The advent of AI has drastically transformed various aspects of our lives and industries ranging from healthcare and education to finance and transportation. However, as we increasingly entrust decision-making processes to AI, we must confront a critical issue: bias in AI.
- Definition of AI and its Importance
Artificial Intelligence (AI) refers to the simulation of human intelligence in machines that are programmed to learn and mimic human actions. As per a Statista report, the global AI market is expected to reach $126 billion by 2025, underscoring the growing significance of AI in our world. AI’s capacity to analyze vast amounts of data and generate insights is making it indispensable across various sectors.
- Brief Overview of Bias in AI
Bias in AI involves the systematic error introduced into AI output due to unfair, partial, or prejudiced assumptions during the AI development process. This can lead to imbalances in representation or decision-making that can unfairly disadvantage certain groups. For instance, a hiring algorithm might favor candidates from certain universities, thereby potentially discriminating against equally or more qualified candidates from other institutions.
- Purpose of the Article
The purpose of this article is to explore the issue of bias in AI – its sources, the challenges it presents, and the solutions that can be implemented to create fairer algorithms. It is crucial to address these biases to ensure that as AI continues to evolve, it is harnessed for the benefit of all, not just a select few.
Before diving into the complexities of AI bias, it is essential to understand the broader context. Consider the example of hiring remote developers. With the expansion of remote work culture, hiring developers is no longer restricted to a particular location. This hiring process has increasingly become reliant on AI-powered tools. If unchecked, these AI systems might perpetuate bias, favoring candidates from certain backgrounds, thereby overlooking a vast pool of deserving candidates. The significance of addressing bias in such a context becomes all the more crucial to ensure a fair hiring process. This is where platforms such as RemoteBase, which allow you to hire remote developers from across the globe, play a pivotal role in diversifying the talent pool and mitigating bias.
In this article, we will navigate through the various aspects of AI bias and examine potential strategies to minimize it, aiming for a world where AI works equitably for everyone.
- Understanding Bias in AI
As AI’s influence continues to grow, its implications for society are becoming increasingly profound. A crucial aspect to consider is the potential for bias in AI, which can significantly impact various sectors and individuals.
- Explanation of What Bias in AI Means
AI bias refers to systematic errors that can occur in the outputs of AI algorithms due to skewed data inputs or flawed design. These biases can perpetuate and even exacerbate existing social inequalities and prejudices, leading to unfair outcomes. For instance, a biased AI model used in loan approval could potentially reject deserving applicants based on their demographic characteristics, instead of solely assessing their creditworthiness.
- Examples of Bias in AI
There are numerous instances where AI bias has resulted in discriminatory practices. One example is the realm of hiring. When recruiting platforms use AI to sort through applicant resumes, existing biases in the training data can result in unjust outcomes. A 2018 Reuters report highlighted how a leading tech company’s hiring algorithm developed a bias against female candidates because it was trained on historical data that favored males.
Similar cases of bias have also been observed in other AI applications. For instance, facial recognition technology has been shown to misidentify people of certain racial or ethnic groups more often than others, raising significant privacy and civil liberties concerns.
Moreover, even AI applications like sentiment analysis tools can display gender bias. According to a study published in the Proceedings of the National Academy of Sciences, some automated systems rated sentences more positively if they seemed to be written by men, reflecting the gender biases encoded in their training data.
- Impact and Repercussions of AI Bias on Society
The implications of AI bias can be significant and wide-ranging. It can result in unfair treatment of individuals or groups, exacerbate social inequities, and cause reputational and legal issues for organizations. For instance, companies using AI tools for hiring might overlook diverse talent due to algorithmic biases, causing not only unfair treatment of prospective candidates but also hampering the organization’s growth by limiting the diversity of ideas and experiences within the team. This makes it more crucial to adopt unbiased platforms such as RemoteBase to hire remote developers, ensuring a fair and diverse hiring process.
- Theories behind the Emergence of AI Bias
AI bias often originates from the data used to train the AI models. If the training data is biased, the AI model will likely reproduce these biases in its output. This is known as ‘algorithmic bias’. Moreover, bias can also occur due to the subjective decisions made by humans during the design and deployment of AI systems. For instance, if an AI system is primarily designed and developed by a homogeneous group, it might unintentionally incorporate their inherent biases, resulting in a biased AI system.
As we delve further into this article, we’ll look into various sources of bias in AI, challenges in addressing them, and potential solutions to create fairer algorithms.
III. Sources of Bias in AI Systems
In order to effectively address bias in AI, it’s essential to understand where and how these biases originate. Primarily, AI bias can be traced back to the training data used, the design of the algorithms, and the interpretation of AI outputs.
- Training Data Bias
Training data forms the foundation of any AI model. If the data used to train an AI system is not representative of the population it’s intended to serve, the system may reproduce and amplify these biases. For instance, if an AI is trained on data predominantly representing one racial or ethnic group, it may perform poorly when tasked with recognizing or understanding individuals from other racial or ethnic backgrounds.
