The integration of Artificial Intelligence (AI) and Big Data into Battery Electric Vehicles (BEVs) represents a cutting-edge fusion of technology and transportation that is driving significant advances in the automotive industry. As BEVs continue to gain popularity as a sustainable alternative to internal combustion engine vehicles, the role of AI and Big Data has become increasingly crucial. These technologies enhance vehicle performance, optimize battery management systems, improve energy efficiency, and contribute to environmental sustainability efforts. This intersection of AI, Big Data, and BEVs not only marks a transformative shift in how vehicles are powered and operated but also how they interact with the broader energy ecosystem, including integration with the power grid through Vehicle-to-Grid (V2G) and Vehicle-to-Home (V2H) technologies. The application of AI and Big Data in BEVs encompasses a range of functionalities from advanced diagnostics and predictive maintenance to improving charging efficiencies and extending battery life.
By analyzing vast amounts of data generated by the vehicle sensors and external sources, AI algorithms can predict battery performance, optimize charging schedules based on real-time grid capacity, and enhance the driving experience through adaptive learning of driver behaviors. Furthermore, these technologies play a pivotal role in the development of V2G services, allowing BEVs to contribute to grid stability and support the transition to renewable energy sources. However, the adoption of AI and Big Data in BEVs is not without its challenges. Issues such as data privacy and security, the environmental impact of data centers, and the need for substantial investments in infrastructure pose hurdles to widespread implementation. Moreover, regulatory and legal frameworks are still evolving to keep pace with these technological advances, particularly concerning V2G and smart grid integration. Despite these challenges, the future of BEVs is intrinsically linked to the advancements in AI and Big Data.
Ongoing research and development are likely to focus on optimizing battery technologies, improving the efficiency of electric drivetrains, and enhancing the sustainability of the BEV ecosystem. As the industry moves forward, the convergence of these technologies will continue to play a vital role in shaping the next generation of electric vehicles, driving towards a more sustainable, efficient, and interconnected automotive future.
Historical Context
The intersection of Artificial Intelligence (AI) and Big Data in the realm of Battery Electric Vehicles (BEVs) is a narrative of evolving technologies and their application in enhancing the automotive industry. The conceptual foundations of big data existed long before the term itself was popularized, signifying a period where immense volumes of data were being accumulated across various sectors[1]. Similarly, the emergence of AI predates its current widespread application, gradually developing into a tool indispensable for data analysis and interpretation. The historical trajectory of these technologies showcases a symbiotic relationship wherein the utility of AI is magnified by the availability of extensive datasets, and the potential of big data is unlocked through sophisticated AI algorithms[1]. The application of big data and AI in the context of BEVs is a relatively recent phenomenon, paralleling the broader adoption of these vehicles.
Battery electric vehicles, characterized by their reliance on electric motors and large traction battery packs, represent a significant shift from traditional internal combustion engine vehicles[2]. This transition not only signifies a move towards more sustainable modes of transportation but also introduces new dimensions for data generation and utilization[3]. Sensors integrated into BEVs generate voluminous data, offering insights into vehicle performance, battery health, and user behavior. These data points serve as a foundation for applying AI to enhance various aspects of BEVs, including battery life, charging efficiency, and vehicle safety[3][4]. The integration of AI and big data in BEVs underscores a broader trend towards data-driven decision-making in the automotive sector. This trend is facilitated by the increasing digitization of vehicles and the proliferation of connected devices, leading to an exponential growth in the volume of data generated[3].
The analysis of this data through AI algorithms enables predictive maintenance, optimized battery usage, and improved vehicle performance, illustrating the transformative potential of these technologies in the electric vehicle domain[4]. Moreover, the historical development of AI and big data adoption in BEVs is influenced by the need for better understanding and managing the charging infrastructure required for these vehicles. Traditional model-based analyses often relied on outdated assumptions about charging behavior, underscoring the necessity for more accurate and empirical data to guide infrastructure development[5][6]. The scarcity of public data on BEV mobility patterns further emphasizes the role of big data in bridging this knowledge gap, facilitating more effective planning and implementation of charging networks[6].
