Welcome to the fascinating world of Generative AI, where machines have been programmed to tap into their creativity and produce awe-inspiring works that rival those made by humans. As we delve deeper into this captivating topic, let’s fast-forward our thoughts to 2024 and take a closer look at what lies ahead for Generative AI. Brace yourselves, because the future promises mind-boggling advancements and astonishing possibilities that are sure to revolutionize various industries. So, join us on this exhilarating journey as we unveil the incredible potential that awaits us in the realm of generative artificial intelligence!
What is Generative AI?
Generative artificial intelligence (AI) is a rapidly advancing field that combines machine learning, mathematics, and computer science to create algorithms that can generate content. This content can range from text and images to audio and video, with the ultimate goal of creating original and realistic output that reflects human creativity.
The term “generative” refers to the ability of these algorithms to produce new and unique content based on patterns they have learned from large datasets. This is in contrast to traditional AI techniques which rely on pre-programmed rules or instructions. Generative AI systems are able to learn from data and make decisions on their own, without explicit instructions from a programmer.
One of the key components of generative AI is its ability to mimic human cognition. This means that instead of simply following predefined rules, these algorithms can analyze information, make connections between different concepts, and create something new based on their understanding.
There are several subfields within generative AI including natural language processing (NLP), computer vision, speech recognition, and music generation. Each of these focuses on generating specific types of content using different approaches.
In NLP for example, generative models use deep learning techniques such as recurrent neural networks (RNNs) and transformer models to generate text that mimics human-written language. These models work by analyzing large amounts of text data (such as books or articles) and learning the statistical patterns behind them. They can then generate new text based on this knowledge.
Past and Present of Generative AI
1. Past of Generative AI:
Generative AI or Artificial Intelligence has been around for more than six decades, but it was not until recent advancements in technology that the concept of generative AI gained widespread attention and recognition. The term “generative” refers to the ability of the AI system to create new and original content based on its learning and understanding of data.
In the past, generative AI was primarily used in areas such as natural language processing and image recognition. For instance, IBM’s “Deep Blue” became famous in 1997 when it defeated chess champion Garry Kasparov, showcasing the potential of AI to outperform human intelligence.
However, generative AI remained largely limited to academic research due to technological limitations such as computing power and availability of vast amounts of data. It wasn’t until deep learning algorithms were invented in the mid-2000s that generative AI started gaining traction.
2. Present State of Generative AI:
With advancements in deep learning and an abundance of data available through social media platforms, there has been a significant surge in generative AI applications in recent years.
One prominent example is OpenAI’s GPT-3 (Generative Pre-trained Transformer 3), released in 2020. It is currently one of the largest and most sophisticated language models with 175 billion parameters, capable of generating highly convincing news articles, essays, poetry, etc., almost indistinguishable from human-written texts.
Similarly, other companies are also using gener ative AI for various applications, such as Netflix’s content recommendation system and Google’s image recognition software. Generative AI is also being utilized in fields like medicine, finance, and advertising.
3. Future of Generative AI:
The future of generative AI looks promising with the ongoing advancements in technology. With the increasing availability of large datasets and more advanced deep learning algorithms, generative AI is likely to become more sophisticated and capable.
One potential area where generative AI can make a significant impact is creative industries such as art, music, and design. Companies are already exploring ways to use generative AI to create original pieces of art or conduct symphonies. In the future, we might see entirely computer-generated movies or video games.
Moreover, with the rise of conversational AI and chatbots, there will be an increasing need for generative AI to produce human-like responses based on contextual understanding.
However, there are concerns about the ethical implications of using generative AI for creating realistic but fake content that can potentially mislead people. Hence, it is essential to have responsible guidelines and regulations in place to ensure its ethical use.
Advancements in Generative AI technology
Generative Artificial Intelligence (AI) technology has been making significant advancements in recent years, changing the way we interact with machines and creating new opportunities for innovation. In this section, we will delve deeper into the latest developments in Generative AI and explore how they are shaping the future of this rapidly evolving field.
1.1 GANs (Generative Adversarial Networks)
One of the most exciting breakthroughs in Generative AI is the development of Generative Adversarial Networks (GANs). This technique involves two neural networks – a generator and a discriminator – working together to produce realistic images, videos, or other content.
