Credit scoring has always been crucial in the financial industry. Traditionally, it relied on human judgment and basic statistical methods to determine an individual’s creditworthiness. However, the advent of machine learning (ML) has revolutionized this field. By leveraging advanced algorithms and vast amounts of data, ML enhances accuracy and reduces bias, leading to more equitable financial decisions. This article delves into how ML impacts credit scoring, its benefits, and the challenges it presents.
Traditional Credit Scoring Methods
Overview of Conventional Methods
Historically, credit scoring models were built using logistic regression and decision trees. These models depended on a limited set of variables, such as credit history, income, and employment status. They used straightforward mathematical techniques to assign scores, which predicted the likelihood of default.
Limitations of Traditional Models
While effective to an extent, traditional methods have significant drawbacks. They often fail to account for the complexity of financial behaviors. Their reliance on a narrow set of variables can lead to inaccuracies. Additionally, these models may perpetuate existing biases, as they reflect the subjective decisions made by human analysts.
Introduction to Machine Learning in Credit Scoring
What is Machine Learning?
Machine learning is a subset of artificial intelligence (AI) that involves training algorithms to recognize patterns and make predictions based on data. Unlike traditional programming, where rules are explicitly defined, ML models learn from data. They improve over time as they are exposed to more information.
How Machine Learning Enhances Credit Scoring
ML algorithms can analyze vast amounts of data, uncovering patterns that traditional models might miss. They use features like transaction history, spending patterns, and social media activity. These algorithms can continuously learn and adapt, resulting in more accurate predictions.
Benefits of Machine Learning in Credit Scoring
Enhanced Accuracy
One of the primary advantages of ML in credit scoring is its ability to enhance accuracy. Traditional models are limited by the variables they use. In contrast, ML algorithms consider thousands of data points, leading to a more comprehensive analysis of an individual’s creditworthiness. This results in more precise predictions and fewer instances of misclassification.
Reduction of Bias
Bias in credit scoring has been a longstanding issue. Human judgment can be influenced by unconscious biases, leading to unfair treatment of certain groups. ML algorithms, when properly trained, can help mitigate this problem. By focusing on data-driven insights rather than subjective opinions, they can provide more equitable assessments. However, it is crucial to ensure that the training data itself is free from bias to avoid perpetuating existing inequalities.
Real-Time Analysis
Traditional credit scoring models are often static, based on historical data. ML, on the other hand, enables real-time analysis. This means that credit scores can be updated continuously, reflecting the most current financial behaviors. For example, if an individual makes a large payment or incurs significant debt, their credit score can be adjusted accordingly. This dynamic approach provides a more accurate picture of an individual’s financial health.
Applications of Machine Learning in Credit Scoring
Feature Engineering
Feature engineering involves selecting and transforming variables to improve model performance. In ML, this process is automated and sophisticated. Algorithms can identify relevant features from raw data, enhancing predictive accuracy. For example, they might recognize that certain spending patterns are indicative of financial stability or risk.
Alternative Data Sources
ML models can incorporate alternative data sources, such as utility payments, rental history, and social media activity. These additional data points can provide a more holistic view of an individual’s financial behavior, particularly for those with limited credit history. This inclusivity helps extend credit access to underserved populations.
Predictive Modeling
Predictive modeling in ML goes beyond traditional statistical methods. Techniques like neural networks, random forests, and gradient boosting can identify complex relationships between variables. These advanced models offer superior predictive power, reducing the likelihood of default and improving overall loan performance.
Challenges and Ethical Considerations
Data Privacy Concerns
The use of ML in credit scoring raises significant data privacy concerns. Collecting and analyzing vast amounts of personal information can lead to potential misuse or breaches. Financial institutions must ensure robust data protection measures are in place to safeguard sensitive information.
Transparency and Explainability
ML algorithms, particularly deep learning models, can be opaque and difficult to interpret. This lack of transparency can be problematic in credit scoring, where individuals have the right to understand how their scores are determined. Regulators and stakeholders must demand transparency and explainability in ML models to ensure accountability.
Risk of Bias in Training Data
While ML can reduce bias, it is not immune to it. If the training data used to develop these models is biased, the resulting predictions will also be biased. Financial institutions must carefully curate and audit their datasets to eliminate any sources of bias and ensure fair treatment for all individuals.
Future of Machine Learning in Credit Scoring
Continuous Improvement and Adaptation
ML models are not static; they evolve over time. As more data becomes available, these models can be retrained and refined to improve their accuracy and fairness. This continuous improvement process ensures that credit scoring methods remain relevant and effective in a rapidly changing financial landscape.
Integration with Financial Technologies
The integration of ML with other financial technologies, such as blockchain and digital identity verification, can further enhance credit scoring. For instance, blockchain can provide a secure and transparent way to verify financial transactions, while digital identity verification can ensure the accuracy of personal information. These advancements can lead to more robust and reliable credit scoring systems.
Regulatory Developments
As ML becomes more prevalent in credit scoring, regulatory frameworks will need to adapt. Policymakers must balance the benefits of ML with the need for consumer protection.
Conclusion
In conclusion, the integration of machine learning into credit scoring marks a significant advancement in the financial industry. By improving accuracy and reducing bias, ML has the potential to create a more inclusive and equitable financial system. However, careful attention must be paid to the ethical and regulatory implications to ensure that these benefits are realized without compromising individual rights and privacy. As we move forward, it is crucial to strike a balance between innovation and responsibility, paving the way for a fairer and more transparent credit scoring landscape.
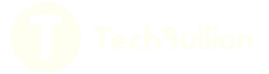