- Bias in Algorithm Design
Bias can also be introduced through the design of the AI algorithm itself. Often, this is unintentional and can arise due to the oversight of the creators. The choice of algorithm, the features considered, and the way these features are weighted can all influence the outputs of the system. For example, if a hiring algorithm places too much weight on a particular characteristic such as attending a particular type of university, it could inadvertently disadvantage potentially qualified candidates from other types of educational institutions.
- Contextual and Cultural Bias
AI systems can also reflect cultural and societal biases. For example, language processing AI models may inherit the biases present in the text they’re trained on, leading to unfair or discriminatory language outputs. Moreover, if an AI system is deployed in a different context or culture than it was trained on, it might produce inappropriate or biased results due to the lack of context-specific data.
- Bias in Interpretation of AI Results
Lastly, bias can arise from the interpretation of AI results. For instance, an AI system may correctly identify trends in data, but the interpretation of these trends by human users can introduce bias. This might lead to misinformed decisions and unjust outcomes.
Addressing these sources of bias requires both technical and organizational changes. For instance, to counteract training data bias in the hiring process, companies can use platforms like RemoteBase to hire remote developers from a diverse global pool, thereby ensuring a more representative workforce. As we move forward, we’ll discuss the challenges in tackling AI bias and the strategies that can be employed to mitigate it.
- Challenges in Addressing Bias in AI
Addressing bias in AI is a complex issue due to multiple intertwined challenges. These range from technical difficulties in identifying and quantifying bias, to broader issues such as lack of diversity in AI development and legal and ethical considerations.
- Identifying and Quantifying Bias
One of the key challenges in dealing with AI bias is the identification and quantification of bias itself. AI systems, particularly those based on machine learning, often operate as a ‘black box’, with internal workings that are difficult to understand and interpret. It can be challenging to isolate the specific factors contributing to biased outputs, let alone quantifying the degree of bias.
- Lack of Diverse Representation in AI Development
The AI industry’s lack of diversity also presents a significant challenge. If the teams developing AI systems aren’t diverse, there’s a risk that the systems they create might unconsciously reflect their biases. According to a 2020 report by AI Now Institute, around 80% of AI professors are male, and racial diversity is also severely lacking in the AI research community. This lack of diversity contributes to the perpetuation of biases in AI systems.
- Complexity of AI Systems and Transparency Issues
The complexity of AI systems and algorithms adds to the difficulty of addressing bias. Many AI models, particularly deep learning models, are opaque, with complex inner workings that are challenging to interpret. This lack of transparency makes it hard to identify where bias might be creeping into the system.
- Legal and Ethical Challenges
Legal and ethical considerations further complicate the matter. It can be tricky to determine who is responsible for AI bias and its consequences – is it the creators, the users, or the decision-makers? From an ethical perspective, what constitutes ‘fairness’ in AI is not always clear and can vary greatly depending on the context.
Despite these challenges, various strategies and efforts are being developed to mitigate bias in AI. Among these, diversifying AI development teams is a key step. Platforms like RemoteBase enable companies to hire remote developers from around the world, offering the potential to build more diverse and inclusive teams. As we move to the next section, we’ll explore these and other measures in more depth.
- Current Efforts and Approaches to Mitigate Bias in AI
Recognizing the potential harm of biased AI, researchers, practitioners, and organizations are working towards developing and implementing strategies to reduce and eliminate bias from AI systems. These approaches span from technical solutions, like fairness in AI models, to organizational measures such as increasing diversity in AI teams.
- Incorporation of Fairness in AI Models
One technical approach involves incorporating fairness directly into AI models. Researchers are developing algorithms designed to reduce bias and ensure fairness. Techniques such as ‘fairness through unawareness’, ‘demographic parity’, and ‘equalized odds’ are being explored to promote fairness in AI outputs.
- Use of Bias-Mitigating Algorithms and Techniques
Another strategy involves the use of bias-mitigating algorithms and techniques, such as differential privacy and federated learning. Differential privacy adds ‘noise’ to the data to protect individuals’ identities while still allowing useful data analysis, thereby mitigating the potential for discriminatory bias. Federated learning, on the other hand, allows AI models to learn from decentralized data, reducing the likelihood of bias stemming from a non-representative centralized dataset.
- Efforts Towards Transparency and Interpretability in AI Systems
Transparency and interpretability in AI systems is another focus area. Explainable AI (XAI) models are being developed that make it possible to understand and interpret the decision-making processes of AI systems. These models can help identify and rectify biases embedded in AI systems.
- Initiatives to Increase Diversity in AI Development
Diversifying AI development teams is a critical non-technical strategy being adopted to counteract AI bias. By including diverse perspectives in the development process, it’s possible to reduce unconscious biases and develop more balanced AI systems. Platforms like RemoteBase make it easier for organizations to hire remote developers from different backgrounds, bringing varied perspectives to AI development.
Addressing bias in AI is a complex task requiring concerted efforts from multiple stakeholders. In the next section, we will look at real-world case studies that provide valuable insights into the successes and failures of bias mitigation in AI.
