In a world increasingly driven by artificial intelligence, the importance of diversity in data cannot be overstated. From biased algorithms to exclusionary practices, ensuring fairness in AI models is crucial for creating a more equitable and just society. Join us as we explore the significance of diverse data sets and discover how we can take action to promote inclusivity and equality in our digital landscape.
Introduction to AI and its Impact on Society
Artificial intelligence (AI) has quickly become an integral part of our daily lives. From voice assistants like Siri and Alexa, to self-driving cars, to personalized recommendations on streaming services, AI is all around us. But what exactly is AI and how does it impact society?
In simple terms, AI refers to the development of computer systems that can perform tasks that typically require human intelligence. These tasks can range from analyzing data and making decisions, to recognizing patterns and understanding speech. With advancements in technology, AI has greatly expanded its capabilities and potential applications.
The impact of AI on society is vast and far-reaching. It has brought numerous benefits such as increased efficiency in industries like healthcare and finance, improved accuracy in decision-making processes, and enhanced convenience for consumers through automation. However, it also raises ethical concerns regarding fairness, bias, and discrimination.
One major concern with AI systems is their potential to perpetuate or amplify the biases present in the data used to train them. This can lead to discriminatory outcomes for certain groups of people based on characteristics such as race or gender. For example, facial recognition technologies have been found to have higher error rates for people with darker skin tones due to biased training datasets.
Furthermore, with the increasing use of algorithms in areas such as hiring or loan approvals, there are worries about algorithmic decision-making reinforcing existing inequalities or creating new ones. Without diversity in data representation and inclusive approaches to model development, these systems can easily perpetuate systemic biases.
Therefore, it’s crucial for organizations developing AI models to prioritize diversity in their datasets by including a wide range of perspectives and experiences that accurately reflect society’s diverse population.
Additionally, promoting diversity within teams working on AI projects can bring different viewpoints and thought processes that contribute towards creating more fair and unbiased algorithms. By having diverse teams work together throughout the development process – from data collection to testing – potential biases can be identified and addressed early on.
Understanding Bias in Data and AI Models
Bias is defined as the uneven or prejudicial treatment of individuals or groups based on certain characteristics such as race, gender, age, etc. In data and AI models, bias can result from the inherent prejudices of individuals involved in creating and implementing these systems. This can lead to discriminatory outcomes that perpetuate existing social injustices.
There are two main types of bias that can occur in data and AI models: algorithmic bias and data bias. Algorithmic bias refers to when the algorithms used to make decisions are biased themselves. Data bias, on the other hand, occurs when the training data used to develop these algorithms is skewed towards a certain group or fails to represent all segments of society equally.
Algorithmic bias can be caused by various factors such as flawed programming techniques or incomplete training data. For example, facial recognition software has been found to have higher error rates for people with darker skin tones because they were not properly represented in the initial training dataset. Similarly, language translation software has also been shown to have biases towards certain languages over others.
Data bias is often a result of historical discrimination and societal inequalities that exist within our communities. When datasets are collected without considering diversity and inclusivity, they can reflect unequal representation of different groups. This leads to biased results that favor one group over another.
The consequences of using biased algorithms and data in decision-making processes cannot be ignored. They can reinforce systemic inequalities and perpetuate discrimination against marginalized communities. For instance, if a financial institution uses biased credit scoring algorithms that favor wealthy individuals over low-income minority groups, it will only further widen the wealth gap between different socioeconomic classes.
It is essential for organizations using data-driven technologies like AI to proactively identify any potential sources of bias within their systems. This requires transparency in how algorithms are developed and implemented so that any discrepancies can be easily identified and addressed.
To ensure fairness in AI models, it is crucial to have diverse teams working on data collection, preparation, and development. This will help bring in different perspectives and ensure that the training datasets are representative of all groups within society.
The Importance of Diversity in Data
In today’s digital world, data is the foundation of many technological advancements, including artificial intelligence (AI). With the rise of AI and its increasing use in various industries, it has become crucial to recognize the importance of diversity in data. Diverse data plays a critical role in ensuring fairness and accuracy in AI models.
Data diversity refers to the inclusion of different types of data from various sources, representing a wide range of demographics, backgrounds, and perspectives. This means that the data used to train an AI model should not only come from one specific group but instead reflect real-world diversity. The lack of diversity in data can lead to biased outcomes and reinforce existing societal inequalities.
