In today’s era of digital transformation, artificial intelligence (AI) is redefining the way databases are managed and optimized. Traditional database performance tuning, which has long relied on manual intervention by Database Administrators (DBAs), is now undergoing a significant shift towards predictive and autonomous systems. With AI-driven workload forecasting, automated indexing, and anomaly detection, organizations are witnessing unparalleled efficiency and accuracy in database operations. This article explores key innovations in AI-powered database performance tuning, a subject extensively researched by Ellavarasan Asokan, whose insights have shed light on the technological advancements in this domain.
The Evolution of Database Performance Tuning
Historically, database performance tuning has been reactive rather than proactive, with DBAs spending considerable time diagnosing slow queries and adjusting system configurations manually. However, AI transforms this process by integrating predictive analytics and automation, allowing databases to optimize themselves dynamically. By analyzing patterns and past performance data, AI-driven systems can anticipate issues before they arise, reducing downtime and enhancing overall system efficiency.
AI-Based Workload Prediction: A Game Changer
One of the most impactful applications of AI in database management is predictive workload forecasting. AI models, leveraging machine learning and deep learning algorithms, can analyze historical trends and predict future database loads with impressive accuracy. This capability enables organizations to allocate resources proactively rather than responding to unexpected demand surges. By preemptively adjusting computational resources, AI-based workload prediction reduces costs while ensuring seamless performance, particularly in high-traffic scenarios.
Intelligent Indexing for Optimized Queries
AI-driven automated indexing revolutionizes database performance by eliminating the inefficiencies of manual indexing. Using reinforcement learning and neural networks, AI analyzes query patterns, workload trends, and access frequencies to dynamically recommend and adjust indexing structures. Unlike static indexing, which may become suboptimal as data evolves, AI continuously refines its strategy based on real-time database usage. This ensures that frequently accessed data is efficiently indexed while minimizing storage overhead. The result is faster query execution, reduced computational strain, and enhanced overall database responsiveness, optimizing performance in complex and ever-changing data environments.
Anomaly Detection and Proactive Performance Monitoring
AI-driven anomaly detection in database performance is a game-changer, surpassing traditional threshold-based monitoring systems. Instead of relying on static rules, AI employs machine learning to learn normal operating behaviors and detect deviations in real time. By analyzing query execution times, CPU and memory usage, disk I/O, and transaction patterns, AI can pinpoint potential issues such as inefficient queries, resource contention, or security threats before they escalate. This proactive approach significantly reduces mean-time-to-diagnosis (MTTD), allowing database administrators to resolve problems swiftly. Additionally, AI-driven insights help optimize database configurations, prevent downtime, and enhance overall system stability. By continuously refining its understanding of database performance trends, AI ensures a more resilient and efficient data management environment, improving both user experience and business continuity.
Overcoming Challenges in AI-Driven Database Optimization
Despite its advantages, integrating AI into database performance tuning is not without challenges. One major hurdle is the requirement for continuous learning, as database workloads evolve over time. AI models must be regularly updated to maintain their predictive accuracy. Additionally, organizations relying on legacy database systems may encounter difficulties in integrating AI-powered solutions due to outdated architectures and limited support for advanced automation features. However, as AI technology advances and database platforms become more adaptive, these challenges are gradually being addressed.
The Future of Autonomous Database Management
The ultimate vision for AI in database management is full autonomy—where databases self-tune, self-repair, and self-optimize with minimal human intervention. AI-driven autonomous databases are poised to revolutionize data management by dynamically adjusting performance parameters, optimizing storage, and even predicting system failures before they occur. This transition will redefine the role of DBAs, shifting their focus from routine maintenance to strategic data architecture and innovation.
In conclusion, Ellavarasan Asokan’s research highlights AI’s transformative potential in predictive database performance tuning. By leveraging AI-driven workload forecasting, intelligent indexing, and advanced anomaly detection, organizations can achieve unprecedented levels of efficiency and reliability in their database operations. As AI technologies evolve, businesses that embrace autonomous database management will position themselves at the forefront of digital innovation, ensuring seamless, optimized, and future-ready data infrastructures.
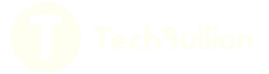