- Case Studies of Bias Mitigation in AI
Several organizations and researchers have made strides in tackling bias in AI, providing insightful case studies. These real-world examples illustrate both the successes and challenges in mitigating bias, offering lessons for others aiming to create fairer AI systems.
- Case Study 1: Gender Bias in Language Models
A notable case is the attempt to mitigate gender bias in AI language models. In a study by the University of Washington and the Allen Institute for AI, researchers developed a method to adjust an AI model’s training process to reduce gender bias in its output. The technique was tested on a popular language model, resulting in significantly less biased outputs. This case demonstrates how modifying the training process of AI models can help reduce bias.
- Case Study 2: Racial Bias in Facial Recognition Technology
Facial recognition technology has often been criticized for its biased performance, especially towards people of color. In response, IBM developed a new dataset, designed to improve the accuracy of its facial recognition system across all skin tones. The company reported improved performance, reducing bias in the system. However, this case also highlights the continuous need for vigilance and testing, as later evaluations by external researchers indicated that the system still showed significant racial biases.
- Case Study 3: Improving Diversity in AI Development
Lastly, Google’s AI ethics initiative is a prominent example of efforts to increase diversity in AI development. Google has committed to increasing diversity within its AI teams and launched the AI Ethics Research Grants program to support external research in areas like fairness in AI. However, the road hasn’t been entirely smooth, with high-profile disputes highlighting the ongoing challenges in achieving diverse and equitable AI development.
These case studies underline the potential for bias mitigation in AI and also expose the difficulties involved. Building fairer AI systems is an ongoing journey that requires consistent efforts from the AI community. One approach to facilitating this process is through diversifying development teams. Platforms such as RemoteBase provide an effective way to hire remote developers from diverse backgrounds, bringing different perspectives to the table. In the concluding section, we will summarize key points and explore the future direction of fairness in AI.
VII. Recommendations for Fairer Algorithms
To reduce and ultimately eliminate bias in AI, a concerted and multi-faceted approach is necessary. Here, we provide several recommendations for organizations and AI practitioners striving to create fairer algorithms.
- Invest in Diverse Teams
A diverse team is crucial to spotting and mitigating biases. Diversity here refers to not only gender, race, or ethnicity, but also socioeconomic status, educational background, geography, and more. When diverse perspectives come together, the chances of recognizing and challenging inherent biases increase. Companies can leverage platforms such as RemoteBase to hire remote developers and build a diverse workforce that reflects a wider range of experiences and viewpoints.
- Improve Transparency and Interpretability
Transparency and interpretability in AI models is a crucial factor in identifying and addressing bias. By adopting explainable AI (XAI) methods, we can understand how a model makes decisions, thereby making it easier to identify potential sources of bias.
- Use Bias-Mitigating Techniques and Tools
AI practitioners should consider employing various bias-mitigating techniques and tools, from fairness-aware algorithms to differential privacy and federated learning techniques. It’s also essential to be aware of the limitations of these techniques, as each has its trade-offs.
- Incorporate Ethical Considerations in AI Development
Ethical considerations should be a key part of AI development. This involves considering the potential impact of AI systems on society and individuals, ensuring that AI systems respect human rights and avoid harm.
- Regular Testing and Auditing of AI Systems
Regular testing and auditing of AI systems can help identify biases and assess the effectiveness of bias mitigation strategies. Third-party audits can also provide an independent assessment of an AI system’s fairness.
These recommendations provide a roadmap towards fairer AI systems. However, achieving this goal will require continuous efforts, as the nature of bias and technology are both ever-evolving. Ensuring fairness in AI is an ongoing journey, and one that will be critical to the ethical and responsible use of AI.
VIII. Conclusion
Bias in AI is a profound issue with far-reaching implications. As AI systems continue to permeate every aspect of our lives, ensuring these systems are fair and unbiased is not just a technical necessity but also a moral imperative. Achieving this goal is challenging due to the complex nature of bias, the ‘black-box’ nature of many AI systems, and the lack of diversity in AI development.
We’ve explored a multitude of strategies to address these challenges, including the integration of fairness into AI models, the use of bias-mitigating algorithms, and efforts to improve transparency and interpretability in AI systems. However, technical solutions alone are not enough. Efforts to increase diversity in AI development, ethical considerations, and regular audits of AI systems are crucial elements in this endeavor.
It’s clear that addressing bias in AI is not a one-time task but an ongoing process that requires vigilance and commitment. This journey is vital to ensuring that AI systems are fair, equitable, and beneficial for all.
One practical step towards achieving this is by diversifying AI teams, bringing in varied perspectives to challenge and mitigate biases. Platforms such as RemoteBase offer an avenue to hire remote developers from different backgrounds, enhancing the potential to create unbiased AI systems.
As we look forward, it’s imperative for AI practitioners, organizations, and society as a whole to engage in this journey towards fairer AI systems. The path might be challenging, but the destination – a world where AI systems are equitable and just – is well worth the effort.
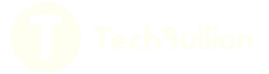