The Evolution of Temporal Causal Analysis, Sree Charanreddy Pothireddi explores a crucial aspect of artificial intelligence and data science in his latest research, focusing on delayed causal effects in complex systems. His work sheds light on how emerging analytical techniques enhance our ability to predict and manage outcomes across diverse industries.
The Challenge of Time in Causal Models
Interventions in complex systems rarely produce immediate effects, but instead create impacts that manifest over time through delayed responses and feedback loops. These temporal dependencies can vary significantly, with some effects appearing minutes later while others emerge after weeks or months. Traditional models that assume contemporaneous relationships often fail to capture these dynamics, leading to inaccurate predictions and suboptimal decisions. By integrating time-delay modeling techniques, we can better represent how interventions ripple through systems, accounting for latency periods, cumulative effects, and temporal cascades. This approach enables more realistic forecasting .
Deep Learning’s Role in Temporal Analysis
Advancements in deep learning have played a pivotal role in improving causal analysis. Neural networks equipped with multi-head attention mechanisms have significantly enhanced prediction accuracy, reducing errors by over 37%. These models enable the identification of complex interactions across multiple variables, which is particularly beneficial in industrial applications where process optimization and predictive maintenance are critical.
Beyond mere correlation detection, these architectures can now discern temporal dependencies with unprecedented granularity, revealing causation patterns previously hidden in noisy data. By incorporating transfer learning techniques, modern systems can leverage domain knowledge across similar processes, accelerating model convergence even with limited training data. This capability has revolutionized fault diagnosis in manufacturing environments, where early detection of emerging issues can prevent costly downtime and extend equipment lifespan while maintaining production quality.
Energy Sector Innovations
The energy sector showcases the importance of delayed causal analysis, especially with the increasing integration of renewable energy sources. Studies indicate that policy interventions in energy grids require months or even years to manifest measurable impacts. Advanced machine learning models help predict grid stability responses, optimizing the balance between demand and supply.
Manufacturing and Process Optimization
Manufacturing industries have leveraged process mining techniques to analyze production delays. Research reveals that inefficient resource allocation accounts for a substantial portion of temporal delays in production cycles. By applying predictive analytics, manufacturers have successfully reduced waiting times and improved operational efficiency by over 30%.
Enhancing Supply Chain Dynamics
Supply chain management has long struggled with delays stemming from logistical coordination, quality verification, and documentation processing. Through temporal analysis, organizations have identified systematic delays and implemented targeted strategies to reduce lead times. These interventions have improved overall efficiency by nearly 29%, showcasing the power of data-driven decision-making.
Advanced Analytical Techniques in Causal AI
The research also highlights several advanced methods improving temporal causal analysis. Granger causality analysis, for example, has achieved remarkable success in fault diagnosis, identifying the root causes of process disturbances with 92.4% accuracy. Similarly, Dynamic Time Warping (DTW) techniques have enhanced real-time monitoring in smart manufacturing, reducing inspection times while maintaining high detection accuracy.
Simulation-Based Validation for Greater Precision
The rise of digital twin technology has revolutionized the validation of temporal models. By simulating real-world conditions, researchers have refined predictive models, improving equipment efficiency and reducing process setup times by over 40%. This approach ensures that AI-driven insights are both practical and actionable.
The Future of Temporal Analysis
Automated delay discovery is rapidly advancing, allowing AI systems to identify temporal sequences with unprecedented precision. New pattern recognition techniques reduce manual effort and enhance accuracy, making it easier to uncover hidden causal relationships. As AI-driven analytics continue to evolve, these advancements will play a crucial role in sectors ranging from healthcare to finance, enabling smarter, data-informed decisions.
In conclusion, Sree Charanreddy Pothireddi’s work underscores the growing importance of understanding delayed causal effects. By leveraging neural networks, advanced pattern recognition, and simulation techniques, the field of temporal causal analysis is poised to transform how industries handle complex, time-dependent processes. As researchers refine these methodologies, the potential applications for AI-driven temporal analysis will continue to expand, paving the way for smarter, more predictive systems across various domains.
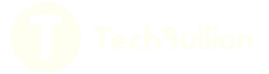