The Role of AI and Big Data in BEVs
The integration of Artificial Intelligence (AI) and Big Data in Battery Electric Vehicles (BEVs) is revolutionizing the automotive industry, enhancing vehicle performance, energy efficiency, and the overall driving experience. These technologies are pivotal in optimizing battery management, vehicle-to-grid (V2G) services, and environmental sustainability efforts.
Battery Management and Performance Enhancement
AI and Big Data play a crucial role in the development of advanced battery management systems (BMS) for BEVs. By leveraging machine learning algorithms, BEVs can better predict battery life, optimize charging cycles, and improve energy efficiency[1][7]. AI algorithms analyze vast amounts of data related to battery chemistry, charge/discharge rates, temperature, and vehicle usage patterns to predict and enhance battery performance over time[8]. This not only prolongs the battery’s lifespan but also ensures that the vehicle operates at peak efficiency.
Vehicle-to-Grid (V2G) Integration
V2G technology represents a significant advancement in how BEVs interact with the power grid. Through the use of AI and Big Data, BEVs can contribute to grid stability by returning energy during peak demand times[9]. AI systems can analyze grid data to determine the best times for charging and discharging, maximizing the financial benefits for vehicle owners and aiding in the efficient use of renewable energy sources[9]. As V2G technology matures, it is expected to play a vital role in the global transition to sustainable energy.
Environmental Sustainability
The adoption of AI and Big Data in battery electric vehicles (BEVs) brings to the fore significant considerations regarding environmental sustainability. As the automotive industry moves towards electrification, the integration of advanced technologies such as AI and big data analysis is seen as a crucial step towards enhancing the sustainability and efficiency of BEVs.
Core Technologies and Implementations
The development and optimization of battery electric vehicles (BEVs) heavily rely on the integration and advancement of various core technologies, particularly artificial intelligence (AI) and big data analytics. These technologies play a crucial role in addressing some of the most significant challenges in the BEV industry, including battery management, vehicle-to-grid (V2G) systems, and driving range prediction.
Battery Management Systems
One of the pivotal aspects of enhancing BEV performance is the optimization of battery management systems (BMS). The complexity of battery behavior, influenced by thermal management, charging cycles, and degradation over time, necessitates sophisticated modeling techniques. Researchers and engineers are leveraging machine learning algorithms to predict battery health, state of charge (SOC), and state of health (SOH) more accurately. Machine learning models can process extensive datasets obtained from experimental measurements to identify patterns indicative of battery aging and performance degradation[7]. This method enables non-invasive monitoring and extends the battery’s useful lifespan by distinguishing critical signals from irrelevant noise. Additionally, the exploration of advanced predictive analytics is advocated to forecast battery degradation, which could facilitate proactive maintenance and improve the reliability of BEVs[14].
Vehicle-to-Grid (V2G) and Vehicle-to-Home (V2H)
The integration of BEVs with the power grid and home energy systems through V2G and V2H technologies represents a significant leap towards smart energy management. These systems allow electric vehicles to not only draw energy for charging but also supply power back to the grid or home, offering a potential solution for energy storage and emergency power supply[15][8]. However, the commercial implementation of such technologies faces challenges, including the need for optimal charging infrastructure and intelligent control systems for charging processes. Big data and AI play vital roles in optimizing these systems by analyzing real-time data from the grid and managing the bidirectional flow of electricity efficiently[16].
Predictive Analytics for Driving Range
Predictive analytics is another area where AI and big data are making a substantial impact. By analyzing driving habits, vehicle conditions, and external factors, machine learning methods can predict the driving range of BEVs with higher accuracy. Incorporating the driving mode into predictive models shows that stable driving habits contribute significantly to conserving battery power and extending the driving range[17]. This not only enhances the user experience by providing more reliable range estimates but also assists in planning charging schedules and routes for better energy utilization.
Environmental Sustainability
The adoption of AI and Big Data in battery electric vehicles (BEVs) brings to the fore significant considerations regarding environmental sustainability. As the automotive industry moves towards electrification, the integration of advanced technologies such as AI and big data analysis is seen as a crucial step towards enhancing the sustainability and efficiency of BEVs. This section explores the environmental implications of such technological advancements, alongside measures and initiatives aimed at mitigating potential adverse effects.
