Unlocking Potential: The Leading AI Solutions for Data Science and Machine Learning in 2024
The rapid growth of artificial intelligence (AI) and machine learning (ML) has transformed how organizations handle data-driven projects. Choosing the right platform is crucial for effectively building, deploying, and scaling machine learning models.
With numerous platforms offering unique features and capabilities, selecting the right one can be challenging. Here’s an in-depth look at the top AI platforms for data science and machine learning in 2024.
1. Google Cloud AI Platform
Google Cloud AI Platform remains one of the leading data science and machine learning choices. It provides a comprehensive suite of tools and services for building, training, and deploying models at scale. The platform is equipped with integrated development environments (IDEs) like JupyterLab and offers support for popular frameworks such as TensorFlow and PyTorch.
Google Cloud AI Platform’s AutoML tool allows users to automate tasks like model selection and hyperparameter tuning, which reduces the complexity of building high-performing models. The platform also supports end-to-end ML workflows, from data preparation to model deployment, making it ideal for enterprises looking to streamline their AI projects.
Additionally, the platform’s advanced features like Explainable AI and the ability to deploy models on Vertex AI enhance transparency and scalability. This makes Google Cloud AI Platform a preferred choice for businesses seeking both innovation and stability in their AI initiatives.
2. Microsoft Azure Machine Learning
Microsoft Azure Machine Learning is another top-tier platform known for its flexibility and enterprise-grade capabilities. It offers a range of tools that cater to both novice and experienced data scientists. With Azure’s automated machine learning (AutoML) feature, users can create models without extensive coding knowledge.
Azure Machine Learning integrates well with other Microsoft services like Power BI and Microsoft 365, providing a seamless experience for companies already within the Microsoft ecosystem. The platform’s support for MLOps (Machine Learning Operations) allows for robust lifecycle management of ML models, from development to deployment and monitoring.
Azure also provides access to pre-built models through Azure Cognitive Services, enabling businesses to quickly incorporate AI into their applications. Its compliance with industry standards and ability to handle large-scale deployments make Azure Machine Learning an excellent choice for enterprises focused on security and scalability.
3. Amazon SageMaker
Amazon SageMaker is one of the most widely used platforms for machine learning due to its extensive range of features and seamless integration with other AWS services. It simplifies the ML process with tools for building, training, and deploying models, all within a single interface.
SageMaker’s strength lies in its modular design, allowing users to choose the components they need. The platform supports various ML frameworks such as TensorFlow, MXNet, and scikit-learn, along with built-in algorithms for common tasks like classification, regression, and clustering.
SageMaker also offers SageMaker Studio, a fully integrated development environment for machine learning, and SageMaker Ground Truth, a tool for data labeling. The platform’s ability to deploy models at the edge using SageMaker Neo makes it versatile for different use cases, from cloud-based applications to edge computing.
4. IBM Watson Studio
IBM Watson Studio is a leading AI platform known for its enterprise-grade capabilities and focus on collaboration. It provides an array of tools for data preparation, model building, and deployment. Watson Studio integrates with IBM’s other data science products like Watson Machine Learning and IBM Cloud Pak for Data, creating a cohesive ecosystem for data projects.
The platform’s AutoAI feature automates the entire ML workflow, from data cleaning to model selection and optimization. It uses machine learning pipelines to identify the best models and configurations, reducing the time needed for experimentation.
IBM Watson Studio also offers support for popular languages like Python, R, and Scala, making it adaptable for different data science teams. Its focus on security, compliance, and data governance makes Watson Studio a top choice for enterprises dealing with sensitive data.
5. DataRobot
DataRobot is an AI platform specifically designed to accelerate the development and deployment of machine learning models. It features a user-friendly interface and automated machine-learning capabilities that allow non-experts to create powerful models quickly.
DataRobot’s strength lies in its automated feature engineering and model training capabilities. It evaluates hundreds of models in parallel, selecting the best-performing ones based on metrics like accuracy and efficiency. The platform’s automated insights provide transparency, making it easier to understand how models make decisions.
DataRobot also supports MLOps, enabling users to monitor, retrain, and deploy models efficiently. Its focus on automation and ease of use makes it ideal for businesses looking to implement AI solutions without a large team of data scientists.
6. H2O.ai
H2O.ai is an open-source AI platform that offers a suite of machine-learning tools and libraries. It is known for its speed and scalability, making it a popular choice among data scientists and machine learning engineers. H2O.ai supports various ML models, including gradient boosting machines, generalized linear models, and deep learning.
H2O.ai’s AutoML feature simplifies model creation by automating tasks like data pre-processing, model tuning, and feature engineering. The platform’s focus on interpretability and model transparency makes it ideal for industries with stringent compliance requirements.
The H2O Driverless AI tool accelerates the entire data science workflow, enabling users to build and deploy models in hours instead of days. Its ability to handle large-scale data and support for cloud-based deployments make H2O.ai a strong contender in the AI platform space.
7. Databricks
Databricks is a unified analytics platform that integrates data engineering, data science, and business analytics. It is built on Apache Spark and offers support for large-scale data processing and machine learning.
Databricks provides a collaborative environment with support for languages like Python, R, and SQL, making it suitable for diverse data science teams. The platform’s built-in machine learning capabilities and support for distributed computing enable users to build and train models on massive datasets.
Databricks’ MLflow integration helps manage the machine learning lifecycle, from tracking experiments to deploying and monitoring models. This makes it a powerful tool for organizations looking to streamline their data science workflows.
8. KNIME Analytics Platform
KNIME is an open-source platform that offers a visual interface for data analytics and machine learning. It supports various data sources and provides a range of pre-built components for data preparation, model building, and deployment.
KNIME’s drag-and-drop interface allows users to create complex workflows without extensive coding knowledge. It also integrates with other tools like R, Python, and Spark, making it adaptable for different use cases.
The platform’s focus on modularity and open-source nature makes it suitable for research, teaching, and enterprise applications. KNIME’s emphasis on explainability and transparency makes it a strong choice for data science teams focused on understanding model behavior.
Choosing the Right AI Platform
Selecting the right AI platform depends on factors like project requirements, team expertise, and budget constraints. Platforms like Google Cloud AI and Amazon SageMaker are ideal for large-scale deployments, while tools like DataRobot and H2O.ai focus on automation and ease of use.
Each platform offers unique strengths, whether it’s for building, training, or deploying models. Understanding these features helps organizations choose a platform that aligns with their goals and maximizes the value of their data science and machine learning initiatives.
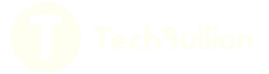