Over the last couple of decades, data analytics has become the ultimate business dialect for better decision-making, with organizations integrating some form of data science into their business operations. And the finance and accounting niches are no exception.
However, the sudden surge in analytics for the finance and accounting sector has been because of the changes in people’s expectations, technology, and market structure. More so, the accounting and finance industry has been able to streamline operations and optimize processes, improving competitiveness and efficiency. Many organizations are working to improve their data analytics to gain a competitive advantage or forecast emerging trends that may impact their businesses. To shed more light on this, we’ll be listing down some of the best uses of data analytics in the accounting and finance sector:
- Analytics in real-time
Processing of data in traditional analytics was done in batches. That is, historical data processing rather than real-time data computation. This caused various industries that rely on real-time data to gain insights into the current situation.
However, with technological advancements and the development of dynamic data pipes, it is now possible to access information with minimal delay. Organizations can use data science to track transactions and extract data from invoices, credit scores, and other financial activities.
- Automated risk management
Every business involves some risks that can severely damage profits. The digital era has produced many sophisticated technologies that can save any business from incurring unnecessary risk. Automation is one of the advanced technologies used by most financial institutions
Machine learning and data science make it possible to automate tasks and business operations. They are used in the finance industry to automate risk management. Risk management is an important business activity that aids in the analysis of flaws in a company. Security software analyzes the data to avoid flaws and discrepancies in the business. It attempts to detect unusual patterns, set up reminders, and manage information. Everything is done through the use of analytics.
Furthermore, with the help of machine learning, the software learns and enhances itself for future fault mitigation. If a financial firm detects any risk in financial activity, the software notifies and blocks the activity for security reasons. The application of data science in accounting and finance has made risk management automation much more convenient, resulting in significant improvements and growth for organizations.
- Making decisions
Finance and accounting stakeholders, executives, and traders must assess market conditions and make decisions daily. With data science, decision-making is only becoming more intelligent. Data science, powered by historical and current data, empowers financial managers to determine the viability of trading at any given time. Furthermore, these forecasts make it simple to determine whether a market is entering a bear or bull market. Knowing these factors assists financial managers in developing and investing in a viable portfolio of strategies at the appropriate time.
- Customization and deep personalization
Companies recognize that increasing interaction with customers through high-quality social ties is one of the essential components of being financially viable in today’s economy. The objective is to explore the digital client experience and make changes based on the desires and needs of the clients. AI is making significant advances in comprehending human emotion and language, allowing for a new level of client personalization. Data engineers may also develop models that evaluate consumer behavior and identify situations where clients demand financial advice.
- Product creation
FinTech (financial technology) providers are one of the fastest-growing data science users in the accounting and finance industry. This budding industry segment has only appeared recently. Still, it has taken advantage of the slower pace of change widespread in larger, more strict financial organizations.
FinTech companies with an obstructive start-up mentality offer exciting innovations faster than international firms can manage. While many fintech companies have initiated digital banks, others have concentrated on specific technology fields before selling them. Data-driven finTech includes cryptocurrency and blockchain, lending software, analytics-driven trading apps, and AI-based insurance products, to name a few examples.
- Customer data administration
Data science enables efficient customer data management. In comparison, many financial firms may have been handling their data solely through the machine learning capacities of (BI). In recent years, the advent of unstructured data and Big Data has rendered this method greatly less effective in visualizing risk and future trends. In short, more transactions occur each minute than ever before, meaning more important knowledge is needed to analyze.
Because of the growth of social media and new Internet of Things devices, much of this data does not fit into the previously used structured data format. With tools like data mining, natural language processing, text analytics, and natural language processing, businesses will be well-equipped to handle massive amounts of unformatted data. So, data science ensures that a company’s analytical capabilities can improve, resulting in greater insight into market trends and consumer behavior.
- Trading algorithms
The most important aspect of financial institutions is algorithmic trading. Algorithmic trading employs complicated mathematical formulas and lightning-fast computations to assist financial firms in developing new trading systems. Big data has significantly impacted algorithmic trading, with data science emerging as its most significant characteristic. The data in algorithmic trading comprises massive data creeks and is accompanied by a model that attempts to measure and describes the fundamental data streams. The analytical engine aims to make future market predictions based on a better understanding of massive datasets.
- Detection of fraud
Financial institutions are concerned about fraud. The risks of fraud have increased as the number of transactions has risen. However, with the advancement of analytical tools, companies can now keep track of frauds. Credit card fraud is one of the most common types of fraud in financial institutions. This type of fraud is being detected due to algorithm advancements.
Additionally, these detections notify businesses about oddities in financial purchases, urging them to block the account to diminish the loss. Many machine learning tools can also identify uncommon movements in trading data and notify financial institutions to investigate further. Banks must deal with other insurance-related frauds. Companies can isolate and cluster data patterns that appear to be highly suspicious using a variety of clustering algorithms.
Conclusion
So these are some of the uses of data analytics in accounting and finance. Data science can help enterprises in the financial industry in resolving day-to-day problems. Additionally, data science ultimately streamlines business strategies through real-time analytics, deep interrogation of trends and data, and advanced algorithms. It is fair to say that ignoring the prevalence of data analytics would only mean failure.
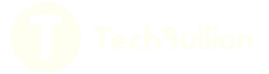