Environmental Impact and Mitigation Efforts
The environmental footprint of processing big data and utilizing AI technologies cannot be overlooked. The Information and Communication Technology (ICT) sector, integral to the functioning of AI and big data, is responsible for approximately 3–4% of global emissions, with data centers consuming vast volumes of water for cooling purposes[10]. To counteract this, efforts like the CODES Action Plan for a Sustainable Planet in the Digital Age are underway. This initiative aims at reducing the environmental footprint of digital technologies, highlighting the industry’s move towards more sustainable practices[10].
AI in Enhancing Environmental Sustainability
AI and big data offer promising solutions to environmental challenges faced by the BEV sector. For instance, AI can significantly contribute to designing energy-efficient vehicles, monitoring deforestation, and optimizing the deployment of renewable energy sources. The versatility of AI applications ranges from global emissions monitoring through satellite technology to more localized solutions such as intelligent home systems that conserve energy by turning off lights and heat when not needed[10].
Sustainable Data Management
Given the environmental costs associated with data processing, the call for sustainable data management practices is growing louder. Enterprises are encouraged to consider the environmental impact of their AI and big data operations critically. This includes improving carbon accounting for more accurate data on carbon footprints and sustainability impacts, thereby enabling more informed decision-making aimed at reducing the environmental impacts of technological advancements in the BEV sector[11].
Legislation and Regulatory Measures
Legislation plays a pivotal role in accelerating the transition to sustainable mobility. Ambitious legislation, along with regulations on Extended Producer Responsibility (EPR) for EV batteries in regions like Australia, Canada, and California, aims at fostering circularity within the automotive sector. Such measures address the looming waste problem associated with end-of-life electric vehicles and the valuable materials contained within lithium-ion batteries, emphasizing the need to retain this value within local economies[12].
Traceability and Accountability
The traceability of key EV components is crucial for monitoring the progress of environmental and social development goals throughout the battery and EV supply chains. Governments and regulatory bodies are tasked with ensuring that the adoption of AI and big data in the BEV industry aligns with broader environmental sustainability objectives. This includes advancing research, development, and the commercialization of energy technologies while keeping a close eye on electric vehicle sales, stock, charging infrastructure, and the impact on oil displacement[13].
Challenges and Solutions
The development and integration of artificial intelligence (AI) and Big Data into the battery electric vehicles (BEVs) ecosystem present a myriad of challenges, as well as potential solutions that promise to revolutionize this sector. This section delves into the primary obstacles and the innovative strategies being employed to navigate these complexities.
Legal and Regulatory Hurdles
One of the significant challenges in the advancement of vehicle-to-grid (V2G) and vehicle-to-home (V2H) technologies is the existing legal and regulatory framework[15]. The deployment of these technologies requires a clear regulatory environment that currently hampers their commercialization. Legal questions surrounding the organization of private services on the power grid need thorough clarification to facilitate further development.
Environmental Impact of Data Processing
The processing of vast amounts of data necessary for enhancing environmental monitoring and sustainable practices in e-commerce platforms has an inherent environmental cost[10]. This issue underscores the need for balancing digital innovation with environmental sustainability, ensuring that the advancements in AI and Big Data do not exacerbate the environmental footprint of digital operations.
Technological Complexities
Implementing V2H charging solutions involves technologically sophisticated systems. These systems must integrate software capable of real-time communication with the central grid to monitor and respond to system demand[8]. The complexity of these technologies poses a significant challenge in making V2G and V2H solutions widely accessible and efficient.
Data Privacy and Ethics
The extraction of value from Big Data analytics in the BEV sector must be approached with a keen consideration for data privacy and ethics[18]. As data collection and analysis become more integrated into vehicle technologies, ensuring the privacy and ethical use of consumer data is paramount. This challenge calls for stringent data management and protection measures throughout the data lifecycle.
Grid Management and Demand
The integration of renewable energy sources and the growing demand from electric vehicles put a strain on the modern power grid[16][19]. AI and Big Data offer solutions for grid analytics and management by providing new insights and control systems to balance electric demand with generation efficiently. However, the complexity and scale of grid management pose a formidable challenge that requires innovative and scalable solutions.
