In the realm of healthcare data management and research, the OMOP (Observational Medical Outcomes Partnership) data model stands out as a pivotal framework for organizing and analyzing vast amounts of health data. This article provides a detailed overview of the OMOP data model, its origins, key components, benefits, and its impact on healthcare research.
Origins and Development
The OMOP data model was developed under the Observational Medical Outcomes Partnership, a public-private partnership initiated in 2008. The goal of OMOP was to improve the understanding of healthcare outcomes through the analysis of observational data. As the project evolved, it became evident that a standardized approach to managing and analyzing health data was essential. This led to the development of the OMOP Common Data Model (CDM), designed to facilitate the efficient use of data from disparate sources.
Key Components of the OMOP Data Model
The OMOP CDM is a comprehensive schema that standardizes the representation of health data across different databases. It comprises several core tables and concepts that provide a structured approach to data organization:
- Person Table: This table contains information about individuals such as demographics, vital status, and unique identifiers. It provides the foundational data required to track patient histories and outcomes.
- Observation Period Table: It captures the time frame during which data is available for each person, helping to define the scope of observational data and ensuring accurate temporal analysis.
- Condition Table: This table holds information about medical conditions diagnosed in patients. It includes details such as condition concepts, onset dates, and severity, allowing for the analysis of disease patterns and treatment outcomes.
- Drug Exposure Table: Here, data related to medication usage is stored. It includes information about drug names, dosages, and durations of use, facilitating studies on drug efficacy and safety.
- Procedure Table: This table records details about medical procedures performed on patients. It provides insights into the types of interventions patients receive and their outcomes.
- Measurement Table: It captures data from clinical measurements such as lab results and vital signs. This table is crucial for analyzing health trends and monitoring patient progress over time.
- Visit Occurrence Table: This table logs patient visits to healthcare providers, including details about visit types, locations, and dates. It helps track healthcare utilization and patient interactions with the healthcare system.
- Death Table: For patients who have deceased, this table records the date and cause of death, contributing to mortality analysis and epidemiological studies.
- Fact Relationship Table: This table facilitates the linking of different facts about patients, such as linking drug exposures to subsequent conditions, enhancing the ability to study causal relationships.
Benefits of the OMOP Data Model
The OMOP CDM offers several benefits that enhance the quality and efficiency of health data analysis:
- Standardization: By providing a standardized format for health data, the OMOP CDM ensures consistency across different datasets. This standardization simplifies data integration and comparison, making it easier to conduct large-scale studies and meta-analyses.
- Interoperability: The OMOP model supports the integration of data from various sources, including electronic health records (EHRs), claims data, and clinical trials. This interoperability is crucial for creating comprehensive datasets that reflect a wide range of patient experiences.
- Enhanced Research Capabilities: Researchers can use the OMOP CDM to perform complex analyses, such as cohort studies and comparative effectiveness research. The structured data format allows for sophisticated querying and analysis, leading to more robust findings.
- Facilitates Collaboration: The OMOP model promotes collaboration among researchers, institutions, and organizations. By working within a standardized framework, teams can share data and insights more effectively, advancing collective knowledge in the field.
- Data Quality and Integrity: The OMOP CDM includes mechanisms for data validation and quality assurance. This ensures that the data used in research is accurate and reliable, leading to more credible results.
Impact on Healthcare Research
The OMOP data model has significantly impacted healthcare research by providing a powerful tool for data analysis and integration. Its standardized approach has enabled researchers to address complex questions about health outcomes, treatment effectiveness, and disease patterns.
One notable application of the OMOP model is in the field of pharmacovigilance. By analyzing data on drug exposures and adverse events, researchers can identify potential safety issues and inform regulatory decisions. The model’s ability to integrate data from diverse sources enhances the ability to detect and assess drug-related risks.
In addition, the OMOP CDM supports epidemiological studies that explore the prevalence and incidence of diseases. Researchers can analyze large datasets to identify trends, evaluate risk factors, and assess the effectiveness of public health interventions.
The model also plays a crucial role in precision medicine, where individualized treatment strategies are developed based on patient-specific data. By providing a comprehensive view of patient health and treatment history, the OMOP CDM enables researchers to identify patterns that can guide personalized treatment approaches.
Future Directions
As the field of health data management continues to evolve, the OMOP data model is expected to adapt and expand. Future developments may include enhancements to accommodate new types of data, such as genomic and wearable device data. Additionally, ongoing efforts to improve data privacy and security will be crucial in maintaining the integrity of health data while ensuring compliance with regulatory requirements.
Conclusion
The OMOP data model represents a significant advancement in the management and analysis of healthcare data. Its standardized approach, combined with its ability to integrate diverse datasets, has transformed the landscape of health research. By providing a robust framework for data organization and analysis, the OMOP CDM continues to facilitate groundbreaking research and improve our understanding of health outcomes. As the healthcare field advances, the OMOP model will remain a cornerstone of data-driven research and innovation.
Read More From Techbullion And Businesnewswire.com
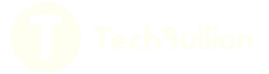