One major concern with the lack of diversity in data is how it affects AI algorithms’ decision-making processes. For example, facial recognition technology has been shown to perform poorly for people with darker skin tones due to a lack of diverse training datasets. This highlights the importance of having more inclusive and representative datasets to prevent discriminatory outcomes.
Moreover, diverse data enables AI models to make more accurate predictions and avoid biases stemming from limited perspectives or preconceived notions. By incorporating diverse voices into the dataset, it helps reduce bias towards specific groups and allows for a more comprehensive understanding of complex issues.
Another significant advantage of diversity in data is its potential impact on product innovation. As technology continues to evolve at a rapid pace, embracing diversity in data can lead to more innovative solutions that cater to diverse communities’ needs. It brings new insights and perspectives that may have previously been overlooked during product development.
Furthermore, promoting diversity in your dataset also promotes ethical values and practices within your organization. By recognizing the importance of inclusivity and representation within your dataset, you are actively working towards creating fairer AI systems that benefit everyone.
However, achieving true diversity within datasets can be challenging as it requires thoughtful planning and conscious effort from all parties involved – from data collection to model development. It is essential to identify any biases within your datasets and actively work towards addressing them.
Real-Life Examples of Bias in AI
Despite the many potential benefits and advancements that AI technology offers, it has become increasingly evident that bias is prevalent in these systems. This bias can have serious and harmful consequences, particularly when it comes to making decisions that impact people’s lives. Here are a few real-life examples of how AI bias has manifested itself:
1. Facial Recognition Technology – One major area where bias in AI has been exposed is facial recognition technology. Studies have shown that these systems tend to misidentify people with darker skin tones at a higher rate than those with lighter skin tones. This means that minority groups are more likely to be falsely accused or targeted by law enforcement based on faulty facial recognition results.
In 2018, Amazon’s facial recognition software, Rekognition, was found to incorrectly match 28 members of Congress to criminal mugshots in a test conducted by the American Civil Liberties Union (ACLU). This highlights the inherent racial biases embedded in the algorithm used for this technology.
2. Hiring and Recruitment Practices – AI-based hiring platforms have also come under scrutiny for perpetuating gender and racial biases. In one high-profile case, Amazon had to scrap an experimental recruiting tool because it was consistently biased against female candidates.
The algorithm was trained on resumes submitted over a 10-year period which were mostly from male applicants since the tech industry has historically been dominated by men. As a result, the system learned to favor male candidates over females, even when their qualifications were similar.
3. Loan Approval Systems – Another example of bias in AI is seen in loan approval systems used by banks and financial institutions. These algorithms use historical data as inputs to determine creditworthiness and risk assessment of loan applicants.
However, this data may contain systemic discrimination towards certain marginalized groups due to factors such as redlining or discriminatory lending practices from previous years. As a result, these algorithms may unfairly deny loans or offer unfavorable terms based on factors beyond the individual’s control.
Detecting and Mitigating Bias in AI Models
Detecting and mitigating bias in AI models is crucial for ensuring fairness and ethical use of artificial intelligence. As we mentioned earlier, AI systems are only as good as the data they are trained on, and if the data is biased, then the results produced by these models will also be biased. This can lead to discriminatory practices and reinforce existing societal biases.
Therefore, it is crucial to have mechanisms in place that can detect and mitigate bias in AI models. One way to achieve this is through a diverse team of individuals working on developing and deploying these models. A diverse team brings different perspectives and ideas to the table, which can help identify potential biases in the data or algorithms being used.
Another important aspect is transparency in the development process of AI models. Companies should be open about their data sources, algorithms used, and decision-making processes so that external experts or auditors can detect any biases present in the model.
Furthermore, continuous monitoring of AI systems is essential to ensure that they do not produce biased results over time. This requires setting up benchmarks and evaluations based on different demographic groups to check for any disparities or discriminatory outcomes.
One technique for detecting potential biases in AI models is using audit frameworks such as FairML or Themis-ML. These frameworks allow researchers to assess various metrics related to fairness and identify any potential sources of bias within an algorithmic system.
Once a bias has been identified, steps must be taken to mitigate its impact. One approach could be re-evaluating the training datasets and identifying problematic data points that could contribute to bias. Another solution could involve introducing diversity into training datasets by including more diverse voices, opinions, and experiences.