Solutions
Advancements in AI for Grid Management
The application of AI to speed up essential calculations for regional electric system planning, such as the security constrained unit commitment (SCUC), demonstrates a potential solution to manage the complexities of grid management[20]. AI can leverage accumulated data to discover patterns that enhance the efficiency of power systems, addressing the challenge of integrating renewable energy sources and electric vehicle charging demands.
Developing Sustainable Data Processing Methods
Addressing the environmental impact of data processing involves the development of more sustainable methods for data analysis and management[10]. This solution aims to reduce the carbon footprint associated with Big Data and AI applications, aligning digital innovation with environmental sustainability goals.
Legal and Regulatory Framework Improvement
To overcome legal and regulatory hurdles, concerted efforts are required to establish a clear and supportive regulatory environment for V2G and V2H technologies[15]. This involves collaboration between stakeholders to clarify legal questions and create conducive conditions for the commercialization and broader adoption of these technologies.
Ethical Data Management Practices
Implementing stringent data privacy and ethics protocols at each phase of the data lifecycle is crucial[18]. By prioritizing data protection and ethical considerations, the BEV sector can navigate the challenges of data management, ensuring consumer trust and compliance with regulatory standards.
Future Directions
The future of battery electric vehicles (BEVs) is inextricably linked with the advancements in artificial intelligence (AI), big data, and the development of battery management systems (BMS). As the sector evolves, several directions are emerging, indicating where efforts and research are likely to concentrate in order to push the boundaries of technology and sustainability in electric mobility.
Optimization and Algorithms in BMS
The continuous development and enhancement of battery management systems are critical for the sustainable operation and management of BEVs. The integration of advanced algorithms and optimization techniques is expected to significantly improve the efficiency, safety, and lifespan of lithium-ion batteries, which are currently the most common type used in electric vehicles[14]. These technological advancements will not only enhance vehicle performance but also contribute to the overall sustainability of electric mobility by reducing waste and the need for frequent battery replacements.
V2G and V2H Technologies
Vehicle-to-Grid (V2G) and Vehicle-to-Home (V2H) technologies present exciting opportunities for the future of BEVs. By allowing electric vehicles to return energy to the power grid or supply emergency power to homes, these technologies can greatly enhance the stability and resilience of the energy supply. Despite the current legal and regulatory challenges hindering their widespread commercialization, ongoing pilot projects and research into intelligent charging controls are paving the way for their eventual integration into the electric vehicle ecosystem[15][8].
AI and IoT in Battery Performance
The potential of AI and the Internet of Things (IoT) to revolutionize battery performance in BEVs is immense. Through the development of new electrode materials and the optimization of battery design, these technologies can significantly improve the cycle life of batteries. Moreover, AI and IoT can enhance battery management systems by providing new ways to monitor and manage energy use, thereby improving both the efficiency and safety of electric vehicles[21][22].
Addressing Environmental and Social Impacts
As the adoption of BEVs continues to grow, it is crucial to monitor and ensure the traceability of key components and assess the environmental and social impacts of their supply chains. Governments and organizations are urged to advance research, development, and commercialization efforts while keeping ambitious environmental and social development goals in focus[13]. This holistic approach will help mitigate any negative impacts associated with the increased demand for electric vehicles and their components.
Big Data and AI in Environmental Sustainability
The relationship between big data, AI, and environmental sustainability is complex. On one hand, the exponential growth in data and its processing demand poses challenges in terms of energy consumption and greenhouse gas emissions[11]. On the other hand, AI and big data analytics offer powerful tools for managing and reducing these emissions, thereby supporting the global effort to combat climate change. The challenge lies in balancing the benefits of these technologies with their environmental impacts, a task that will require innovative solutions and sustainable practices[1][23].
Case Studies and Industry Examples
The integration of AI and Big Data in the realm of Battery Electric Vehicles (BEVs) showcases a transformative approach towards enhancing performance, reliability, and the overall driving experience. This section delves into various industry examples and case studies that highlight the pivotal role of these technologies in the evolution of electric vehicles.