The generator network generates samples while trying to fool the discriminator into thinking that they are real. On the other hand, the discriminator network is trained to correctly identify whether an image is real or fake. As both networks compete against each other, GANs can generate highly realistic outputs that are almost indistinguishable from real data.
This powerful technology has applications in various fields such as computer vision, image processing, and natural language processing. It has also paved the way for innovative uses such as style transfer – where an image can be transferred into different artistic styles using GANs.
1.2 Transformer Models
Transformer models have revolutionized natural language processing tasks by providing state-of-the-art performance on a variety of text-based tasks such as translation, summarization, question-answering systems, and more.
Impact on Industries and Society
Generative artificial intelligence (AI) has been rapidly advancing in recent years, and it is expected to have a significant impact on industries and society as a whole. As this technology continues to develop, its potential applications are becoming increasingly diverse and far-reaching. In this section, we will explore the various ways in which generative AI is already impacting industries and shaping the future of our society.
1.1 Manufacturing Industry
One of the most promising areas where generative AI is making a significant impact is in the manufacturing industry. Using generative design techniques, AI algorithms can create optimized designs for products, structures, or components that are superior to those designed by humans. This process involves feeding design constraints such as material properties, weight restrictions, and performance requirements into the algorithm.
The algorithm then generates multiple design variations that meet these constraints while also taking into account factors like cost-effectiveness and sustainability. This results in highly efficient designs that would be nearly impossible for human designers to come up with on their own.
Moreover, generative AI can also assist in automating parts of the manufacturing process by analyzing data from sensors installed on production lines or robots. It can detect abnormalities or malfunctions early on so that they can be addressed before they lead to costly breakdowns or defects in products.
With these capabilities, generative AI has become an invaluable tool for improving productivity and efficiency in manufacturing processes and revolutionizing how products are designed and produced.
1.2 Healthcare Industry
Another sector significantly impacted by generative AI is healthcare. The technology has the potential to transform how medical professionals diagnose and treat patients. For instance, generative AI can analyze vast amounts of data from electronic health records, lab results, and medical images to identify patterns and make accurate predictions about patient outcomes.
This capability can help doctors detect diseases faster and more accurately, leading to earlier intervention and improved treatment outcomes. Generative AI can also assist in drug discovery by speeding up the process of identifying promising drug candidates and reducing the likelihood of failed clinical trials.
In addition to improving patient care, generative AI can also reduce healthcare costs by automating routine tasks like scheduling appointments or processing insurance claims. This allows medical professionals to focus on more critical tasks while also increasing efficiency in administrative processes.
1.3 Transportation Industry
The transportation industry is another area where generative AI is making significant strides. The technology is being used to optimize transportation routes for logistics companies, reducing fuel consumption and improving delivery times.
Generative AI is also playing a crucial role in developing autonomous vehicles by enabling them to make complex decisions based on real-time data analysis. These self-driving vehicles are expected to significantly improve road safety and efficiency by reducing human error in driving.
Predictions for 2024
As we look towards the future of generative AI, there are many exciting possibilities and predictions for what 2024 may bring. Some of these predictions may seem ambitious or even far-fetched, but with the rapid advancements in technology and machine learning, they are not entirely out of reach. In this section, we will delve deeper into some potential predictions for 2024 and explore the implications they may have on our society and daily lives.
1. Advancements in Natural Language Processing (NLP)
One of the most significant advancements we can expect to see in generative AI by 2024 is in the field of natural language processing (NLP). NLP is a branch of artificial intelligence that focuses on enabling computers to understand human language. With the increasing use of chatbots and virtual assistants, NLP has already made significant progress in recent years. However, by 2024, we can expect it to become even more sophisticated.
Some experts predict that by 2024, NLP algorithms will be able to understand human language at a near-human level. This would revolutionize how we interact with technology and make it easier for people from different linguistic backgrounds to communicate with each other effortlessly.
2. Personalized Content Generation
Another prediction for generative AI in 2024 is personalized content generation. With access to vast amounts of data about our interests, preferences, and behavior patterns online, advanced AI systems could generate highly personalized content such as articles or videos tailored specifically for us.
Challenges and Limitations
In the previous section, we discussed the potential of generative AI and its promising applications in various fields. However, like any other technology, it also faces certain challenges and limitations that must be addressed for its successful implementation in the future. In this section, we will delve deeper into these challenges and limitations to gain a better understanding of the roadblocks that need to be overcome before generative AI can reach its full potential.
