In today’s digital transformation era, the growing demand for scalable, intelligent, and cost-efficient data storage has accelerated the shift toward artificial intelligence-enhanced architectures. Gopinath Govindarajan, a thought leader in next-generation computing systems, explores this evolving frontier with deep insight and forward-thinking analysis. He presents a strategic vision that redefines traditional storage systems, aligning technological advancements with modern enterprise demands.
Building Bridges with Multi-Cloud Integration
As enterprises expand globally, data dispersion across cloud platforms is inevitable. Modern architectures now prioritize multi-cloud integration, using abstraction layers and metadata management to unify provider access. These systems simplify data movement, synchronize assets in real-time, and adapt dynamically to workload demands. Cost efficiency is another focal point—machine learning algorithms optimize storage allocation by analyzing usage patterns and predicting each dataset’s most economical and high-performing placement.
Reinventing Security Through Blockchain Storage
AI’s role in storage security is transformative, but when paired with blockchain technologies, it introduces a new dimension of data integrity. Blockchain-enabled storage systems offer tamper-proof audit trails and distribute verification across nodes, removing single points of failure. This is especially vital for industries bound by strict regulatory frameworks. Unlike traditional models that rely solely on access controls and encryption, these decentralized architectures provide immutable assurance through cryptographic validation.
Intelligent Tiering: Automating the Storage Hierarchy
Intelligent tiering systems powered by machine learning represent a transformative leap in data management. Rather than relying on static, manual policies, these systems use algorithms trained on historical access patterns to anticipate future data usage. Based on these predictions, data is automatically assigned to optimal storage tiers—ranging from high-performance to cost-efficient archival layers. Reinforcement learning techniques refine these decisions over time, enabling dynamic, real-time file migration. This automation enhances operational efficiency by balancing performance with cost, reducing human error, and freeing IT resources. Additionally, by ensuring that frequently accessed data remains on faster media. At the same time, infrequently used files are archived, these systems help lower storage costs, improve responsiveness, and extend the lifespan of expensive hardware infrastructure.
Taking Storage to the Edge for Real-Time Insights
Edge computing has become increasingly vital as organizations aim to process data nearer to its point of origin, minimizing reliance on centralized cloud infrastructure. This proximity significantly reduces latency, which is crucial for real-time applications in IoT, autonomous systems, and mobile platforms. Edge storage solutions incorporate intelligent pre-fetching, local caching, and selective synchronization to ensure efficient data access and transfer. These mechanisms allow edge devices to operate seamlessly even with intermittent connectivity or limited power resources. The ability to maintain performance under such constraints highlights the resilience and versatility of edge architectures, making them indispensable in today’s distributed and hyper-connected digital environments.
Self-Optimizing Systems: The Autonomous Frontier
AI has pushed storage management beyond automation into self-governance. Self-optimizing systems constantly analyze telemetry data, adjusting configuration parameters like cache sizes and data layout strategies to enhance performance. Reinforcement learning models further refine these adjustments by rewarding configurations that yield better outcomes. These architectures not only maintain operational efficiency under fluctuating workloads but also reduce the need for manual oversight, empowering storage to evolve as an intelligent, autonomous system.
Glimpsing Tomorrow: Quantum and Serverless Horizons
Quantum-inspired algorithms are revolutionizing storage optimization by enhancing data placement and pattern recognition, significantly improving search in large datasets—even before quantum hardware matures. Concurrently, serverless storage is transforming resource provisioning by removing infrastructure management complexities. This paradigm enables automatic scaling based on real-time demand, ensuring cost-efficiency and high performance without predefined allocation. Together, these innovations pave the way for smarter, more adaptive storage solutions that meet the growing demands of modern data-centric applications.
In conclusion, Gopinath Govindarajan encapsulates a pivotal moment in enterprise storage evolution—where data systems no longer just store but think, adapt, and optimize. The integration of AI across multiple storage layers not only transforms operational models but redefines the role of data infrastructure itself. As organizations confront rising volumes, varied access patterns, and heightened security expectations, the future of storage lies in its ability to learn, act, and respond—intelligently and autonomously. The journey from static systems to self-optimizing frameworks is not just technological—it’s strategic, shaping the digital enterprise of tomorrow.
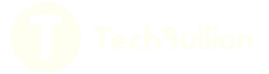