In the modern digital era, only a handful of technologies have driven as much innovation as artificial intelligence (AI). However, AI’s rapid growth comes with concerns about its environmental impact, particularly regarding the energy-intensive nature of GPUs (Graphics Processing Units). While GPUs are often seen as major contributors to AI’s increasing power consumption, research by Aditya Avinash Atluri presents a more nuanced perspective. An expert in AI hardware efficiency highlights that while GPU power usage has risen.advancements in architecture, optimization techniques, and parallel processing efficiency have significantly improved their energy-to-performance ratio. This evolution is reshaping the way AI models are trained and deployed, ensuring sustainable progress in the field.
Rethinking AI’s Energy Footprint
The widespread belief that GPUs are inefficient stems from outdated comparisons with CPU-based systems. While AI workloads demand high computational power, GPUs offer a superior performance-to-power ratio. Modern architectures achieve exponentially higher computational throughput with only modest increases in energy consumption. For example, a comparison of GPU generations from Pascal to Blackwell shows a 256-fold improvement in AI training performance, while power consumption has only doubled.
The Role of GPUs in Deep Learning Acceleration
AI model training and inference require immense parallel processing capabilities, making GPUs the preferred choice over CPUs. A benchmarking study found that GPU-accelerated AI training is up to 940 times faster than CPU-based implementations, significantly reducing the time required to develop and deploy machine learning models. This acceleration translates to practical benefits, such as reducing model training from weeks to mere hours while consuming considerably less power.
Synthetic Data Generation: Reducing AI’s Carbon Footprint
One of the most energy-intensive aspects of AI development is data generation and labeling. GPUs have revolutionized this process by enabling synthetic data generation, producing up to 1.2 million labeled training samples per hour. This innovation not only eliminates the need for manual data labeling but also reduces the computational energy required to process vast datasets, cutting training time by over 97% compared to traditional CPU-based methods.
The Evolution of GPU Efficiency
The evolution of GPU efficiency is evident in the transition from older architectures to modern designs. For example, the Blackwell architecture delivers 10,000 GFLOPS per watt, a substantial improvement over Pascal’s 10.6 GFLOPS per watt. This significant boost in energy efficiency enables AI developers to process larger datasets while consuming considerably less energy. The enhanced efficiency not only accelerates AI advancements but also supports sustainable and scalable growth, making modern GPUs an essential component for the future of AI development.
Challenging Traditional Computing Models
CPU-based AI infrastructures struggle to match the efficiency of GPU-accelerated systems. A study analyzing research computing facilities found that CPU-only AI workloads required 3.7 times more rack space and 4.2 times more cooling capacity than GPU-powered alternatives. Additionally, maintaining CPU-based AI clusters demands 7.8 times more power distribution infrastructure, making GPUs a far more energy-efficient option for large-scale AI deployments.
The Economic and Environmental Impact of GPU Acceleration
Switching to GPU-based AI systems yields significant economic and environmental benefits. Research computing facilities operating on CPU-only infrastructure spend an average of €4.2 million annually on electricity, whereas GPU-powered systems reduce this cost to €0.89 million. Additionally, GPU-accelerated AI models reduce carbon emissions by up to 82%, contributing to more sustainable AI research and development.
The Future of AI Hardware Efficiency
As AI continues to evolve, the need for power-efficient computing solutions will become even more pressing. While GPU power consumption remains a topic of discussion, the overall efficiency gains, reduced training times, and lower infrastructure demands solidify GPUs as the cornerstone of sustainable AI development. With ongoing architectural innovations, future GPUs are expected to push computational efficiency even further, enabling AI advancements while minimizing their environmental impact.
In conclusion, Aditya Avinash Atluri’s research dispels common misconceptions about GPU energy consumption, demonstrating that these processors are not just necessary for AI’s rapid growth but also instrumental in making AI development more energy-efficient. As the world moves toward more sustainable computing practices, GPUs will continue to play a pivotal role in balancing performance with environmental responsibility.
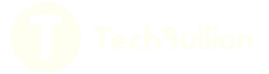