Over the past year, it’s been increasingly clear that generative AI has the capacity to transform the manufacturing sector. With efficiency gains and promises of innovation, the technology is expected to create novel solutions and designs on the fly. Whether this will be the case or not remains to be seen.
The global AI in manufacturing market is projected to reach $20.8 billion by 2028, according to a MarketsandMarkets report, which will be up from just over $3 billion in 2023.
With 87% of manufacturers claiming to have adopted AI (or are planning to within two years), the race for generative AI solutions in manufacturing is intensifying.
Current Trends in Generative AI for Manufacturing
The manufacturing industry is witnessing a surge in generative AI adoption. OpenAI’s GPT-4 has received the most attention. However, it appears to currently be suited to more chat-based assistant roles, rather than playing any significant role in IT systems or operations. Major players like Siemens are instead partnering with tech giants like NVIDIA to develop industrial metaverse applications, while general investment into AI is rising across the board.
Another trend of note is the focus on sustainability, with over 25% of manufacturing facilities globally expected to employ generative AI for energy optimisation by 2024. This shift may appear pre-emptive regarding global concerns around corporate responsibility, but it’s actually a reactive response to reports that we may have energy limitations concerning AI usage.
Underlying the leveraging of AI is a foundation of good quality and organised data. High-performing data warehouse solutions have been on the rise because it provides this infrastructure for data insights, and potentially bespoke AI training.
Key Use Cases of Generative AI in Manufacturing
Product Design and Optimisation
Autodesk’s generative design software for aerospace components shows the extent of how AI can create lightweight and efficient designs. The technology has helped speed up the exploration of alternative designs, which helps reduce development time and costs.
Predictive Maintenance
Rolls-Royce’s use of AI for jet engine maintenance prediction is where AI proves itself to be useful in foreseeing equipment failure before it happens. By analysing vast amounts of sensor data, AI can predict maintenance needs and reduce downtime, which also extends the lifespan of machinery.
Quality Control and Defect Detection
Landing AI’s visual inspection system for PCB manufacturing has demonstrated that AI can be useful in identifying very minute defects. It helps to boost quality assurance processes, which again reduces waste and improves product reliability.
Supply Chain Optimisation
According to McKinsey, 61% of executives report decreased costs. Generative AI can simulate various supply chain scenarios, optimising inventory levels and predicting demand fluctuations.
Process Optimisation
BMW have been using AI simulations to optimise paint shop processes. By creating digital twins of production lines, the manufacturers can test and refine processes virtually before implementation.
Benefits of Implementing Generative AI in Manufacturing
According to a McKinsey report, AI could boost labour productivity by 0.1 to 0.6 percent annually, all the way through to 2040. Labour productivity is the basis of the macroeconomy, in which we need gains in productivity in order to boost global wealth. On a micro scale, labour productivity boosts efficiency and reduces the marginal cost of producing an additional unit.
Cost reduction and resource optimisation can be passed onto the customer, along with a better product overall. GE’s use of generative design to create lighter, more efficient turbine parts was a good example of this. The technology enables a much faster exploration of design alternatives, so prototyping becomes much easier. This also overcomes the main bottleneck in innovation, beyond legal obstacles.
Improved decision-making and risk management are also possible benefits that come from AI. AI-powered tools provide real-time monitoring and risk analysis of manufacturing operations, enabling quick identification and resolution of issues. As mentioned with Enterprise Data Warehouse solutions, there is already a solid base for data visualisation to springboard from, but AI may speed up how quickly reports are generated using such data.
Lastly, generative AI contributes to sustainability efforts. This is a big part of the global alignment to ESG goals, particularly with ethical investors, meaning that firms can make it onto ESG funds and boost their appeal. Unilever and Alibaba have created an AI-powered waste reduction initiative for factories to automatically identify and sort plastic packaging.
Risks in Adopting Generative AI
Despite its potential, adopting generative AI in manufacturing faces several challenges. Data quality and availability are primary concerns, and when using AI trained on third-party data, it may have less relevance to your own operations. Many manufacturing organisations, having grown through mergers and acquisitions, struggle with legacy systems and siloed data.
With this also comes the problem of data security and regulation. Sensitive data cannot be trained by third-party AI models because it may be regurgitated. AI will therefore need to be local, or strong security measures must be in place to safeguard this data.
Final Word
The future of generative AI in manufacturing is promising. We are already seeing some productivity gains, with many analysts predicting that these gains will not plateau for decades to come. The bottleneck will be creating AI models that have transferable relevance while also safeguarding data.
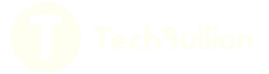