Introduction
In the ever-evolving landscape of technology, Artificial Intelligence (AI) stands out as one of the most transformative and promising innovations of our time. However, as AI systems become more pervasive in our daily lives, there is a growing need for transparency and understanding of how these systems arrive at their decisions. This is where Explainable Artificial Intelligence (XAI) comes into play.
Understanding XAI: Bridging the Gap
Explainable Artificial Intelligence, or XAI, is a concept aimed at making AI systems more transparent and understandable to humans. It seeks to bridge the gap between the complex inner workings of AI algorithms and the need for human comprehension and trust. By providing explanations for AI-driven decisions and predictions, XAI enables users to better understand and trust these systems.
Importance of Transparency
Transparency is crucial in ensuring the ethical and responsible deployment of AI technologies. Without insight into how AI systems reach their conclusions, it becomes challenging to identify biases, errors, or unintended consequences. XAI addresses this issue by opening the black box of AI and shedding light on the decision-making process.
Building Trust Through Interpretability
Trust is fundamental in the adoption of AI technologies across various domains, including healthcare, finance, and criminal justice. XAI enhances trust by offering interpretable explanations for AI outputs, allowing users to verify the validity and fairness of the decisions made. This transparency fosters confidence in AI systems and encourages their acceptance and adoption.
Techniques for Explainability
There are various techniques employed in XAI to enhance interpretability and transparency. These include:
Feature Importance:
Identifying the most influential features or factors contributing to AI predictions.
Model Visualization:
Visual representations of AI models and their decision boundaries to aid understanding.
Rule Extraction:
Deriving human-readable rules from complex AI models to explain their behavior.
Counterfactual Explanations:
Presenting alternative scenarios to illustrate how changes in input would affect the output.
Real-World Applications
XAI has applications across diverse industries, where trust and understanding are paramount. In healthcare, for example, XAI can provide explanations for medical diagnoses, helping clinicians interpret AI-driven recommendations and improving patient outcomes. Similarly, in finance, XAI can enhance transparency in credit scoring and investment decisions, reducing the risk of bias and discrimination.
Challenges and Considerations
Despite its benefits, implementing XAI poses several challenges. Ensuring both accuracy and interpretability can be complex, as simpler models may sacrifice predictive performance for transparency, while more complex models may be harder to interpret. Additionally, maintaining privacy and security while providing explanations for AI decisions requires careful consideration and robust safeguards.
The Future of XAI
As AI continues to advance and integrate into society, the importance of XAI will only grow. Innovations in explainable techniques and frameworks will enable AI systems to become more transparent, trustworthy, and accountable. By prioritizing transparency and interpretability, we can harness the full potential of AI while mitigating risks and ensuring ethical use.
Conclusion
Explainable Artificial Intelligence (XAI) holds the key to unlocking the full potential of AI while maintaining transparency, trust, and accountability. By providing interpretable explanations for AI-driven decisions, XAI empowers users to understand, verify, and ultimately trust these systems. As we navigate the complexities of AI adoption, embracing XAI will be essential in ensuring ethical and responsible AI deployment for the benefit of society as a whole.
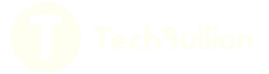