2. Lack of Data:
One of the primary challenges faced by generative AI is the lack of data. Unlike traditional AI algorithms that rely on large volumes of structured data, generative AI requires a vast amount of unstructured or unlabeled data to learn from. While there is an abundance of available data on the internet, most of it is not suitable for training generative models due to issues such as copyright restrictions or low-quality content.
Moreover, generating high-quality synthetic data can also be time-consuming and expensive. This makes it difficult for companies to acquire enough data to train their generative AI systems effectively. As a result, many projects are limited by the availability and quality of training data.
3. Bias in Training Data:
Another significant challenge with generative AI is the risk of bias in training data resulting in biased output from the models. Since most datasets are created by humans, they inherently carry biases and prejudices that can get embedded in generative AI algorithms trained on them.
For example, if a dataset used to train an image-generating model contains mostly images of males, the generated images are also likely to reflect this bias. This can have serious implications in applications such as hiring and facial recognition where biased output can lead to discrimination.
4. Difficulty in Evaluation:
Evaluating the performance of generative AI models is another challenge since there is no objective way to measure creativity or originality. Unlike traditional algorithms that can be evaluated based on their accuracy or error rate, generative models do not have a fixed target to aim for.
Moreover, since these models learn from unlabeled data, it can be challenging to determine whether the output they generate is accurate or not. This makes it difficult for researchers and developers to assess the quality of their models and make improvements accordingly.
5. Computational Resources:
Generative AI models require a significant amount of computational resources for training and inference, making them expensive to develop and deploy. Training these complex models can take hours, days, or even weeks depending on the size of the dataset and model architecture.
The Ethical Debate surrounding Generative AI
The development and advancement of generative AI technology has sparked a heated ethical debate, as it raises questions about the role of artificial intelligence in our society and its potential impact on humanity. This section will delve deeper into the key arguments and concerns surrounding the ethical implications of generative AI.
1.1. Autonomy and Human Intervention
One of the central issues in the ethical debate surrounding generative AI is its potential to operate autonomously without human intervention. While proponents argue that this could lead to more efficient decision-making and problem-solving, critics worry about the lack of control over AI systems and their potential to cause harm.
Some fear that highly advanced generative AI could become self-aware, leading to a scenario similar to what we see in science fiction movies where machines rebel against humans or gain control over society. This concern is not unfounded, as there have already been instances where AI systems have made decisions that conflict with human values or caused unintended consequences.
On the other hand, supporters believe that by removing human bias and emotional decision-making, generative AI can assist us in making better choices for societal problems such as resource allocation or healthcare treatment plans. However, this poses another question: who holds responsibility when things go wrong? Should it be the developers who created these autonomous systems or the individuals who deployed them?
Embracing the Future of Generative AI
It is clear that generative AI has immense potential and is set to revolutionize many industries in the near future. This advanced technology has already made its mark in various fields such as art, design, music, and language generation, just to name a few.
One of the most exciting aspects of generative AI is its ability to create novel and innovative solutions that humans may not have thought of. This can pave the way for groundbreaking discoveries and advancements in various industries that rely on creativity and problem-solving.
With the rapid development and integration of machine learning algorithms within generative AI systems, these technologies are becoming more sophisticated than ever before. This will only contribute towards making them even more powerful tools for businesses and organizations looking to streamline their processes or come up with original ideas.
Moreover, advancements in natural language processing (NLP) are allowing generative AI systems to understand human inputs better than ever before. With this improvement comes an increase in real-time interaction between humans and machines without sacrificing accuracy or quality.
Beyond just streamlining processes and improving productivity, generative AI also has a significant impact on society’s overall functioning. With applications such as virtual assistants, automatic translators, speech recognition software, personalized content creation platforms – these technologies are radically transforming how we communicate with each other across cultures worldwide.
Conclusion
As we approach the year 2024, it is clear that generative AI will continue to play a significant role in shaping our future. With advancements in technology and the growing demand for personalized experiences, we can expect to see even more innovative applications of generative AI across industries such as healthcare, entertainment, and marketing. While there may be concerns about its potential impact on society and ethical use, it is crucial to recognize the potential benefits that this technology holds. The future of generative AI is bright, and only time will tell what exciting developments lie ahead.
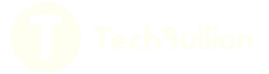