Big Data Analytics in Insurance
In May 2019, the European Insurance and Occupational Pensions Authority (EIOPA) conducted a thematic review to assess the adoption of Big Data Analytics within the motor and health insurance sectors. This review revealed that approximately one-third of the participating insurance companies were already leveraging a broad array of AI use cases throughout various stages of the insurance value chain. Another third were in the experimental phase, testing the waters with this technology to optimize insurance models specifically for BEVs[23]. This initiative underscores the increasing reliance on Big Data to tailor insurance products that are more aligned with the unique attributes and risk profiles of electric vehicles.
Advancements in Battery Development
A study led by the Toyota Research Institute presented a novel approach to battery development, employing Big Data and AI to expedite the design and optimization processes for electric vehicle batteries. This method not only reduced the time required for testing battery charge speeds but also showed potential for application in numerous other aspects of battery development, including chemistry design, size, shape, and manufacturing[24]. By conducting over 20,000 experimental measurements to train machine learning models, researchers were able to identify specific features in the electrical response indicative of battery aging, thereby enhancing the safety, reliability, and overall performance of BEVs[7].
Vehicle-to-Home (V2H) Systems
The concept of Vehicle-to-Home systems represents a significant stride towards integrating electric vehicles within the broader energy ecosystem. Electric vehicles, with their substantial energy storage capacity, can supply emergency power to homes for several days. Implementing V2H systems, however, necessitates complex technological solutions to enable seamless communication between charging stations, electric vehicles, and the central power grid[8]. This integration is facilitated by sophisticated software and Big Data analytics that monitor real-time system demand and manage the distribution of energy between the vehicle and home.
Vehicle-to-Grid (V2G) Technology
Vehicle-to-Grid technology exemplifies the potential of BEVs to contribute actively to the energy grid. By allowing electric vehicles to synchronize with the electricity grid and potentially return power during peak demand periods, V2G technology represents a paradigm shift in how energy is stored and distributed. This system relies on advanced bidirectional chargers and AI algorithms that optimize the interaction between electric vehicles and the power grid, thereby stabilizing it and reducing congestion at charging stations[25][26]. The development of V2G technology is poised to make electric vehicles not just consumers of energy but also providers, further integrating them into the smart grid infrastructure.
References
[1] : Title of the article: Big Data and Artificial Intelligence: How They Work Together. (2017, July 21). Maryville Online. https://online.maryville.edu/blog/big-data-is-too-big-without-ai/
[2] : Title of the article: How Do All-Electric Cars Work?. Alternative Fuels Data Center. Retrieved from https://afdc.energy.gov/vehicles/how-do-all-electric-cars-work
[3] : Delnevo, G., Di Lena, P., Mirri, S. et al. On combining Big Data and machine learning to support eco-driving behaviors. J Big Data 6, 64 (2019). https://doi.org/10.1186/s40537-019-0226-z
[4] : Staff. (2021, September 1). How electric vehicles are leveraging artificial intelligence to improve battery performance and charging efficiency. Circuit Digest. https://circuitdigest.com/article/how-electric-vehicles-are-leveraging-artificial-intelligence-to-improve-battery-performance-and-charging-efficiency
[5] : Liao, Y., Tozluoğlu, Ç., Sprei, F., Yeh, S., & Dhamal, S. (2023). Impacts of charging behavior on BEV charging infrastructure needs and energy use. Transportation Research Part D: Transport and Environment, 116, 103645. https://doi.org/https://doi.org/10.1016/j.trd.2023.103645
[6] : Gaete-Morales, C., Kramer, H., Schill, WP. et al. An open tool for creating battery-electric vehicle time series from empirical data, emo boy. Sci Data 8, 152 (2021). https://doi.org/10.1038/s41597-021-00932-9
