In this modern era, Artificial Intelligence (AI) has undergone a dramatic transformation, and at the heart of this evolution is vector embeddings—a mathematical breakthrough enabling machines to process language and images with unprecedented accuracy. Vijay Vaibhav Singh, a distinguished researcher, explores the intricate foundations of vector embeddings, tracing their journey from early word representations to modern transformer-based architectures.
Decoding Meaning Through Mathematical Vectors
Machines have long struggled to comprehend human language, but vector embeddings have changed the game. By representing words, phrases, and concepts in continuous vector spaces, AI can now capture complex semantic relationships. This mathematical innovation allows algorithms to detect subtle nuances in language, paving the way for more sophisticated natural language processing (NLP) systems.
The transformation began with models like Word2Vec, which introduced a method to train word vectors efficiently, demonstrating remarkable capabilities such as recognizing analogies like “King – Man + Woman = Queen.” This ability to model relationships within a high-dimensional space has since evolved into deeper learning techniques, significantly enhancing AI’s understanding of language.
From Static Words to Contextual Understanding
Early embedding models treated words in isolation, but modern AI demands more. Transformer-based models have revolutionized this space by generating context-aware embeddings. Unlike static representations, these embeddings adjust dynamically based on surrounding words, leading to significant advancements in machine comprehension.
Techniques such as BERT (Bidirectional Encoder Representations from Transformers) refine this process by analyzing words in both forward and backward directions, greatly improving AI’s ability to understand context. This approach enables more accurate responses in applications like chatbots, search engines, and automated translation systems.
Beyond Words: Vector Embeddings in Computer Vision
Vector embeddings are not limited to text; they also play a crucial role in visual recognition. AI can now analyze images by breaking them into numerical representations, capturing essential features like shapes, colors, and textures. This process enhances tasks such as image classification, facial recognition, and medical diagnostics.
One significant breakthrough is the Vision Transformer (ViT), which adapts transformer models for image processing. By dividing images into smaller sections and analyzing each component separately, ViT surpasses traditional convolutional neural networks (CNNs) in recognizing patterns and objects. This has wide-ranging applications, from autonomous vehicles to industrial quality control.
Scaling AI with Efficient Computing
As AI models grow in complexity, their computational demands increase. Efficient training techniques have become essential to handling large-scale embeddings. Researchers have developed methods like hierarchical softmax and subsampling, which optimize memory usage and accelerate training speeds without sacrificing accuracy.
Advancements in hardware acceleration, such as GPU-based implementations, have also propelled vector embeddings to new heights. By leveraging parallel processing, AI can now perform similarity searches across billions of vectors within milliseconds. This enables real-time recommendations, fraud detection, and even medical imaging analysis at scale.
The Future of Adaptive AI
One of the most exciting frontiers in AI is the shift toward adaptive embeddings—models that evolve with time. Instead of static representations, future embeddings will continuously update based on new data, improving AI’s ability to handle dynamic language, trends, and evolving user behaviors.
Multimodal embeddings, which integrate text, images, and audio into a unified space, represent another breakthrough. This approach is unlocking new possibilities in fields like content recommendation, virtual assistants, and even creative AI, where machines generate images and music based on contextual inputs.
Expanding AI’s Capabilities with Multimodal Learning
Modern AI systems are no longer confined to a single type of data. By combining text, images, and even audio, vector embeddings enable machines to achieve a deeper understanding of context. This innovation is paving the way for more intuitive virtual assistants, seamless cross-lingual translations, and smarter AI-driven applications in fields like education, entertainment, and personalized healthcare solutions.
In conclusion, Vector embeddings have become the backbone of modern AI, enabling machines to understand and process human language, images, and even complex reasoning. From early word representations to cutting-edge transformer models, these innovations have pushed the boundaries of what AI can achieve. Vijay Vaibhav Singh’s research highlights the transformative impact of embeddings, pointing toward a future where AI continues to bridge the gap between human intelligence and computational efficiency.
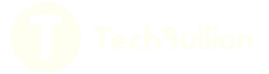