Equipment downtime is more than just an inconvenience—it’s a serious financial burden. Organizations across manufacturing, energy, transportation, and many other sectors are actively seeking solutions to reduce unplanned outages. Among the most revolutionary solutions is Artificial Intelligence (AI), specifically in the realm of predictive maintenance (PdM). By leveraging data and advanced analytics, AI helps businesses forecast equipment failures before they occur, resulting in reduced maintenance costs, improved efficiency, and extended asset life.
Understanding Predictive Maintenance in the Context of Artificial Intelligence Integration
Traditional maintenance strategies have largely revolved around two methods: reactive maintenance, where repairs are made after a breakdown, and preventive maintenance, which relies on scheduled servicing. While both methods have their place, they are not always cost-effective and can either lead to excessive maintenance or unexpected failures.
Predictive maintenance, on the other hand, is data-driven. It uses real-time data from various sensors and IoT devices to determine the condition of equipment. AI enhances this process by analyzing vast amounts of data to predict failures with high accuracy. In essence, AI makes predictive maintenance smarter and more proactive.
How AI Powers Predictive Maintenance: Key Technologies and Methods That Drive Success
The integration of AI into predictive maintenance systems involves several advanced technologies. Let’s look at the core components driving this transformation.
Machine Learning Algorithms for Pattern Recognition and Anomaly Detection
Machine Learning (ML), a subset of AI, plays a pivotal role. These algorithms learn from historical and real-time data to identify patterns and detect anomalies. For instance, if a piece of machinery begins to vibrate unusually, the ML model will compare this against historical norms and flag it as a potential problem.
Over time, these models become increasingly accurate, learning from each instance to improve future predictions. This adaptive capability makes AI-driven systems significantly more reliable than static rule-based approaches.
Internet of Things (IoT) and Sensor Networks to Capture Real-Time Operational Data
AI in predictive maintenance relies heavily on IoT devices and sensors. These sensors monitor various machine parameters—like temperature, vibration, pressure, and noise—providing real-time data to the AI system.
When integrated with AI, this data helps build a comprehensive picture of a machine’s health. Not only does this reduce human error, but it also ensures that decisions are based on empirical evidence rather than guesswork.
Cloud Computing and Big Data Infrastructure for Scalability and Storage
Handling massive volumes of sensor data requires a robust infrastructure. Cloud computing platforms offer the necessary scalability, allowing organizations to process and store terabytes of information without needing on-premise servers.
Moreover, these platforms facilitate centralized analytics, meaning data from multiple sites can be analyzed in one place. This centralization enhances decision-making and ensures consistency across operations.
Key Benefits of Using Artificial Intelligence in Predictive Maintenance Strategies
The value AI brings to predictive maintenance is undeniable. Below are some of the most prominent benefits businesses are reaping:
Significant Reduction in Unplanned Downtime
One of the greatest advantages of AI-driven predictive maintenance is the dramatic decrease in equipment downtime. With early warnings of component wear or potential failures, companies can act before a breakdown occurs. This allows for scheduled maintenance that doesn’t interfere with production cycles.
Optimized Maintenance Schedules and Cost Savings
Traditional preventive maintenance often leads to over-maintenance, where components are replaced or serviced before it’s truly necessary. With AI, maintenance is only carried out when the data indicates a need. This optimized approach reduces labor costs, spare part usage, and machine wear due to unnecessary interventions.
Improved Safety and Regulatory Compliance
Predictive maintenance not only preserves machinery but also ensures worker safety. Malfunctioning equipment can pose serious risks. Early detection mitigates these risks, leading to safer working environments. Furthermore, it helps companies maintain compliance with safety and environmental regulations, avoiding potential fines or reputational damage.
Extended Asset Lifespan Through Proactive Interventions
By detecting problems early, AI helps in extending the life of equipment. Early repairs prevent minor issues from turning into catastrophic failures, allowing businesses to maximize their return on asset investments.
Enhanced Decision-Making with Actionable Insights and Reporting
AI provides not just data, but actionable insights. Maintenance teams receive clear recommendations on what needs attention, when, and why. This data-backed approach enhances decision-making across the board—from technicians to executives.
Overcoming the Challenges of Implementing AI in Predictive Maintenance Workflows
Despite its numerous benefits, adopting AI for predictive maintenance is not without hurdles. Organizations need to address these challenges head-on for successful implementation.
Data Quality and Integration Complexity
The effectiveness of AI models depends on the quality of input data. Inconsistent or incomplete data from various legacy systems can hinder model accuracy. Integration of AI platforms with existing enterprise systems like ERP and SCADA also requires careful planning and technical expertise.
High Initial Investment and ROI Concerns
Setting up an AI-based PdM system involves substantial initial investments in sensors, software, infrastructure, and training. Companies must consider the long-term ROI rather than focusing only on short-term costs. Gradual deployment through pilot projects can help manage risk and demonstrate value.
Workforce Upskilling and Cultural Shifts
Maintenance teams need to be trained to interpret AI-driven insights and incorporate them into their workflows. Additionally, organizations may need to overcome resistance to change, particularly in industries where traditional methods have dominated for decades.
Future Trends: What to Expect from AI in Predictive Maintenance Going Forward
The integration of AI in predictive maintenance is only in its early stages. As technology evolves, we can expect even more sophisticated solutions.
The Rise of Autonomous Maintenance Systems and Digital Twins
Future systems may become autonomous, where AI not only predicts failures but also initiates corrective actions without human intervention. Digital twins, which are virtual replicas of physical systems, will allow for even more precise simulations and predictions.
Edge AI for Real-Time Localized Decision-Making
Edge computing will allow AI models to run directly on local devices, reducing latency and dependence on cloud connectivity. This is particularly useful in remote locations or environments with unstable internet access.
Integration with Augmented Reality (AR) for Maintenance Assistance
Technicians could use AR headsets powered by AI to receive real-time guidance while performing repairs. This enhances productivity and reduces the likelihood of errors during complex maintenance tasks.
Conclusion
AI is no longer a futuristic concept—it’s a practical tool delivering measurable benefits today. From reducing downtime to cutting costs and improving safety, the role of AI in predictive maintenance is transforming industries across the board. However, successful adoption requires strategic planning, quality data, and skilled personnel.
Businesses that invest in AI-powered predictive maintenance now are positioning themselves for long-term competitiveness and resilience. Furthermore, we can expect AI to become even more embedded in the operational fabric of modern industry, delivering smarter, more efficient, and more reliable maintenance solutions.
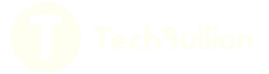