[7] : Title of the article: AI techniques used to improve battery health and safety. University of Cambridge, Energy Department. Retrieved from https://www.energy.cam.ac.uk/news-and-events/news/ai-techniques-improve-battery-health-safety
[8] : Title of the Wikipedia article: Vehicle-to-grid. (n.d.). In Wikipedia. Retrieved May 1, 2024, from https://en.wikipedia.org/wiki/Vehicle-to-grid
[10] : Title of the article: How artificial intelligence is helping tackle environmental challenges. (2022, November 7). United Nations Environment Programme. Retrieved from https://www.unep.org/news-and-stories/story/how-artificial-intelligence-helping-tackle-environmental-challenges
[11] : Lipu MSH, Miah MS, Jamal T, Rahman T, Ansari S, Rahman MS, Ashique RH, Shihabuddin ASM, Shakib MN. Artificial Intelligence Approaches for Advanced Battery Management System in Electric Vehicle Applications: A Statistical Analysis towards Future Research Opportunities. Vehicles. 2024; 6(1):22-70. https://doi.org/10.3390/vehicles6010002
[12] : Title of the webpage: Vehicle-to-Grid. (n.d.). The Mobility House. Retrieved from https://www.mobilityhouse.com/int_en/vehicle-to-grid
[13] : Sisinna, G. (2022). How artificial intelligence & big data are transforming the energy sector. LinkedIn. Retrieved May 1, 2024, from https://www.linkedin.com/pulse/how-artificial-intelligence-big-data-transforming-energy-sisinna
[14] : Shuai Sun, Jun Zhang, Jun Bi, Yongxing Wang, “A Machine Learning Method for Predicting Driving Range of Battery Electric Vehicles”, Journal of Advanced Transportation, vol. 2019, Article ID 4109148, 14 pages, 2019. https://doi.org/10.1155/2019/4109148
[15] : Marr, B. (2023, March 22). Green intelligence: Why data and AI must become more sustainable. Forbes. Retrieved May 1, 2023, from https://www.forbes.com/sites/bernardmarr/2023/03/22/green-intelligence-why-data-and-ai-must-become-more-sustainable/
[16] : Shell, C. (2021, January 25). Battery analytics and diagnostics: How big data makes EV batteries smarter, increases longevity, and unlocks second-life use. CleanTech. Retrieved from https://www.cleantech.com/battery-analytics-and-diagnostics-how-big-data-makes-ev-batteries-smarter-increases-longevity-and-unlocks-second-life-use/
[17] : International Energy Agency. (n.d.). Electric vehicles. Retrieved from https://www.iea.org/reports/electric-vehicles
[18] : Wing, J. M. (2019). The Data Life Cycle. Harvard Data Science Review, 1(1). https://doi.org/10.1162/99608f92.e26845b4
[19] : EV Connect. (Year, December 20). What is vehicle-to-grid for electric vehicles? EV Connect Blog. Retrieved from https://www.evconnect.com/blog/what-is-vehicle-to-grid-for-electric-vehicles
[20] : Nunez, C. (2019, June 14). Artificial intelligence can make the US electric grid smarter. Argonne National Laboratory. Retrieved from https://www.anl.gov/article/artificial-intelligence-can-make-the-us-electric-grid-smarter
[21] : Mahabadi, P. (2022, December 17). Artificial intelligence & its role in mass adoption of electric vehicles. e-vehicleinfo.com. Retrieved from https://e-vehicleinfo.com/artificial-intelligence-its-role-in-mass-adoption-of-electric-vehicles/
[22] : (2022, August 24). The role of IoT & AI in battery management of electric vehicles. Aeologic Technologies. Retrieved from https://www.aeologic.com/blog/the-role-of-iot-ai-in-battery-management-of-electric-vehicles/
[23] : European Insurance and Occupational Pensions Authority. (n.d.). Artificial intelligence and big data. Retrieved from https://www.eiopa.europa.eu/browse/digitalisation-and-financial-innovation/artificial-intelligence-and-big-data_en
[24] : Stanford University. (2020, February 22). Artificial intelligence used to supercharge battery development for electric vehicles. SciTechDaily. Retrieved from https://scitechdaily.com/artificial-intelligence-used-to-supercharge-battery-development-for-electric-vehicles/
[25] : Svarc, J. (2024, February 8). V2G explained: Vehicle-to-grid benefits. Clean Energy Reviews. Retrieved from https://www.cleanenergyreviews.info/blog/v2g-explained-vehicle-to-grid-benefits
[26] : Ahmed, M., Zheng, Y., Amine, A., Fathiannasab, H., & Chen, Z. (2021). The role of artificial intelligence in the mass adoption of electric vehicles. Joule, 5(9), 2296–2322. https://doi.org/https://doi.org/10.1016/j.joule.2021.07.012
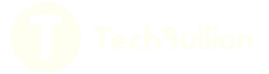