Moreover, it may also be necessary to modify certain features or parameters within the model itself to reduce bias. However, this needs to be done carefully without compromising on accuracy or performance.
Steps to Ensure Fairness in AI Models
When it comes to building AI models, ensuring fairness is crucial. Fairness ensures that the AI model does not discriminate against any group of people and provides equal opportunities for all. This is especially important in today’s world where AI is being used in various decision-making processes, from hiring to loan approvals.
To ensure fairness in AI models, here are some steps that can be taken:
1. Diverse Data Collection: The first step towards ensuring fairness in AI models is to have diverse and unbiased data sets. Biased data sets will lead to biased results, which can have adverse effects on certain groups of people. Therefore, it is essential to collect data from a diverse set of sources and ensure that the data collected does not reflect any particular bias.
2. Identify Potential Bias: Once the data has been collected, it is vital to identify any potential biases present in the data. This could include demographic or historical biases that may impact the training of the AI model.
3. Conduct Regular Audits: It is essential to regularly audit the AI model for any signs of bias or discrimination throughout its development process. These audits should be conducted by a diverse team with expertise in both technology and ethics.
4. Use Multiple Algorithms: Instead of relying on just one algorithm, it is advisable to use multiple algorithms during training and testing phases. Each algorithm may have different strengths and weaknesses, allowing for a more comprehensive evaluation of fairness within the model.
5. Include Diverse Perspectives: When building an AI model, having a diverse team with varied perspectives is crucial for identifying potential biases and ensuring fair outcomes for all stakeholders involved.
6. Continual Monitoring: After an AI model has been deployed, continual monitoring should be conducted to ensure that it continues producing fair results over time. Monitoring can also help catch any new biases or discriminatory patterns that may arise as new data comes into play.
7.You Create What You Train On: As seen many times in the past, the AI model can inadvertently learn and amplify biases present in society. Therefore, it is crucial to be mindful of the data used for training an AI model as it directly impacts its decision-making abilities.
The Role of Individuals and Companies in Promoting Diversity and Fairness
One of the most crucial factors in promoting diversity and ensuring fairness in AI models is the role played by individuals and companies. In today’s society, where technology plays a significant role in our daily lives, it is essential for both individuals and companies to take responsibility for creating a diverse and fair world.
Individuals have a powerful influence on shaping how AI models are built and used. It is crucial for them to have a deep understanding of diversity and its importance in data collection, analysis, and algorithm development. As users of AI technology, individuals must demand fairness and transparency from companies that create these systems.
One way individuals can promote diversity is by actively seeking out different perspectives and experiences when collecting data. This includes considering diverse demographics such as race, gender, age, ethnicity, religion, sexual orientation, socio-economic status, disability status, etc. When data sets are more inclusive and accurately represent the population they serve, there is a higher chance of creating unbiased AI models.
Furthermore, individuals can also advocate for companies to prioritize diversity in their hiring practices. By having diverse teams working on AI projects, there will be an increased likelihood of identifying potential biases or blind spots in the system’s design process. Additionally, these teams can provide valuable insights into cultural nuances that may affect how certain groups are represented or treated in the model.
On the other hand ,companies also play a critical role in promoting diversity and maintaining fairness within their AI models. They must recognize that their products have real-world consequences on various communities; thus they should prioritize ethical considerations throughout every step of the development process.
Firstly , companies should invest resources in developing transparent algorithms that allow users to understand why certain decisions were made by the AI model. This transparency promotes trust between users and improves accountability among organizations responsible for deploying these systems.
Secondly , organizations must make sure that their data sets are diverse . Companies need to actively seek out underrepresented populations’ perspectives to ensure inclusivity in their data collection and analysis. Moreover, companies should regularly audit their AI systems to detect any potential biases and take necessary steps to address them.
Conclusion
As technology continues to advance, it is crucial to recognize the significance of diversity in data and its impact on AI models. By prioritizing diversity in our data collection and addressing any biases present, we can ensure fairness and accuracy in AI decision-making processes. It is up to individuals and organizations to take proactive steps towards promoting diversity in their data practices, ultimately leading to more inclusive and ethical technologies for all. Let us work together towards a future where everyone’s experiences are represented equally in the data that fuels our modern world.
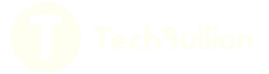