style fosters collaboration and leverages both his technical background and diverse team expertise, embedding ethical responsibility within the team culture.
By championing a holistic strategy that includes continuous learning, fostering internal innovation, adopting advanced technologies responsibly, and iterating based on real-world feedback, Lohitaksh plays a key role in ensuring companies not only keeps pace with the rapid evolution of AI but continues to shape the future of AI-driven customer experiences and enterprise solutions, maintaining the company’s position at the vanguard of the digital landscape.
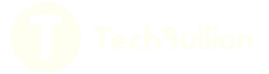