A leader in federated learning and cross-cloud system design, Krupal Gangapatnam has introduced a groundbreaking framework that redefines enterprise system migrations. This innovative approach combines federated learning with advanced security protocols to ensure secure, efficient, and privacy-preserving solutions for migrating critical systems across cloud platforms..
Tackling Migration Challenges
Migrating business-critical systems to the cloud faces challenges like data privacy, downtime, and compliance. This framework leverages federated learning to ensure efficiency, robust protection, and seamless solutions for modern enterprises.
A Privacy-Centric Approach
This framework’s privacy-preserving architecture employs hybrid encryption for secure data transfer, combining symmetric speed with asymmetric security. Techniques like k-anonymity and differential privacy ensure sensitive data remains anonymized yet usable.
The framework employs role-based access control with attribute-based encryption for granular permissions, ensuring authorized access. Aligned with GDPR and ISO 27001, it suits organizations in highly regulated industries.
Superior Performance and Security
Traditional migration methods can cause extended downtimes, taking up to 48 hours for 2TB of data. This solution reduces downtime to just 12 hours, achieving 99.95% availability with real-time synchronization and robust encryption protocols.
The framework’s security features include TLS 1.3, immutable storage, and centralized key management, ensuring protection against modern cyber threats. It demonstrated resilience to DDoS attacks up to 1Tbps, maintaining robust operational stability during testing.
Federated Learning: Redefining Migrations
Federated learning lies at the heart of this migration framework. Unlike traditional methods that centralize data for processing, federated learning enables distributed learning across multiple cloud environments. Local models are trained on-site and aggregated into a global model, significantly reducing privacy risks and network overhead.
This decentralized design not only ensures data sovereignty but also enhances regulatory compliance, making it a game-changer for organizations that prioritize privacy and security. Federated learning further reduces the system’s reliance on centralized data repositories, a critical aspect for businesses managing large-scale migrations.
Addressing Scalability Needs
The framework’s scalability has been tested extensively, demonstrating consistent performance for datasets up to 5TB. This scalability makes it a reliable choice for medium and large enterprises. However, coordination overhead becomes evident with datasets exceeding this threshold, highlighting areas for future innovation. The development of intelligent algorithms for conflict resolution and delta synchronization is underway to address these challenges.
Simplifying Complex Integrations
Integrating legacy systems into modern cloud platforms often presents hurdles for enterprises. This framework employs containerized agents to simplify deployment across heterogeneous environments. Automated resource allocation, intelligent load balancing, and event-driven synchronization ensure seamless operations during migrations. These features make the framework adaptable to the unique requirements of diverse enterprises while optimizing overall migration efficiency.
A Secure Future for Cloud Migrations
The success of this framework highlights exciting opportunities for the future. Planned advancements include AI-driven optimization of federated learning parameters to minimize expertise requirements and streamline adoption. Enhanced privacy-preserving techniques for handling increasingly diverse datasets are also on the horizon, which will expand the framework’s applicability to a broader range of industries.
The framework’s ability to automate resource optimization and prioritize data protection sets a benchmark for secure migration tools. It not only ensures a smooth transition to the cloud but also reduces the costs and risks associated with legacy systems. For organizations prioritizing data security and operational continuity, this methodology offers a compelling alternative to traditional approaches.
Driving Digital Transformation
The integration of cutting-edge federated learning techniques and enterprise-grade security measures demonstrates how this framework contributes to broader digital transformation goals. By reducing downtime, enhancing system availability, and safeguarding sensitive data, it addresses the core challenges of enterprise migrations. Moreover, its alignment with privacy and compliance regulations ensures that businesses can modernize their IT landscapes without compromising security.
The evolution of cloud technologies and stringent data protection requirements will continue to shape the demand for such privacy-preserving solutions. This framework provides a solid foundation for future innovations, paving the way for a more secure and efficient cloud migration landscape.
Krupal Gangapatnam’s contribution to this field underscores the transformative potential of federated learning. By addressing critical challenges in privacy and scalability, this framework offers a roadmap for secure and efficient enterprise migrations, setting new standards for innovation in cloud technologies.
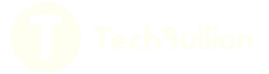