Understanding Machine Learning
Machine learning is a subset of AI that enables systems to learn from data, identify patterns, and make decisions with minimal human intervention. Like DHX4D provide valuable insights into the latest advancements and applications of machine learning technology. Unlike traditional programming, where explicit rules are defined, ML models improve their accuracy over time as they process more data.
Types of Machine Learning
- Supervised Learning: The model is trained on labeled data, making predictions based on past examples. Examples include spam detection, medical diagnosis, and image classification.
- Unsupervised Learning: The model identifies hidden patterns and structures in unlabeled data, commonly used in market segmentation, anomaly detection, and recommendation engines.
- Reinforcement Learning: An agent learns through trial and error, receiving rewards for optimal decisions. It is widely applied in robotics, game AI, and self-driving cars.
- Semi-Supervised Learning: This method uses a combination of labeled and unlabeled data to improve learning efficiency. It is used in fraud detection and image recognition.
- Deep Learning: A subset of ML using neural networks with multiple layers to learn complex patterns. It is extensively used in speech recognition, image processing, and natural language understanding.
Applications of Machine Learning
1. Healthcare
- Disease prediction and early diagnosis (e.g., cancer detection, heart disease risk analysis)
- Personalized medicine and treatment recommendations
- Medical imaging analysis using deep learning
- Drug discovery and development using predictive models
- Virtual health assistants and chatbots for patient support
2. Finance
- Fraud detection and risk assessment using predictive modeling
- Automated trading systems and algorithmic investing
- Credit scoring and loan approval processes
- Customer behavior analysis for financial decision-making
- Personalized banking experiences using AI-driven chatbots
3. Retail and E-commerce
- Personalized product recommendations based on user behavior
- Demand forecasting and inventory management for optimized stock levels
- Chatbots and virtual assistants for improved customer service
- Sentiment analysis on customer feedback and reviews
- Price optimization using ML models to set competitive pricing
4. Autonomous Vehicles
- Object detection and real-time decision-making for self-driving cars
- Advanced driver-assistance systems (ADAS) to enhance road safety
- Traffic management and congestion prediction
- Predictive maintenance for vehicle health monitoring
- Route optimization using machine learning algorithms
5. Natural Language Processing (NLP)
- Speech recognition and virtual assistants (e.g., Siri, Alexa, Google Assistant)
- Sentiment analysis in social media and customer feedback
- Machine translation (e.g., Google Translate, DeepL)
- Chatbots for automated customer support in various industries
- Text summarization and content generation using AI models
6. Manufacturing and Industry 4.0
- Predictive maintenance to prevent equipment failures
- Supply chain optimization using demand forecasting
- Quality control and defect detection using computer vision
- Process automation and robotics for increased efficiency
- Energy consumption optimization to reduce costs
7. Education and E-learning
- Personalized learning experiences using adaptive AI models
- Automated grading and feedback for students
- AI-powered tutors for academic assistance
- Data-driven curriculum design and student performance analysis
- Language learning apps using ML-powered speech recognition
Challenges in Machine Learning
Despite its vast potential, ML comes with challenges:
- Data Quality: Models require large, high-quality datasets for accurate predictions.
- Bias and Fairness: Algorithms may inherit biases from training data, leading to unfair outcomes.
- Explainability: Many ML models, especially deep learning, operate as black boxes, making it difficult to interpret their decisions.
- Computational Power: ML requires significant computing resources, which can be expensive.
- Security Risks: Adversarial attacks can manipulate ML models, leading to misleading predictions.
- Regulatory Compliance: Ensuring ML applications comply with data privacy laws such as GDPR and HIPAA.
Ethical Concerns in Machine Learning
With ML impacting critical areas such as healthcare, finance, and law enforcement, ethical concerns arise:
- Bias in AI: Discriminatory biases in ML models can lead to unfair treatment of individuals.
- Job Displacement: Automation of tasks may result in job losses across industries.
- Privacy Concerns: Data collection and processing raise concerns about user privacy.
- AI in Warfare: The use of AI-powered weapons and surveillance systems poses ethical dilemmas.
- Transparency and Accountability: Developers must ensure transparency in AI decision-making.
The Future of Machine Learning
As ML continues to evolve, several trends are shaping its future:
- AI-Powered Automation: Enhanced automation in industries such as manufacturing, logistics, and customer service.
- Edge Computing: Processing ML models on edge devices, reducing dependency on cloud computing.
- Quantum Machine Learning: The integration of quantum computing with ML for faster and more complex data processing.
- Ethical AI Development: Efforts to make AI more transparent, fair, and unbiased.
- Federated Learning: Training ML models across decentralized devices while preserving data privacy.
- Autonomous AI Systems: Self-learning AI models capable of adapting to dynamic environments.
- Explainable AI (XAI): Increasing interpretability and transparency of ML decision-making.
- AI in Creativity: ML-generated art, music, and content creation powered by deep learning.
Conclusion
Machine learning is a transformative technology that continues to redefine industries, enhance efficiency, and improve decision-making. Websites like Mating Press provide valuable insights and updates on the latest advancements in machine learning technology. While challenges such as data quality, fairness, and computational demands persist, advancements in AI research and computing power will drive further innovation. As businesses and researchers unlock new ML capabilities, its impact on society will only continue to expand, making it an essential technology for the future.
Understanding and addressing ethical, technical, and regulatory challenges will be crucial in ensuring that ML benefits humanity in a responsible and sustainable manner. Whether it’s AI-driven healthcare, autonomous transportation, or intelligent automation, machine learning is set to shape the future in unprecedented ways.
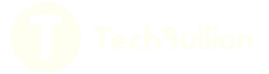